Online score statistics for detecting clustered change in network point processes
arxiv(2023)
摘要
We consider online monitoring of the network event data to detect local changes in a cluster when the affected data stream distribution shifts from one point process to another with different parameters. Specifically, we are interested in detecting a change point that causes a shift of the underlying data distribution that follows a multivariate Hawkes process with exponential decay temporal kernel, whereby the Hawkes process is considered to account for spatiotemporal correlation between observations. The proposed detection procedure is based on scan score statistics. We derive the asymptotic distribution of the statistic, which enables the self-normalizing property and facilitates the approximation of the instantaneous false alarm probability and the average run length. When detecting a change in the Hawkes process with nonvanishing self-excitation, the procedure does not require estimating the postchange network parameter while assuming the temporal decay parameter, which enjoys computational efficiency. We further present an efficient procedure to accurately determine the false discovery rate via importance sampling, as validated by numerical examples. Using simulated and real stock exchange data, we show the effectiveness of the proposed method in detecting change while enjoying computational efficiency.
更多查看译文
关键词
Change point detection,graph scanning statistics,score statistics
AI 理解论文
溯源树
样例
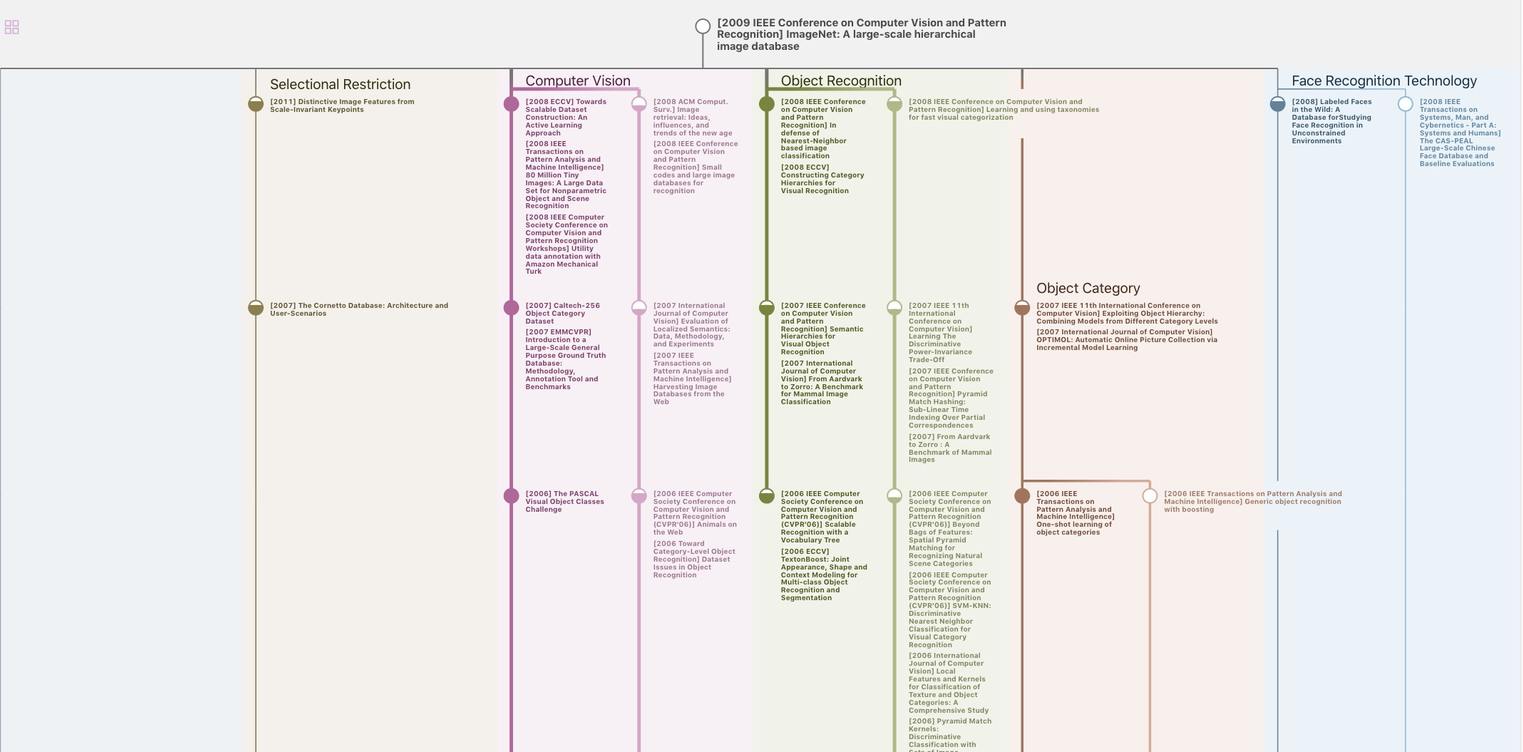
生成溯源树,研究论文发展脉络
Chat Paper
正在生成论文摘要