Hybrid Twins. Part II. Real-time, data-driven modeling
ESAFORM 2021(2021)
摘要
We have seen in Part I of this paper that model order reduction allows the involvement of physics-based models in design, as in the past, but now also in online decision-making, without requiring unreasonable computing resources. On the other hand, machine learning techniques were not ready to cope with the processing speed and the lack of data. It was therefore necessary to adapt a number of techniques, and to create others, capable of operating online and even in the presence of a very small amount of data: the so-called "physics-informed artificial intelligence" techniques. For that purpose, we have adapted and proposed a number of techniques, which have proven and are proving every day in many industrial applications their capabilities and performances. Six major uses of AI in engineering concern: (i) visualization of multidimensional data; (ii) classification and clustering, supervised and unsupervised, where it is assumed that members of the same cluster have similar behaviors; (iii) model extraction, that is, discovering the quantitative relationship between inputs (actions) and outputs (reactions) in a consistent manner with respect to the physical laws. When addressing knowledge extraction, item (iv) above, as well as the need of explaining for certifying, item (v), advances are much limited and both items need for major progresses, as the one enabling discarding useless parameters, or discovering latent variables whose consideration becomes compulsory for explaining experimental findings, or combining parameters that act in a combined manner. Discovering equations is a very timely topic because it finally enables transforming data into knowledge.
更多查看译文
AI 理解论文
溯源树
样例
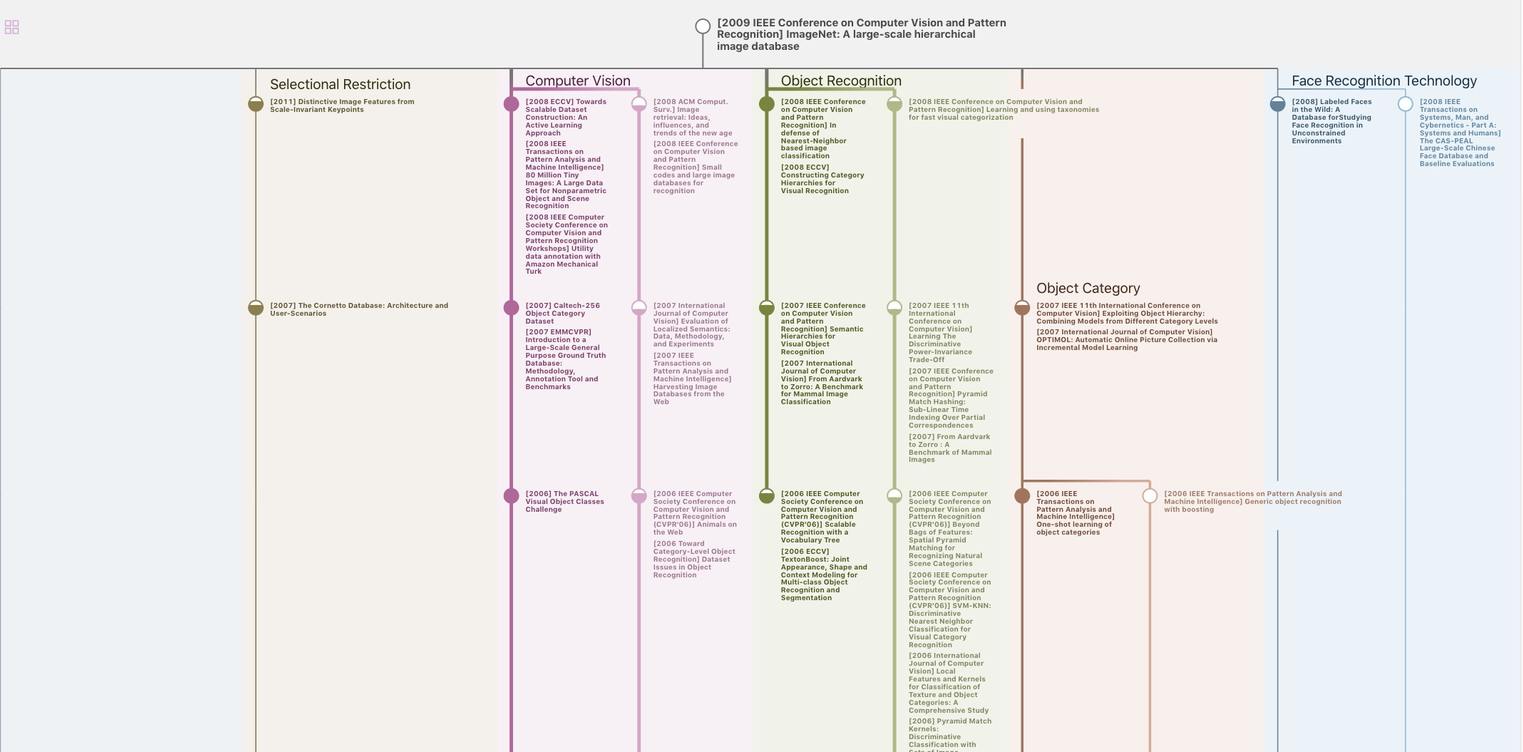
生成溯源树,研究论文发展脉络
Chat Paper
正在生成论文摘要