Reaction: A commentary on Lustick and Tetlock (2021)
FUTURES & FORESIGHT SCIENCE(2021)
摘要
Lustick and Tetlock (2021) argue for the use of theory-guided simulation for aiding geopolitical planning and decision-making. This is a very welcome contribution, as in our experience, geopolitical analyses are often qualitative in nature and prone to group think. The complexities of geopolitical issues, however, really call for the use of theory-guided simulations. These issues are too complex for mental simulation (Atkins et al., 2002; Sterman, 1989, 1994). Dynamic simulations, if properly grounded in appropriate theories and well-motivated assumptions, can derive the possible dynamics from interacting nonlinear processes, and thus aid human reasoning about system behavior (Sterman, 2002). Moreover, since geopolitical issues are subject to uncertainty, scarce data, and conflicting information, using an ensemble modeling approach is appropriate. An ensemble of simulations enables reasoning across alternative assumptions consistent with the available data and information. Such an ensemble can capture much more of the available theories, information, and educated guesses than any single model in isolation (Bankes, 2002). With the rising computational power, ensemble modeling is increasingly a feasible research strategy. Despite our broad agreement with Lustick and Tetlock (2021), we have three major comments on their work. First, from the broader perspective of modeling and simulation, they offer little that is truly novel or surprising. The envisioned approach of ensemble simulations is already well established under the label of exploratory modeling. By not engaging with this literature, the authors have deprived themselves from a rich set of analytical techniques that could have substantially strengthened their case study, as well as relevant theories and concepts which would have strengthened the appeal of the manifesto. Second, we content that validating simulation models of complex systems with partially open system boundaries should focus of perceived usefulness, not supposedly predictive accuracy captured through brier scores. Third, in interpreting results from simulation models it is possible and useful to try and increase understanding between the system's structural characteristics and theories, instead of using simulations as point predictions. There is ample literature that has emerged over the last 30 years on the use of computational experimentation with simulation models to aid planning and decision-making. Hodges (1991) identified six things that could be done with simulation models in the absence of good data. Hodges and Dewar (1992) identified a seventh use case. Bankes (1993) moved away from enumerating the number of use cases, and simply spoke of exploratory modeling. Since these formative ideas from the early 1990s, a large body of literature on exploratory modeling has emerged (see, e.g., Kwakkel, 2017; Moallemi et al., 2020 for detailed overviews). Perhaps most importantly, exploratory modeling has become one of the cornerstones of the field of decision-making under deep uncertainty (Marchau et al., 2019). It is quite unfortunately that the authors seem to be utterly unaware, with the exception of Davis et al. (2007), of this rich existing body of literature on exploratory modeling. The reported case study could have benefitted from various techniques for model analysis like scenario discovery (Bryant & Lempert, 2010; Kwakkel & Jaxa-Rozen, 2016) and global sensitivity analysis (Razavi et al., 2021). In particular, dynamic scenario discovery (Kwakkel et al., 2013; Steinmann et al., 2020) could have been useful in identifying characteristics dynamics over time, as well as their conditions for occurring. Dynamics scenario discovery and global sensitivity analysis could substantially enhance the mapping of the state space of possibilities by identifying the conditions under which the different possibilities would occur. So, in their Bangladeshi case study, these techniques could have helped in answering questions about the representativeness of the three scenarios for the entire ensemble, and the regions of the model input space from which these three scenarios originate. This, in turn, would enable grounding the resulting narratives much more strongly in the underlying model (Greeven et al., 2016). Lustick and Tetlock (2021) strongly emphasize the importance of theoretically grounding the simulation models. Unfortunately, for many social phenomena, we have a variety of possible theoretical explanations. Rather than narrowing down on a preferred theory, exploratory modeling is increasingly being used for opening up the conversation by exploring over a range of alternative theories. For example, Pruyt and Kwakkel (2014) explore the rise of home-grown terrorism following two rival theories and use this to identify policy interventions that work in either case. Both Mitchell (2003) and Page (2018) argue more generally for using a plurality of structurally different simulation models because such an ensemble sheds a rich light on the phenomenon under study, which is particularly appropriate in case of complex systems. Next to the potential of various techniques that have emerged over the last two decades for exploratory modeling, there have also been ample relevant theoretical developments that are directly relevant to the manifesto. Most importantly, what exactly is the status of individual computational experiments, and what is the status of the ensemble? A key question in this context is whether one can take the frequencies of occurrence of different classes of model results within an ensemble, and directly translate these frequencies into probabilities that hold in the real world. For obvious reasons, you cannot. The frequencies found in model land are critically dependent on the way in which one samples the model input space. Are the various dimensions that characterize the model input space taken as independent, or are correlations assumed? What prior is assumed for each of the dimensions? Modeling choices related to these questions strongly alter the observed frequencies (Quinn et al., 2020). So, instead of treating frequencies of possibilities in model land as probabilities that also hold in the real world, Shortridge and Zaitchik (2018) and Taner et al. (2019) explore ways of using scenario discovery results as input to an a posteriori Bayesian probabilistic assessment. This might offer an alternative way of synthesizing the inside and outside view as discussed by Lustick and Tetlock (2021) The authors use phrases like validation and prediction. These phrases are deeply problematic and should be used with care. Hodges and Dewar (1992), adopting a very narrow understanding of what proper validation is, argue that most simulation models used for policymaking cannot be validated. A similar, more modest, position is adopted by Oreskes et al. (1994), according to whom validation is only possible for closed systems. However, state stability and basically all other geopolitical issues, do not take place within closed systems. Historical replication, and thus also trying to establish predictive accuracy, is problematic as a source of evidence for validation because of equifinality. Building on this, Oreskes (1998) argues that one cannot proof the predictive reliability of models of complex systems prior to their actual use. Like with natural systems, in case of geopolitical systems one is confronted with limits to measurability, accessibility and a lack of spatiotemporal stationarity. The yardstick for model quality is thus not to be found in its purported predictive quality (e.g., brier scores), but rather in its perceived usefulness for guiding planning and decision-making. Ensembles of simulation experiments can meaningfully inform decision-making beyond merely the summary frequencies of types of outcomes. For example, the absence of a particular type of outcome in an ensemble can be highly decision relevant. An example of this is offered by Auping et al. (2014) who analyzed the geopolitical implications of the US’ shale revolution. In short, they first generated an ensemble of energy price dynamics at a global scale. A set of exemplars from this ensemble was subsequently used as input to a state stability model for stress testing a wide variety of rentier states to identify which of those were most vulnerable to price swings. The global energy model, across the ensemble showed low oil prices for the decade of 2010–2020. Under no condition explored by the model would there be high prices. This ran counter to conventional wisdom at, for example, NATO, where the results were presented. However, because of the underlying theory-informed causal model, there was a very good explanation for the absence of such high price simulation runs. This example highlights the value of analyzing the ensemble in more detail, and carefully explaining how the different types of dynamics arise out of the structural assumptions within the simulation models. We are in broad agreement with the plea of Lustick and Tetlock (2021) for the use of theory-guided simulations in aiding decision-making on complex issues, and content that this appeal has a reach well beyond geopolitical questions. Saltelli et al. (2019) lament the fact that simulation and modeling is not its own field (c.f., Padilla et al., 2017), yet is being practiced across many fields, because it hampers the development of shared best practices. The simulation manifesto of Lustick and Tetlock (2021) unfortunately exemplifies this regrettable state of affairs because it seems that they are trying to reinvent the wheel. We have highlighted here primarily the rich body of literature on exploratory modeling which could have meaningfully informed and strengthened the manifesto. Other examples where Lustick and Tetlock (2021) bypass much relevant literature could easily have been given as well. For example, both the shale gas study and the homegrown terrorism study relied on system dynamics models, rather than agent-based models, as advocated by Lustick and Tetlock (2021). Rather than fruitlessly debating which is the better approach, we content that both can be useful for representing key causal mechanisms, and both produce emergent aggregate dynamics. The primary difference is the level at which causal mechanisms are described. System dynamics models use a lumped, mesoscopic representation where the aggregate dynamics are an emergent property of interacting feedback loops involving accumulation and delay. If there is no obvious way of lumping, or compartmentalizing, your agents, a microscopic representation where the aggregate dynamics arise out of local interactions among heterogeneous agents as used in agent-based models is more suitable (c.f., Rahmandad & Sterman, 2008). Similar remarks could be made with respect to federating models, which under labels such as multimodeling, multimodel ecologies, and co-simulation is being practices in various scientific fields (Nikolic et al., 2019).
更多查看译文
关键词
tetlock,lustick,reaction,commentary
AI 理解论文
溯源树
样例
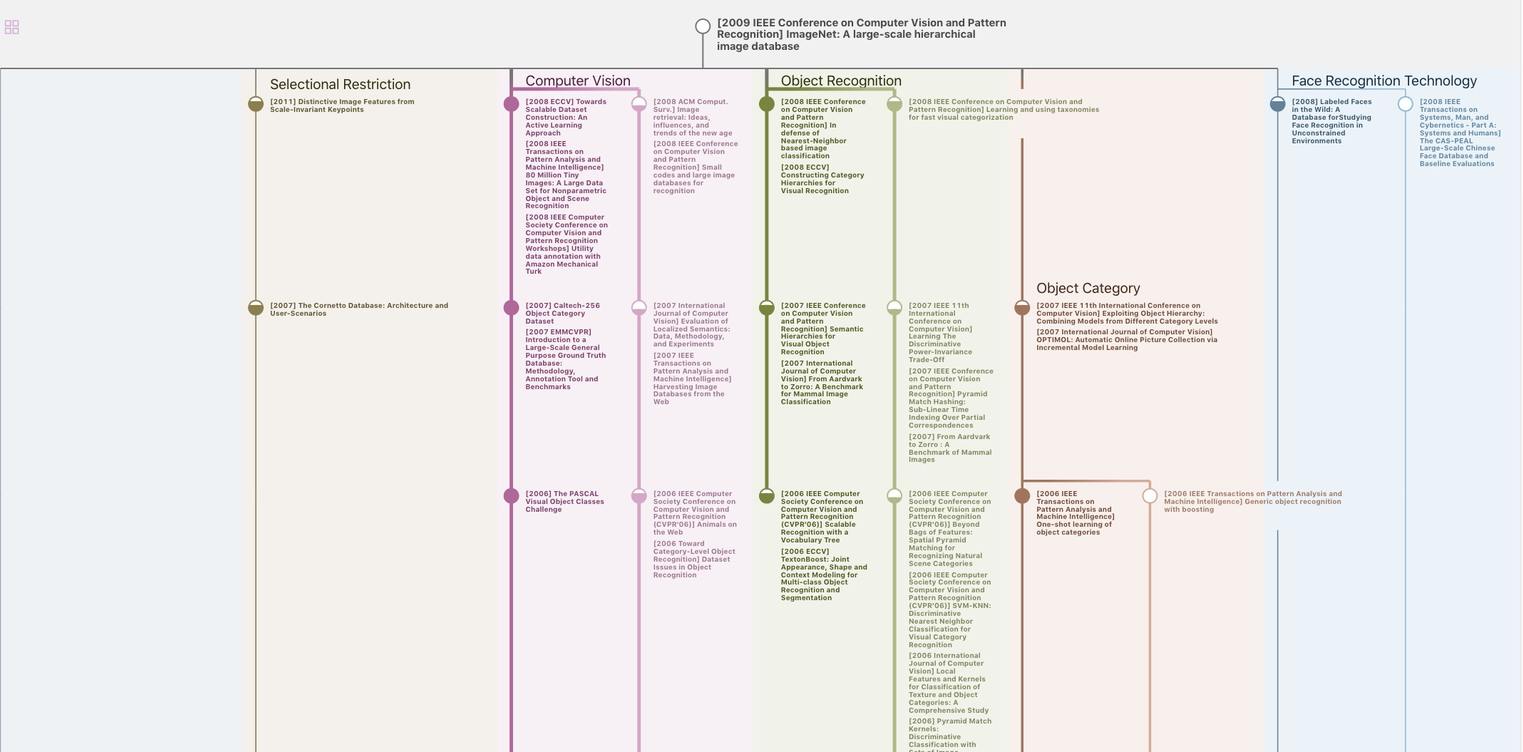
生成溯源树,研究论文发展脉络
Chat Paper
正在生成论文摘要