An efficient machine learning approach for activity recognition
Journal of Physics: Conference Series(2021)
摘要
Abstract The population of older people in western nations are growing drastically. Independent lifestyle is their preference and this leads to fall instances often. Falls of this kind results in severe health issues or sometimes causes deadly damages to the elderly people. Considering this problem, it is highly important to come up with fall discovery systems. A machine learning framework has been proposed in this regard which covers both fall discovery and day to day movement recognition. Utilizing acceleration and speed inputs from dual unrestricted repositories are highly helpful in diagnosing to the maximum of seven movements. Acceleration and angular velocity parameters can be used to extract the attributes that are relevant to time and frequency domain. These attributes are then offered to a classification algorithm. An attempt has been made to check the outcomes of four different procedures for categorizing manual movements. The above-mentioned procedures are the Artificial Neural Network (ANN), K-Nearest Neighbours (KNN), Quadratic Support Vector Machine (QSVM), and FP growth. Power spectral density of the acceleration is used to maximize the outcomes of the classifier. Acceleration data alone is considered for activity discovery in the initial step. Experimental results show that the KNN, ANN, QSVM, and FP growth procedures can attain correctness of 81.2%, 87.8%, 93.2%, and 94.1%. The accuracy of fall detection touches 97.2% and 99.1% with no false positive values for the QSVM and FP growth procedures. As a next phase, the attributes can be taken autocorrelation function and the power spectral density of the acceleration and the angular velocity data that obviously maximizes the classification correctness. Projected attributes are adopted to achieve notable improvement in accuracy of 85.8%, 91.8%, 96.1%, and 97.7% for the KNN, ANN, QSVM, and FP growth algorithm. The accurateness of fall discovery touches 100% for both the QSVM and FP growth procedures in the absence of any incorrect alarm, which is the finest attainable performance.
更多查看译文
关键词
efficient machine learning approach,activity,machine learning,recognition
AI 理解论文
溯源树
样例
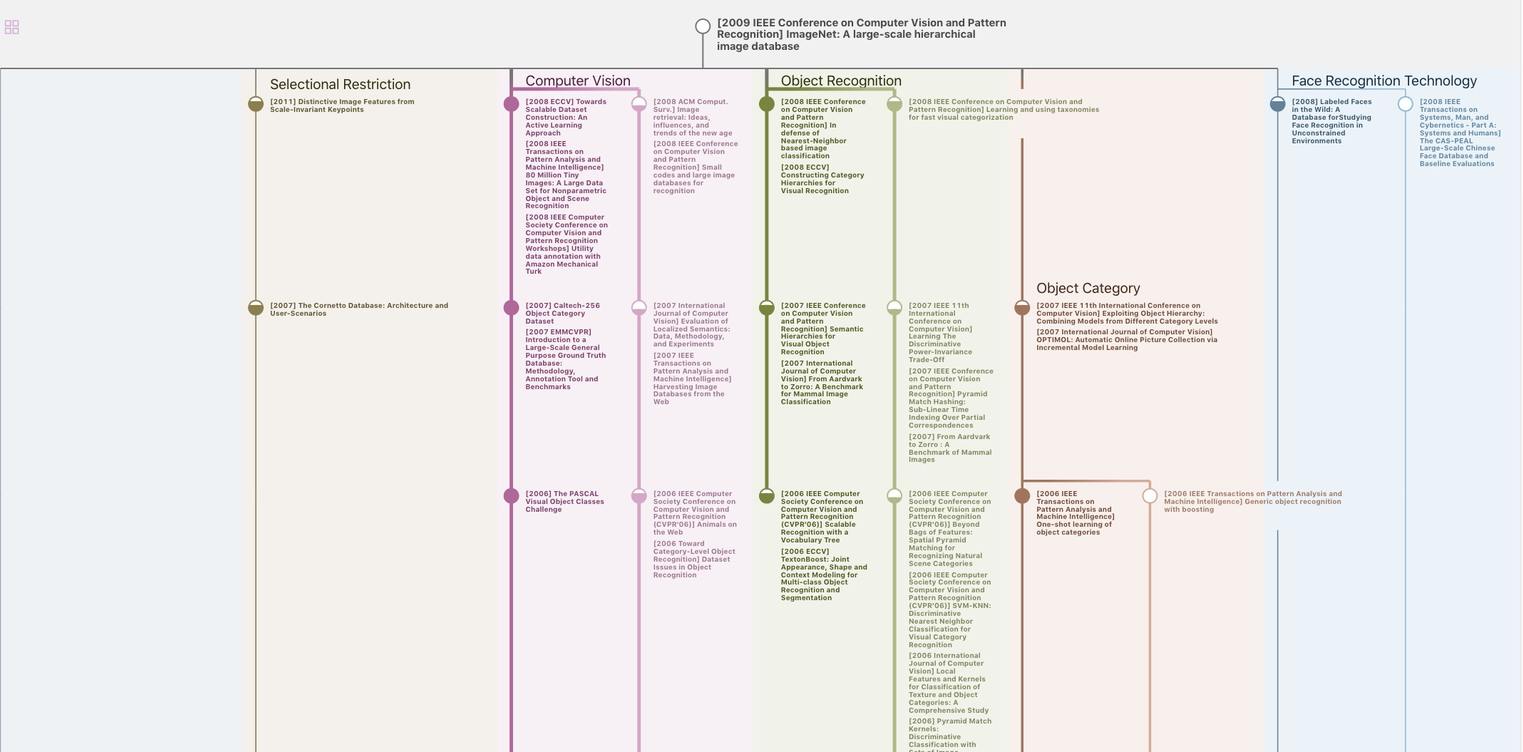
生成溯源树,研究论文发展脉络
Chat Paper
正在生成论文摘要