Design Exploration of Machine Learning Data-Flows onto Heterogeneous Reconfigurable Hardware
Anais do XXI Simpósio em Sistemas Computacionais de Alto Desempenho (WSCAD 2020)(2020)
摘要
Machine/Deep learning applications are currently the center of the attention of both industry and academia, turning these applications acceleration a very relevant research topic. Acceleration comes in different flavors, including parallelizing routines on a GPU, FPGA, or CGRA. In this work, we explore the placement and routing of Machine Learning applications dataflow graphs onto three heterogeneous CGRA architectures. We compare our results with the homogeneous case and with one of the state-of-the-art tools for placement and routing (P&R). Our algorithm executed, on average, 52% faster than Versatile Place&Routing (VPR) 8.1. Furthermore, a heterogeneous architecture reduces the cost without losing performance in 76% of the cases.
更多查看译文
AI 理解论文
溯源树
样例
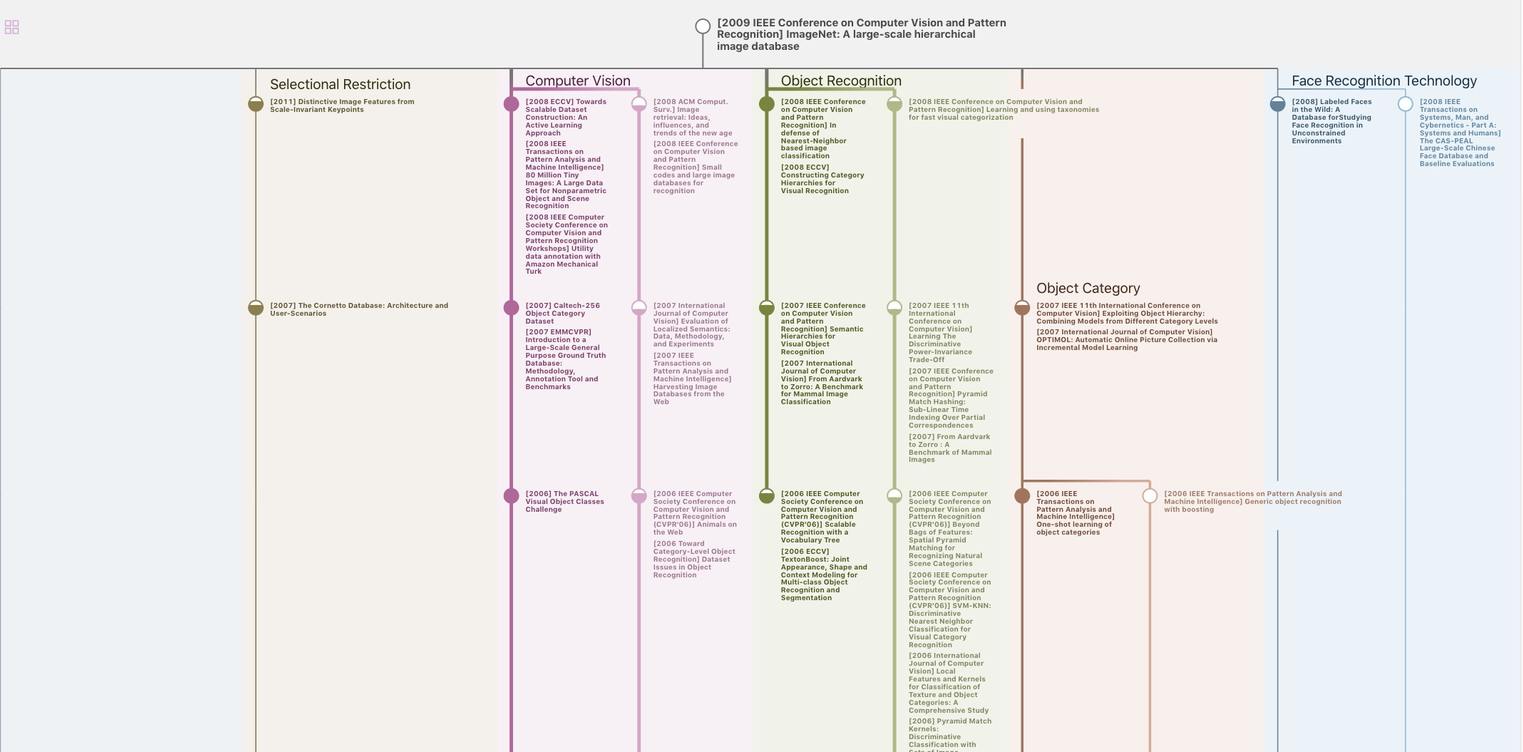
生成溯源树,研究论文发展脉络
Chat Paper
正在生成论文摘要