TLETA: Deep Transfer Learning and Integrated Cellular Knowledge for Estimated Time of Arrival Prediction.
International Conference on Intelligent Transportation Systems (ITSC)(2022)
摘要
Vehicle arrival time prediction has been studied widely. With the emergence of IoT devices and deep learning techniques, estimated time of arrival (ETA) has become a critical component in intelligent transportation systems. Though many tools exist for ETA, ETA for special vehicles, such as ambulances, fire engines, etc., is still challenging due to the limited amount of traffic data for special vehicles. Existing works use one model for all types of vehicles, which can lead to low accuracy. To tackle this, as the first in the field, we propose a deep transfer learning framework TLETA for the driving time prediction. TLETA constructs cellular spatial-temporal knowledge grids for extracting driving patterns, combined with the road network structure embedding to build a deep neural network for ETA. TLETA contains transferable layers to support knowledge transfer between different categories of vehicles. Importantly, our transfer models only train the last layers to map the transferred knowledge, that reduces the training time significantly. The experimental studies show that our model predicts travel time with high accuracy and outperforms many state-of-the-art approaches.
更多查看译文
关键词
Deep Transfer Learning,Estimated Time of Arrival (ETA),Integrated Cellular Knowledge
AI 理解论文
溯源树
样例
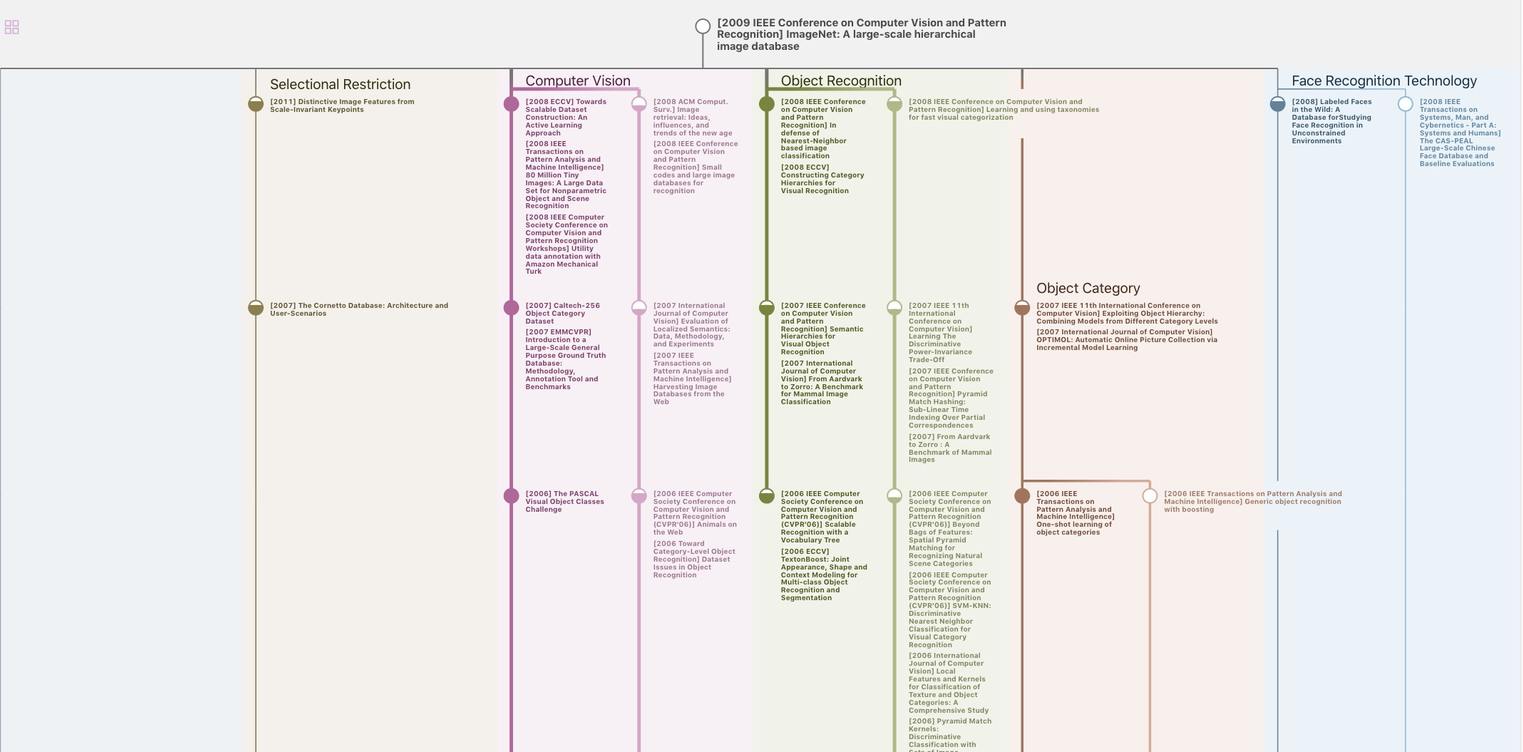
生成溯源树,研究论文发展脉络
Chat Paper
正在生成论文摘要