Scalability of effective adherence interventions for patients using cardiovascular disease medication: A realist synthesis-inspired systematic review
British journal of clinical pharmacology(2023)
摘要
Upscaling of medication adherence interventions to routine care is still challenging. This realist theory-inspired review aimed to assess which intervention aspects are potentially important for the scalability of effective cardiovascular disease (CVD) medication adherence interventions and how they are reported in effectiveness studies. A total of 4097 articles from four databases were screened of which ultimately 31 studies were included. Relevant information on scalability was extracted using a theoretic framework based on the scalability assessment tool used in the QUALIDEC study for the following domains: (i) innovation, (ii) implementers and patients, (iii) adopting organizations and health system, and (iv) socio-political context. Extracted articles were analysed for themes and chains of inference, which were grouped based on commonality and source of evidence to form new hypotheses. Six different domains relevant for scalability of adherence interventions were identified: (1) Complexity of the intervention; (2) training; (3) customization of the intervention; (4) drivers of the intervention; (5) technical interventions; and (6) stakeholder involvement. These six domains might be useful for the development of more scalable interventions by bridging the gap between research and practice. Data relevant for scalability is not well reported on in effectiveness trials for CVD medication adherence interventions and only limited data on scalability has been published in additional papers. We believe the adoption and reach of effective CVD medication adherence interventions will improve with increased awareness for the necessity of scalability in all phases of intervention development.
更多查看译文
关键词
effective adherence interventions,cardiovascular disease medication,systematic review,cardiovascular disease
AI 理解论文
溯源树
样例
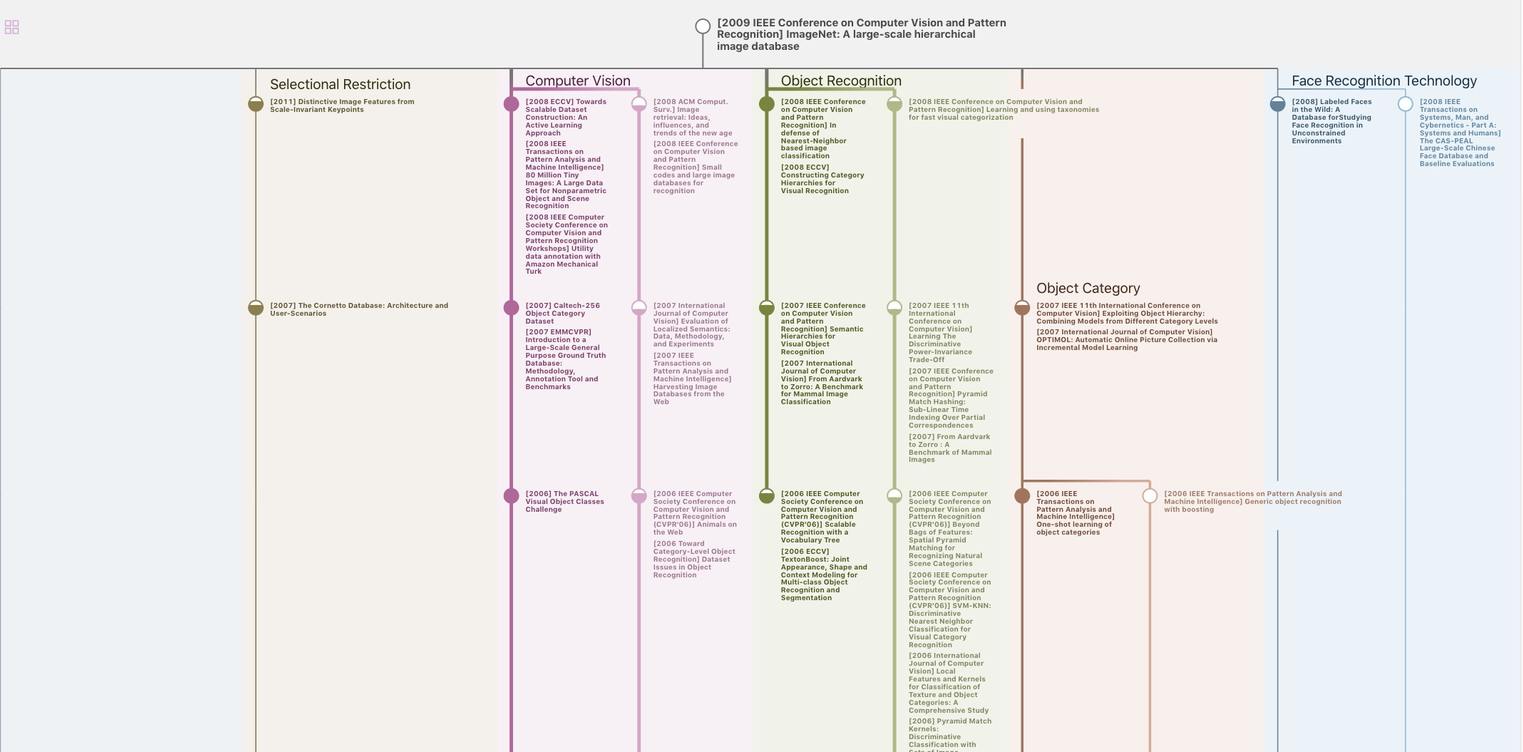
生成溯源树,研究论文发展脉络
Chat Paper
正在生成论文摘要