Defend Against Jamming Attacks Using Deep Reinforcement Learning
2021 13th International Symposium on Antennas, Propagation and EM Theory (ISAPE)(2021)
摘要
Cognitive radio network has the learning ability to adjust transmission behavior to adapt to dynamic electromagnetic environment, and it has become a research hotspot in the field of the new generation of intelligent wireless communication systems. However, recent research results show that cognitive radio networks are vulnerable to interference attacks, resulting in a serious decline in spectrum utilization. In this paper, an intelligent anti-jamming scheme based on deep reinforcement learning is proposed, and the anti-jamming decision of the communication party is modeled as a Markov decision chain. This method can help the communication transmitter to learn a combination of communication parameters that can maximize the probability of communication success. In the specific implementation, we use Double DQN as the communication decision-making brain, and specially designed a convolutional neural network suitable for the communication spectrum as input to accurately estimate the action value function. The simulation results show that our method has better performance than the traditional anti-jamming method based on reinforcement learning.
更多查看译文
关键词
Markov decision chain,communication success probability,communication decision-making brain,convolutional neural network,communication spectrum,antijamming method,jamming attacks,deep reinforcement learning,cognitive radio network,dynamic electromagnetic environment,intelligent wireless communication systems,interference attacks,spectrum utilization,intelligent antijamming scheme,double DQN
AI 理解论文
溯源树
样例
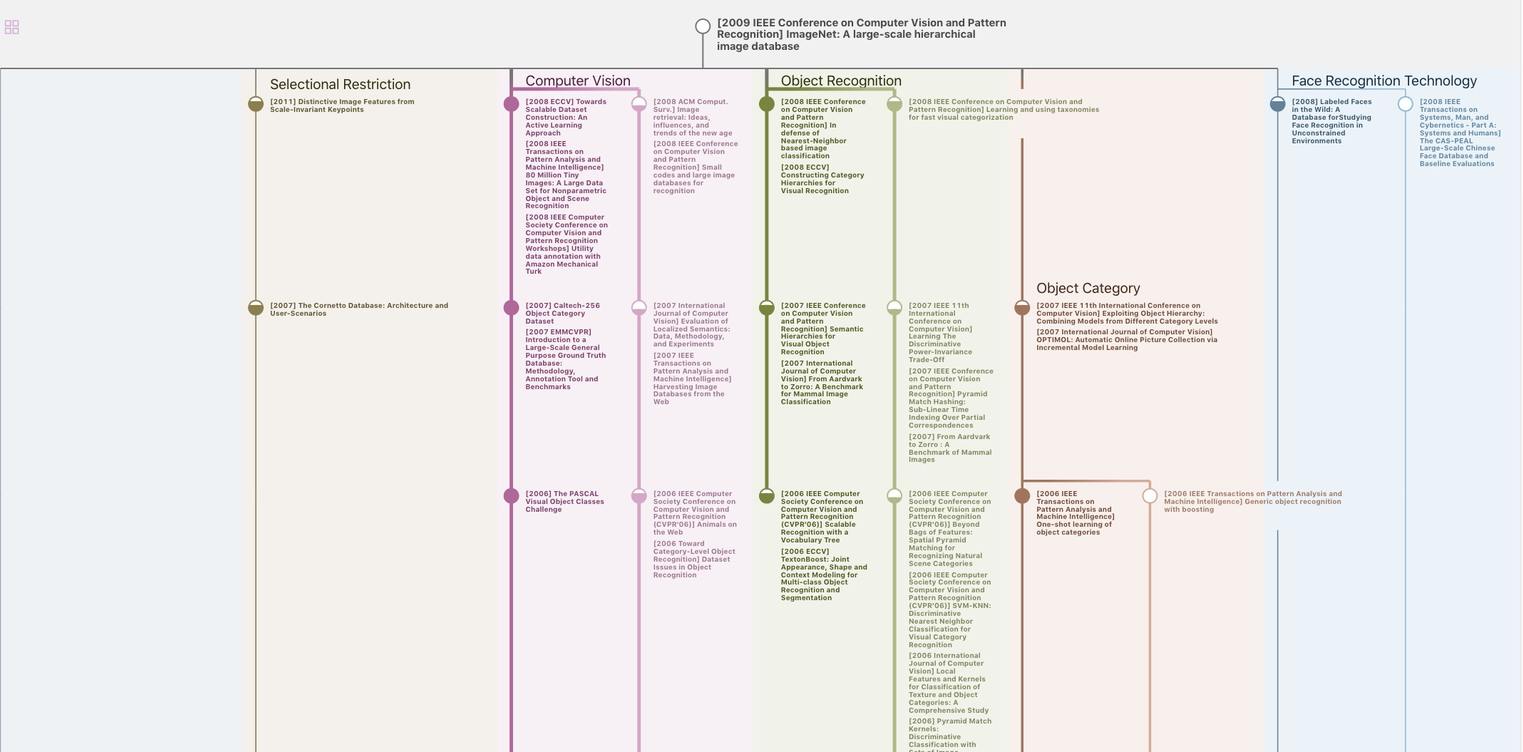
生成溯源树,研究论文发展脉络
Chat Paper
正在生成论文摘要