Medical Dialogue Response Generation with Pivotal Information Recalling
Proceedings of the 28th ACM SIGKDD Conference on Knowledge Discovery and Data Mining(2022)
摘要
Medical dialogue generation is an important yet challenging task. Most previous works rely on the attention mechanism and large-scale pretrained language models. However, these methods often fail to acquire pivotal information from the long dialogue history to yield an accurate and informative response, due to the fact that the medical entities usually scatters throughout multiple utterances along with the complex relationships between them. To mitigate this problem, we propose a medical response generation model with Pivotal Information Recalling (MedPIR), which is built on two components, i.e., knowledge-aware dialogue graph encoder and recall-enhanced generator. The knowledge-aware dialogue graph encoder constructs a dialogue graph by exploiting the knowledge relationships between entities in the utterances, and encodes it with a graph attention network. Then, the recall-enhanced generator strengthens the usage of these pivotal information by generating a summary of the dialogue before producing the actual response. Experimental results on two large-scale medical dialogue datasets show that MedPIR outperforms the strong baselines in BLEU scores and medical entities F1 measure.
更多查看译文
关键词
medical dialogue generation,pivotal information recalling
AI 理解论文
溯源树
样例
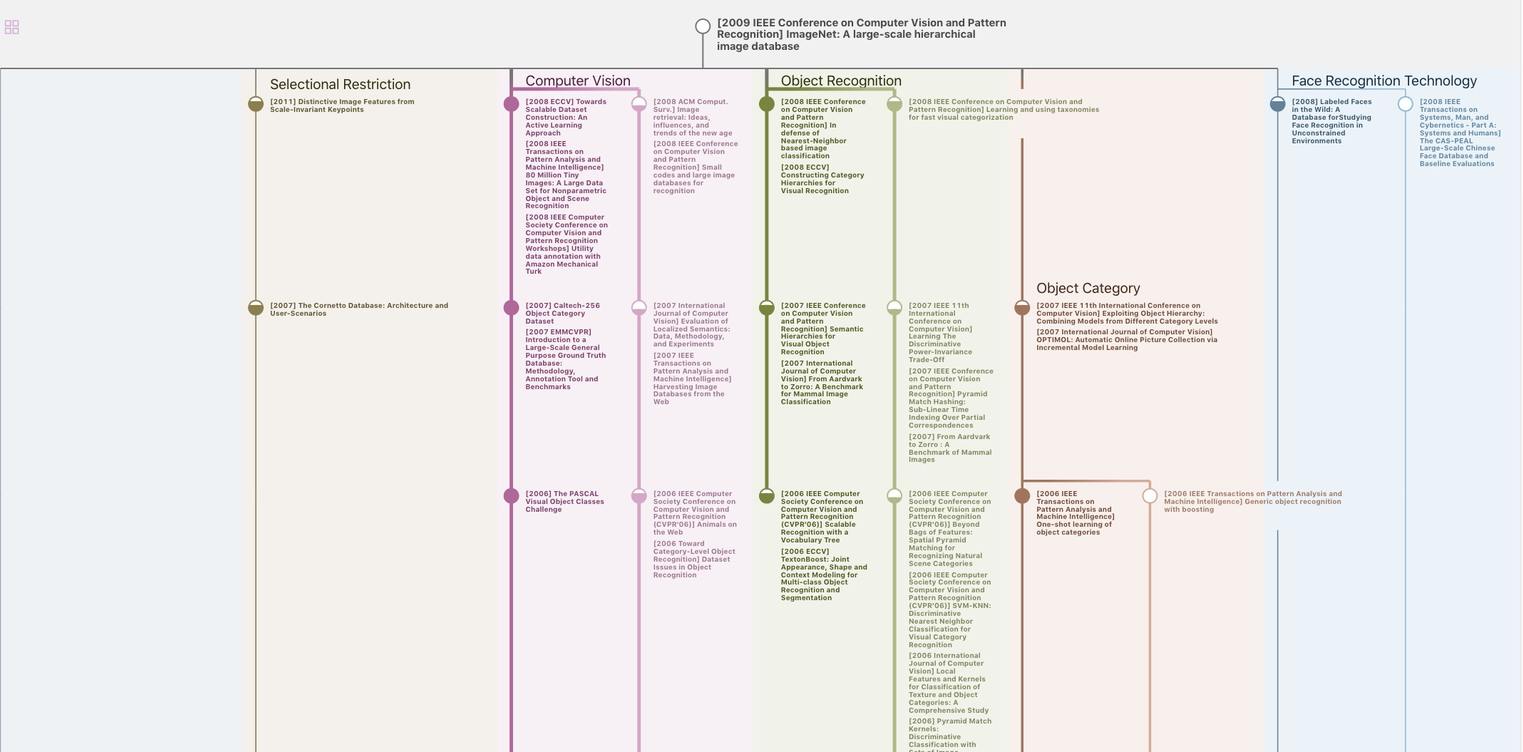
生成溯源树,研究论文发展脉络
Chat Paper
正在生成论文摘要