Heterogeneous Graph Convolution Based on In-Domain Self-Supervision for Multimodal Sentiment Analysis
Social Science Research Network(2022)
摘要
The inability to fully exploit domain-specific knowledge and the lack of an effective integration method have been the difficulties and focus of multimodal sentiment analysis. In this paper, we propose heterogeneous graph convolution with in-domain self-supervised multi-task learning for multimodal sentiment analysis (HIS-MSA) to solve these problems. Firstly, HIS-MSA carries out the second pre-trained with different self-supervised training strategies to fully mine the unique knowledge of the in-domain corpus, and give BERT the awareness of professional field. Secondly, HIS-MSA uses heterogeneous graph, which is good at integrating heterogeneous knowledge, to fuse feature from multiple sources. Finally, a unimodal label generation module is used to jointly guide multimodal tasks and unimodal tasks to balance independent and complementary information between the modalities. We conducted experiments on the datasets MOSI and MOSEI, which have 2199 and 23454 video segments respectively. The results show an average improvement of approximately 1.5 points in all metrics compared to the current state-of-the-art model.
更多查看译文
关键词
Multimodal sentiment analysis,Self-supervised learning,In-domain pretrained,Heterogeneous graph convolution,Multi-task learning
AI 理解论文
溯源树
样例
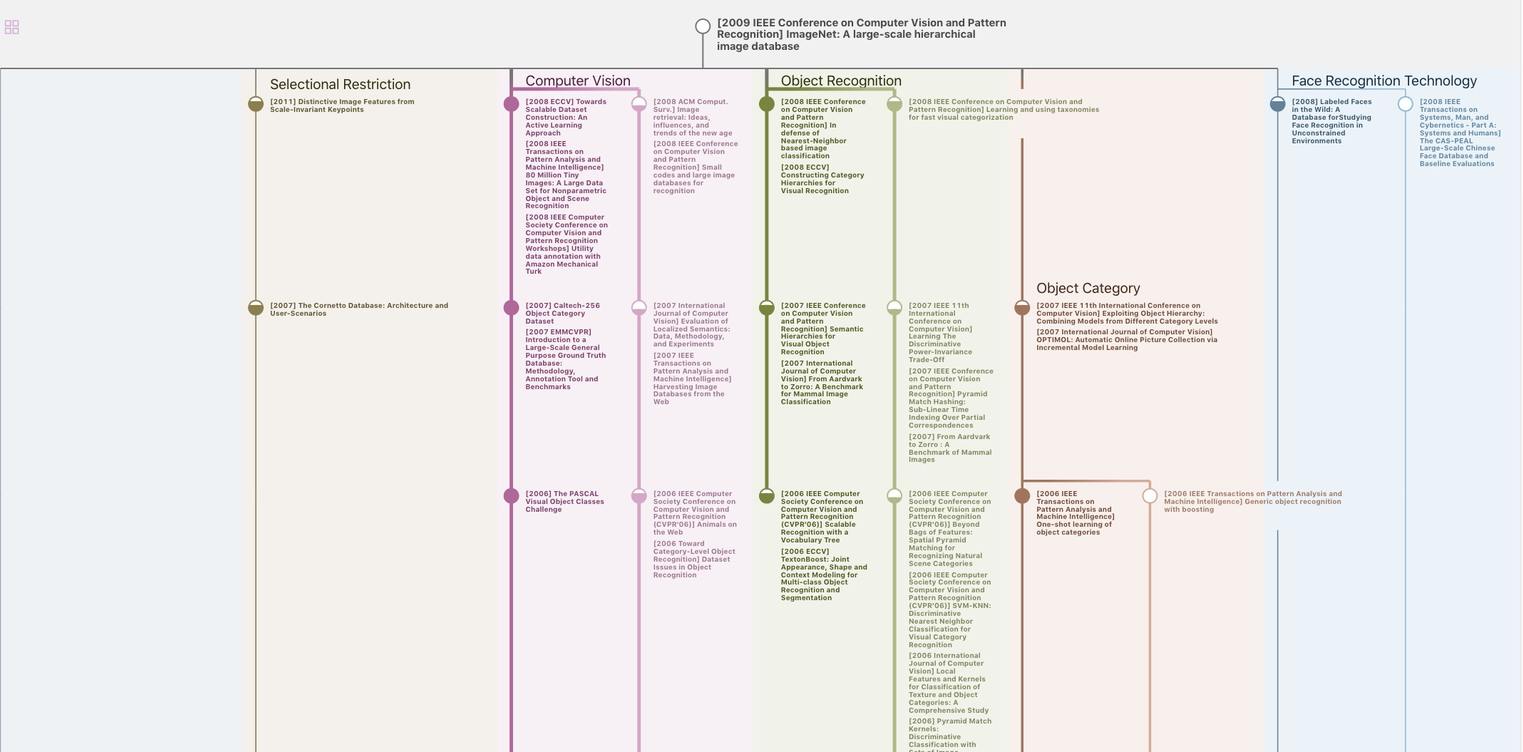
生成溯源树,研究论文发展脉络
Chat Paper
正在生成论文摘要