Binary Cuckoo Optimisation Algorithm and Information Theory for Filter-Based Feature Selection
Lecture Notes on Data Engineering and Communications TechnologiesInnovative Systems for Intelligent Health Informatics(2021)
摘要
Dimensionality reduction is among the data mining process that is used to reduce the noise and complexity of the features. Feature selection (FS) is a typical dimensionality reduction that is used to reduce the unwanted features from the datasets. FS can be either filter or wrapper. Filters lack interaction among selected subsets of features which in turns affect the classification performance of the chosen subsets of features. This study proposes two ideas of information theory entropy (E) as well as mutual information (MI). Both of them were used together with binary cuckoo optimisation algorithm BCOA (BCOA-E and BCOA-MI) to reduce both the error rate and computational complexity on four different datasets. A support vector machine classifier was used to measure the error rates. The results are in favour of BCOA-E in terms of accuracy. In contrast, BCOA-MI is computationally faster than BCOA-E. Comparison with other approaches found in the literature shows that the proposed methods performed better in terms of accuracy, number of selected features and execution time.
更多查看译文
关键词
Feature selection,Filter-based,Binary Cuckoo optimisation,Information theory
AI 理解论文
溯源树
样例
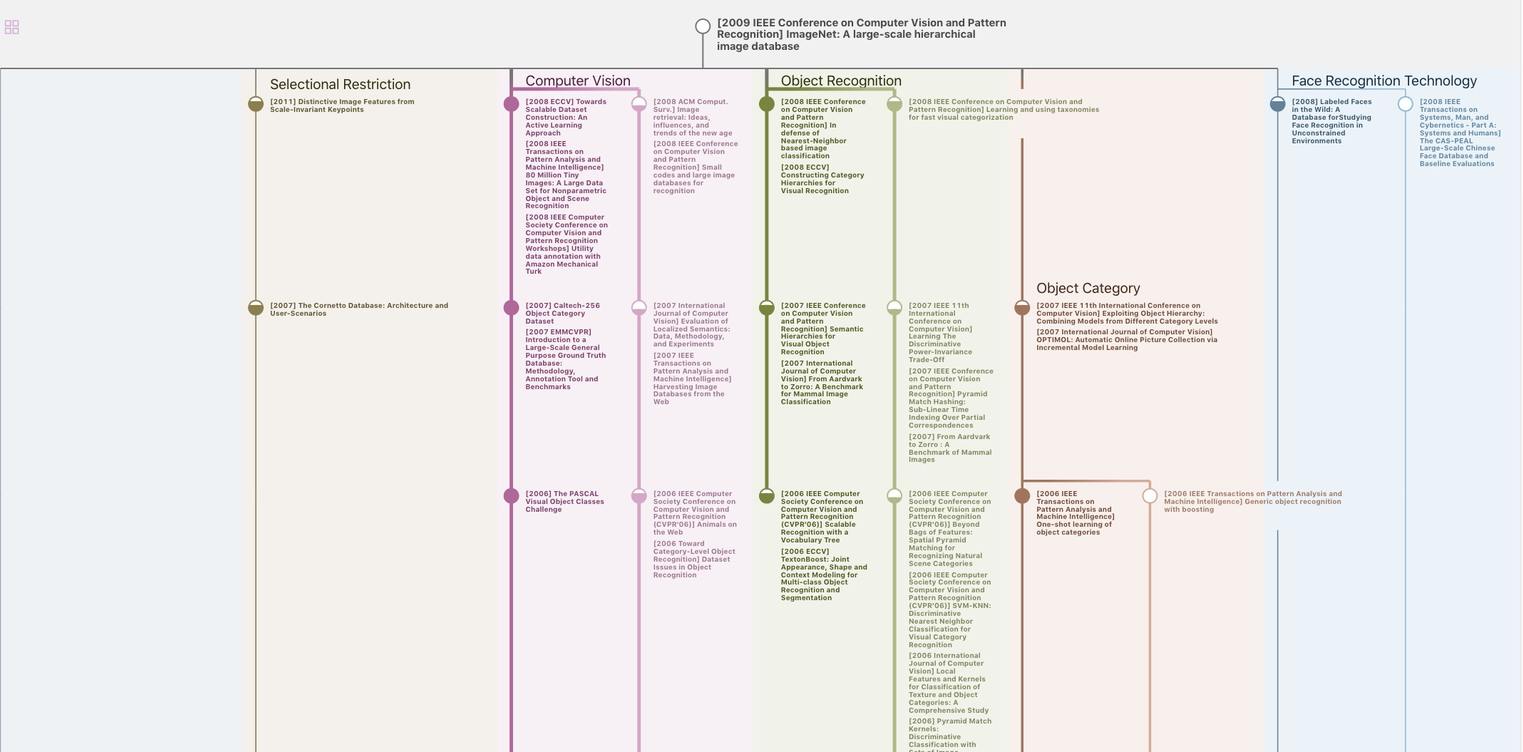
生成溯源树,研究论文发展脉络
Chat Paper
正在生成论文摘要