Mobile Phone Sensor-Based Detection of Subjective Cannabis “High” in Young Adults: A Feasibility Study in Real-World Settings
Abstracts from the 2020 Virtual Scientific Meeting of the Research Society on Marijuana July 24th, 2020(2021)
摘要
Aim: Acute cannabis intoxication can impair motor skills and cognitive functions. Given possible impairment related to acute cannabis intoxication, we explored whether mobile phone-based sensors (e.g., GPS, text/phone logs) can detect episodes of acute cannabis intoxication (subjective “high” state) as self-reported in natural environments by young adults. Methods: Young adults (ages 18-25), who reported cannabis use at least twice per week, were recruited by research registry and Craigslist to participate in a mobile phone data collection study (up to 30 days) in Pittsburgh, PA (2017-2019). Participants responded to fixed time phone surveys (3 times/day) and self-initiated reports of cannabis use (start/stop time, rating of subjective high: 0-10, 10=very high). Our mobile AWARE app continuously collected phone sensor data, which was segmented into 5-minute windows for analysis. We built and tested multiple machine learning classifiers (e.g., Support Vector Machine, Light Gradient Boosting Machine (LGBM)) on training (60%), validation (20%), and test (20%) datasets to determine which classifier performed best in distinguishing subjective cannabis “high” (rating=1-10) vs “not high” (rating=0). To minimize the influence of imbalanced data on model performance in the training dataset, we used both over-sampling with Synthetic Minority Over-sampling Technique (SMOTE) and random under-sampling of the majority class, so that both classes (“high”, “not-high”) had the same number of training samples. We also tested the importance of time features (i.e., day of week, time of day: morning, afternoon, evening) relative to smartphone sensor data only on model performance, since time features alone might predict “routines” in cannabis use. Results: Young adults (N=57; 58% female; mean age=19.82 [SD=1.76]; 71.92% White, 15.78% Black, 12.28% Asian and other ethnicity) reported 451 episodes of cannabis use, mean subjective high rating=3.77 (SD=2.64). The sensor dataset included 1,648 datapoints representing reports of subjective ""high"" and 60,580 data points representing ""not high"" reports. For the two time-based features only model, the LGBM classifier had 91% accuracy in detecting subjective cannabis intoxication (vs “not-high”) in the test dataset (Area Under the Curve [AUC]=0.75). Combining smartphone sensor data with the two time-based features (day of week, time of day) improved model performance, with 95% accuracy (AUC=0.93), indicating that smartphone features contribute unique information, and that time features further improve model performance in detecting rating of subjective cannabis ""high"". Among the 102 phone sensor features entered into the analyses (smartphone sensors + time model), some of the most important features (the top 2 were the time features) included travel (GPS: smaller travel radius within a day when feeling ""high”), movement (e.g., smaller number of activity changes when feeling ""high”), and communication/sociability (e.g., increased phone usage interactions, greater voice and noise level around individuals). Conclusion: Results from this proof-of-concept study indicate the feasibility of using phone sensors to detect effects of cannabis intoxication in the natural environment in a population-based model among young adults. Mobile phone sensors show promise for automated and continuous detection of cannabis use in daily life in a sample of young adults, with potential implications for triggering the delivery of just-in-time interventions to minimize marijuana-related harm.
更多查看译文
AI 理解论文
溯源树
样例
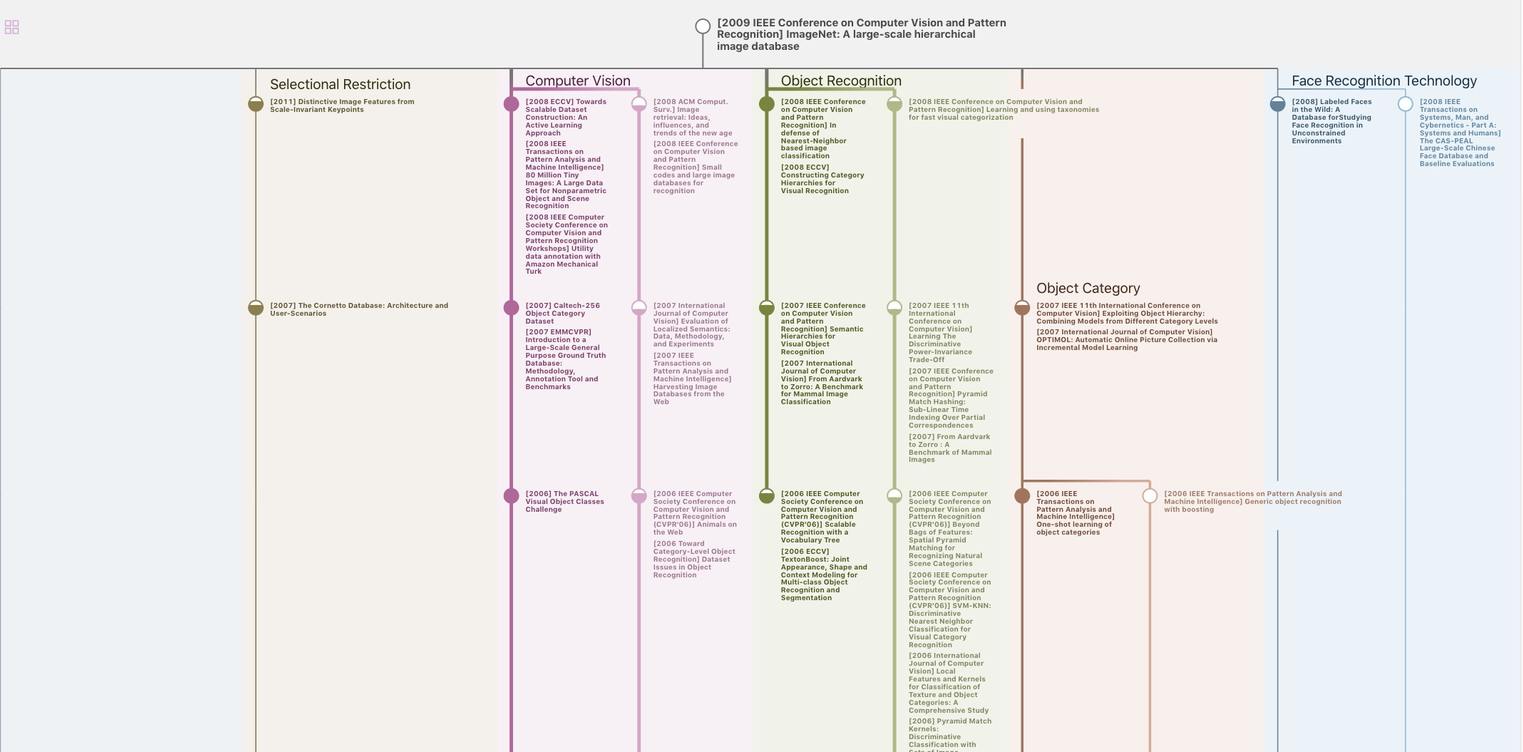
生成溯源树,研究论文发展脉络
Chat Paper
正在生成论文摘要