Classification of datasets with imputed missing values: does imputation quality matter?
arxiv(2022)
摘要
Classifying samples in incomplete datasets is a common aim for machine learning practitioners, but is non-trivial. Missing data is found in most real-world datasets and these missing values are typically imputed using established methods, followed by classification of the now complete, imputed, samples. The focus of the machine learning researcher is then to optimise the downstream classification performance. In this study, we highlight that it is imperative to consider the quality of the imputation. We demonstrate how the commonly used measures for assessing quality are flawed and propose a new class of discrepancy scores which focus on how well the method recreates the overall distribution of the data. To conclude, we highlight the compromised interpretability of classifier models trained using poorly imputed data.
更多查看译文
关键词
imputation
AI 理解论文
溯源树
样例
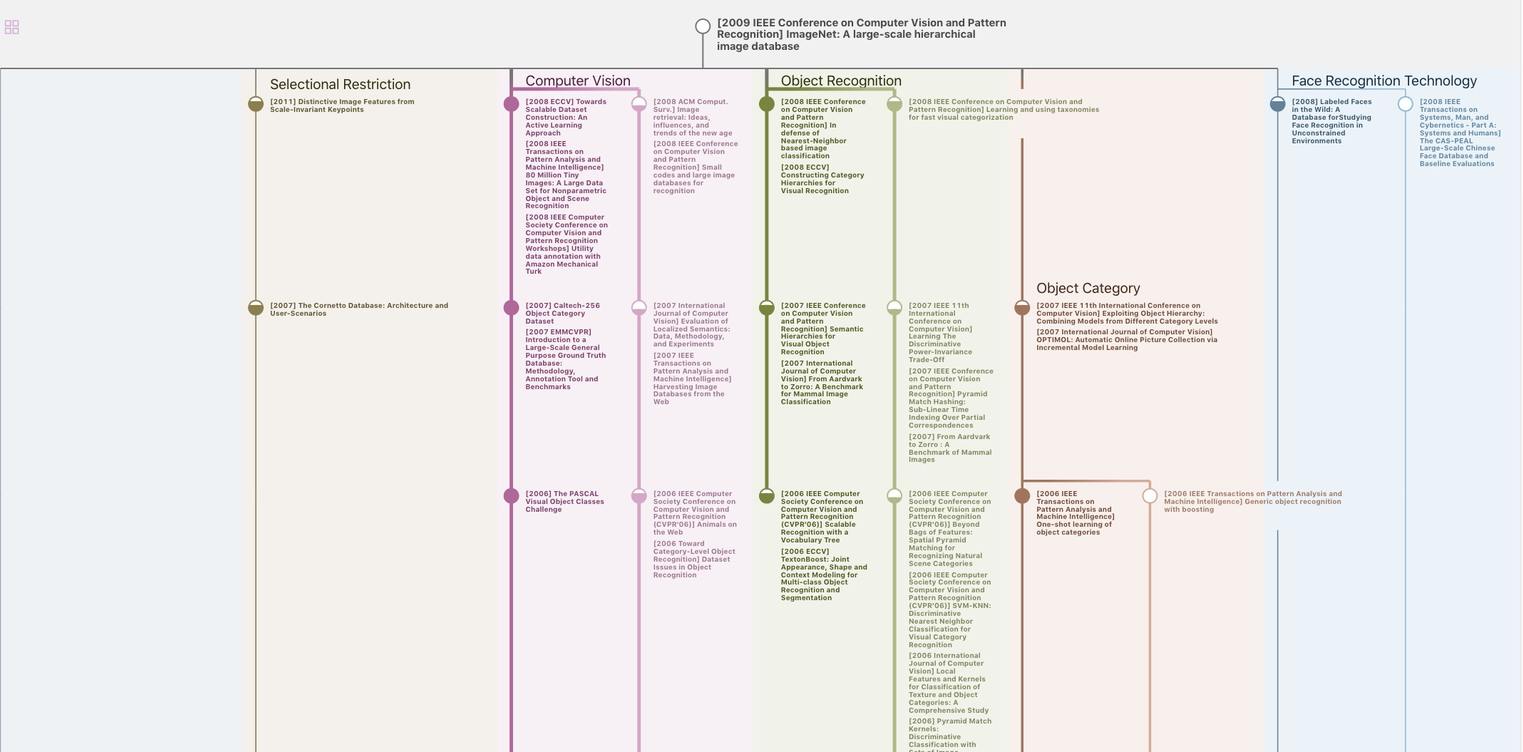
生成溯源树,研究论文发展脉络
Chat Paper
正在生成论文摘要