Effective Representation Learning via The Integrated Self-Supervised Pre-training models of StyleGAN2-ADA and DINO for Colonoscopy Images
biorxiv(2022)
摘要
In order to reach better performance in visual representation learning from image or video dataset, huge amount of annotated data are on demand. However, collecting and annotating large-scale datasets are costly and time-consuming tasks. Especially, in a domain like medical, it is hard to access patient images because of the privacy concerns and also not knowing what exactly to annotate without expert effort. One of the solutions to obviate the hassle is to use Self-Supervised learning methods (SSL) and Generative Adversarial Networks (GANs) together. SSL and GANs are quickly advancing fields. GANs have unique capability to create unlabeled data sources containing photo-realistic images while SSL methods are able to learn general image and video features from large-scale data without using any human-annotated labels. In this work, we explore leveraging the power of recently introduced StyleGAN2-ada and Self-Supervised Pre-Training of Dino together for the pretext task. Our underlying insight is also that leveraging the current approaches with Transfer Learning (TF) together brings benefit on doing pretext task in medical domain. By the strategy of unifying these two approaches, we propose the integrated version and use it derive representation learning on polyp dataset.
### Competing Interest Statement
The authors have declared no competing interest.
更多查看译文
关键词
colonoscopy images,effective representation learning,self-supervised,pre-training
AI 理解论文
溯源树
样例
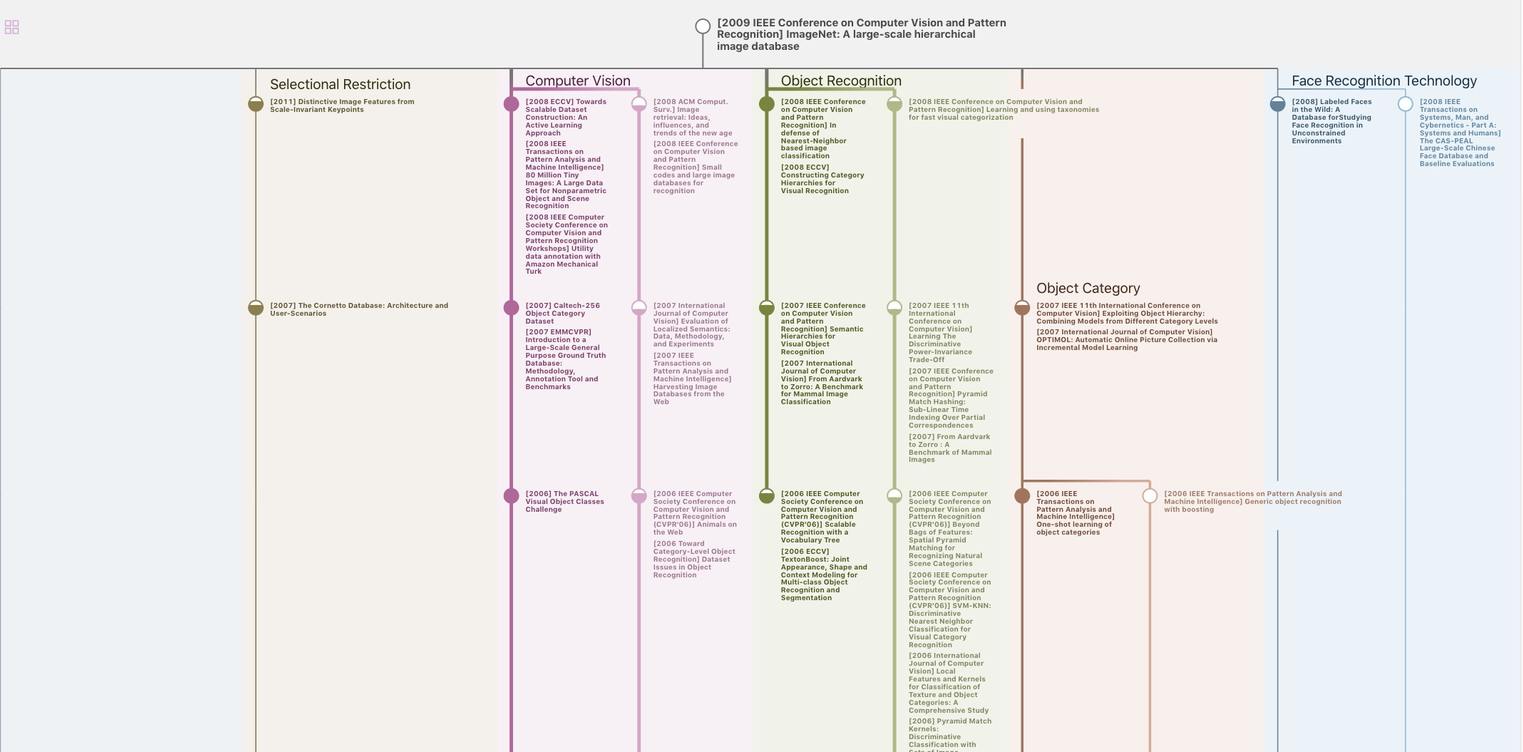
生成溯源树,研究论文发展脉络
Chat Paper
正在生成论文摘要