Improving Generalization of Metric Learning via Listwise Self-distillation
arxiv(2022)
摘要
Most deep metric learning (DML) methods employ a strategy that forces all positive samples to be close in the embedding space while keeping them away from negative ones. However, such a strategy ignores the internal relationships of positive (negative) samples and often leads to overfitting, especially in the presence of hard samples and mislabeled samples. In this work, we propose a simple yet effective regularization, namely Listwise Self-Distillation (LSD), which progressively distills a model's own knowledge to adaptively assign a more appropriate distance target to each sample pair in a batch. LSD encourages smoother embeddings and information mining within positive (negative) samples as a way to mitigate overfitting and thus improve generalization. Our LSD can be directly integrated into general DML frameworks. Extensive experiments show that LSD consistently boosts the performance of various metric learning methods on multiple datasets.
更多查看译文
关键词
metric learning,generalization,listwise
AI 理解论文
溯源树
样例
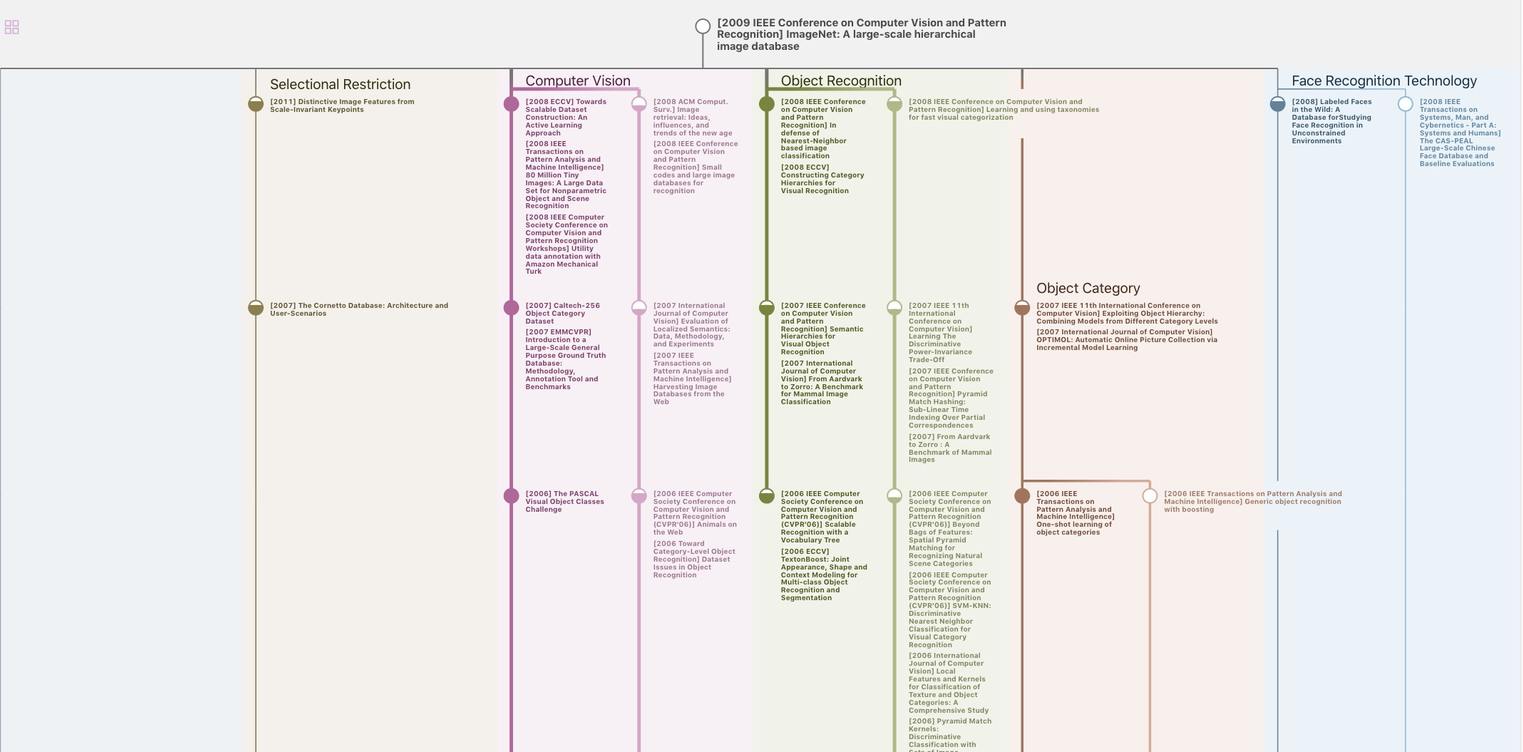
生成溯源树,研究论文发展脉络
Chat Paper
正在生成论文摘要