Masked Autoencoders for Generic Event Boundary Detection CVPR'2022 Kinetics-GEBD Challenge
arxiv(2022)
摘要
Generic Event Boundary Detection (GEBD) tasks aim at detecting generic, taxonomy-free event boundaries that segment a whole video into chunks. In this paper, we apply Masked Autoencoders to improve algorithm performance on the GEBD tasks. Our approach mainly adopted the ensemble of Masked Autoencoders fine-tuned on the GEBD task as a self-supervised learner with other base models. Moreover, we also use a semi-supervised pseudo-label method to take full advantage of the abundant unlabeled Kinetics-400 data while training. In addition, we propose a soft-label method to partially balance the positive and negative samples and alleviate the problem of ambiguous labeling in this task. Lastly, a tricky segmentation alignment policy is implemented to refine boundaries predicted by our models to more accurate locations. With our approach, we achieved 85.94% on the F1-score on the Kinetics-GEBD test set, which improved the F1-score by 2.31% compared to the winner of the 2021 Kinetics-GEBD Challenge. Our code is available at https://github.com/ContentAndMaterialPortrait/MAE-GEBD.
更多查看译文
AI 理解论文
溯源树
样例
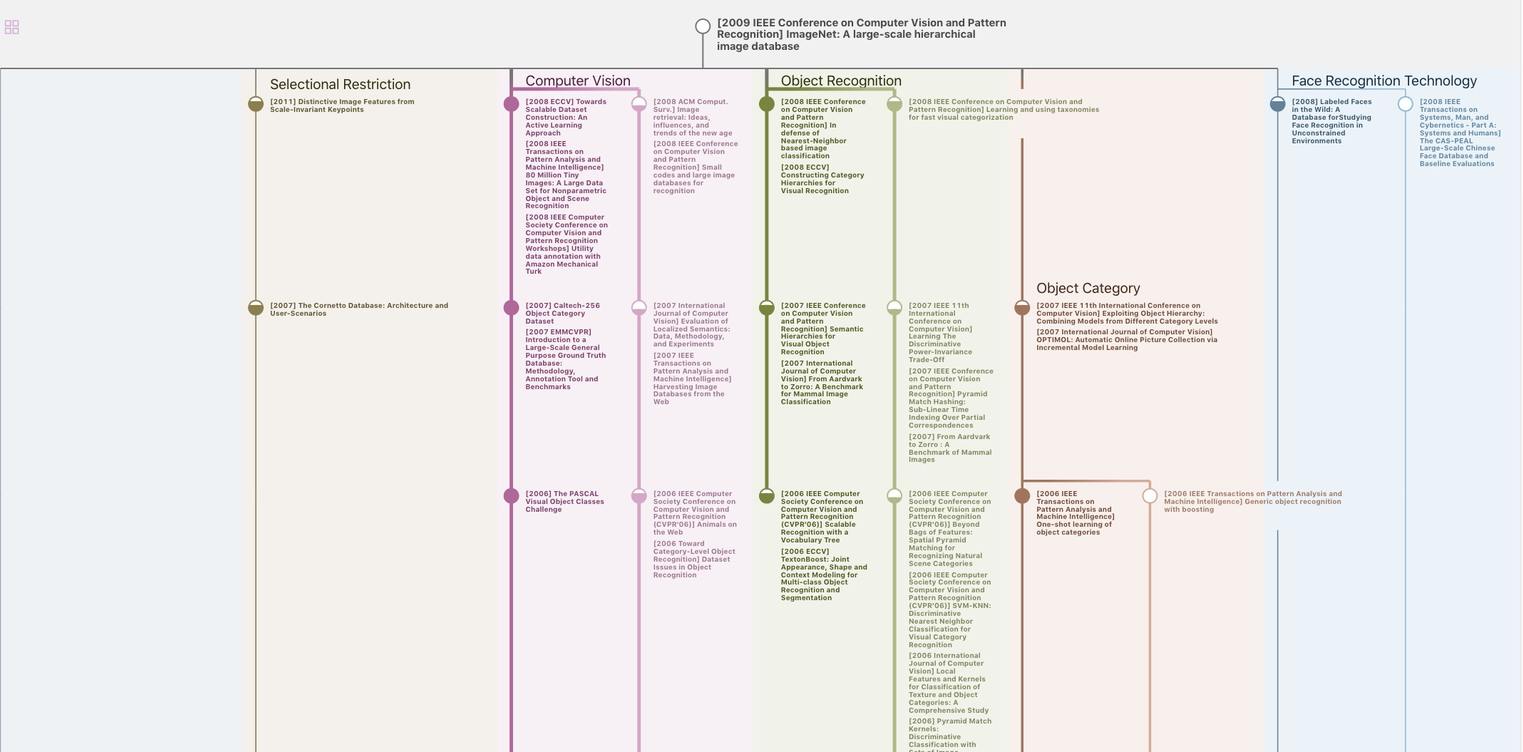
生成溯源树,研究论文发展脉络
Chat Paper
正在生成论文摘要