Spatial Enhanced Pattern Through Graph Convolutional Neural Network for Epileptic EEG Identification
INTERNATIONAL JOURNAL OF NEURAL SYSTEMS(2022)
摘要
Feature extraction is an essential procedure in the detection and recognition of epilepsy, especially for clinical applications. As a type of multichannel signal, the association between all of the channels in EEG samples can be further utilized. To implement the classification of epileptic seizures from the nonseizures in EEG samples, one graph convolutional neural network (GCNN)-based framework is proposed for capturing the spatial enhanced pattern of multichannel signals to characterize the behavior of EEG activity, which is capable of visualizing the salient regions in each sequence of EEG samples. Meanwhile, the presented GCNN could be exploited to discriminate normal, ictal and interictal EEGs as a novel classifier. To evaluate the proposed approach, comparison experiments were conducted between state-of-the-art techniques and ours. From the experimental results, we found that for ictal and interictal EEG signal discrimination, the presented approach can achieve a sensitivity of 98.33%, specificity of 99.19% and accuracy of 98.38%.
更多查看译文
关键词
Epileptic seizure, electroencephalogram, classification, feature extraction
AI 理解论文
溯源树
样例
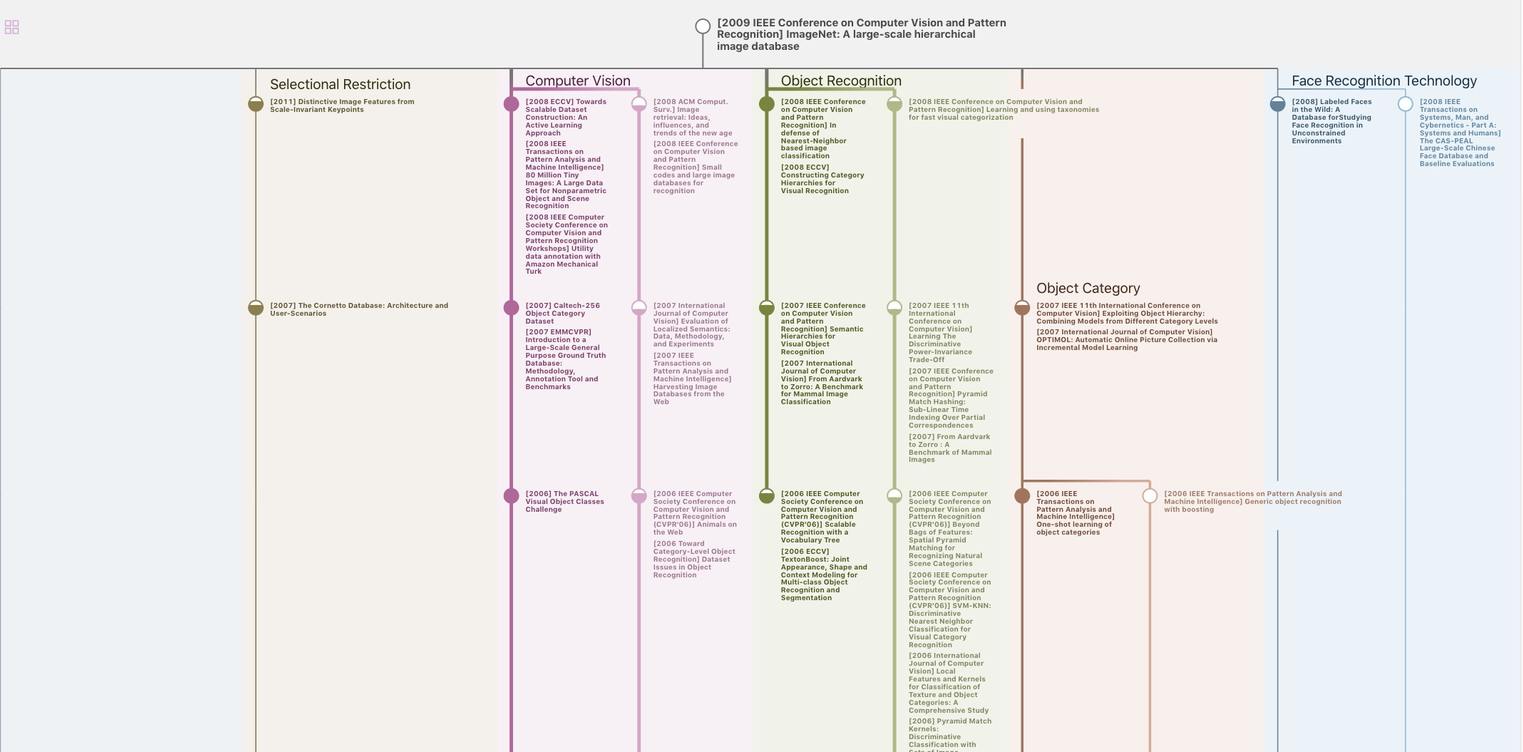
生成溯源树,研究论文发展脉络
Chat Paper
正在生成论文摘要