How You Start Matters for Generalization
arxiv(2022)
摘要
Characterizing the remarkable generalization properties of over-parameterized neural networks remains an open problem. In this paper, we promote a shift of focus towards initialization rather than neural architecture or (stochastic) gradient descent to explain this implicit regularization. Through a Fourier lens, we derive a general result for the spectral bias of neural networks and show that the generalization of neural networks is heavily tied to their initialization. Further, we empirically solidify the developed theoretical insights using practical, deep networks. Finally, we make a case against the controversial flat-minima conjecture and show that Fourier analysis grants a more reliable framework for understanding the generalization of neural networks.
更多查看译文
关键词
generalization,start,matters
AI 理解论文
溯源树
样例
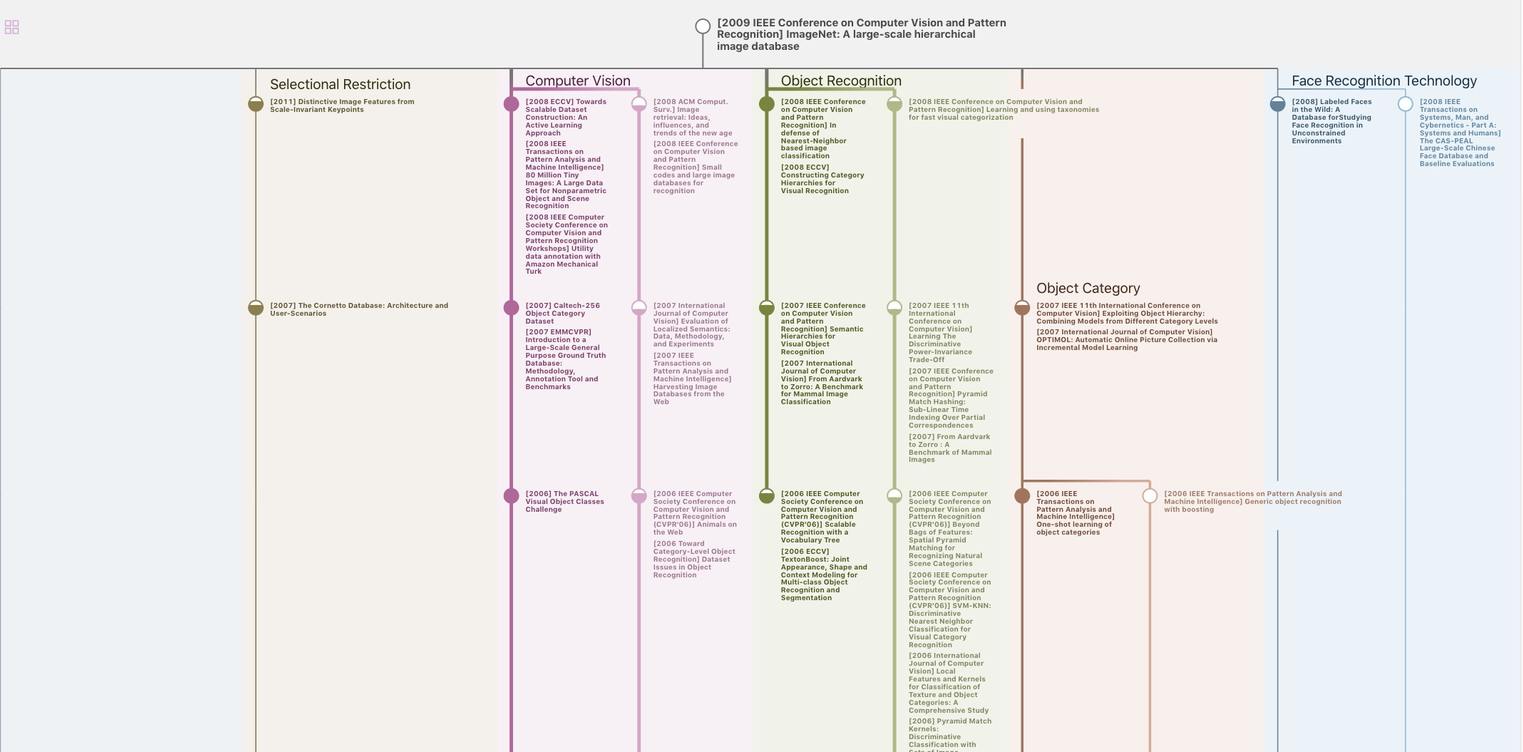
生成溯源树,研究论文发展脉络
Chat Paper
正在生成论文摘要