TDNCIB: Target-Driven Navigation with Convolutional Information Branch Based on Deep Reinforcement Learning
2021 China Automation Congress (CAC)(2021)
摘要
Target-driven navigation has been a significant application field of reinforcement learning. However, sparse reward and lack of useful environment information are still existing problems. Therefore, in this paper, we propose a novel design to the target-driven visual navigation based on deep reinforcement learning: Target-Driven Navigation with Convolutional Information Branch (TDNCIB), which enriches the high-dimensional information learned by the network from the environment. It is an improvement of A2C algorithm. CIB can help make full use of environmental information and serve as a complement to the baseline. Besides, a novel reward design using similarity is introduced to relieve sparse reward. The ablation study is implemented in 4 different scenes of AI2-THOR framework comparing the baseline and our TDNCIB. According to our evaluation, TDNCIB converges faster than the baseline and achieves better performance in 5 common metrics with shorter trajectory length, less collision and higher success rate.
更多查看译文
关键词
deep reinforcement learning,sparse reward,target-driven visual navigation,TDNCIB,high-dimensional information,environmental information,convolutional information branch,A2C algorithm,AI2-THOR framework
AI 理解论文
溯源树
样例
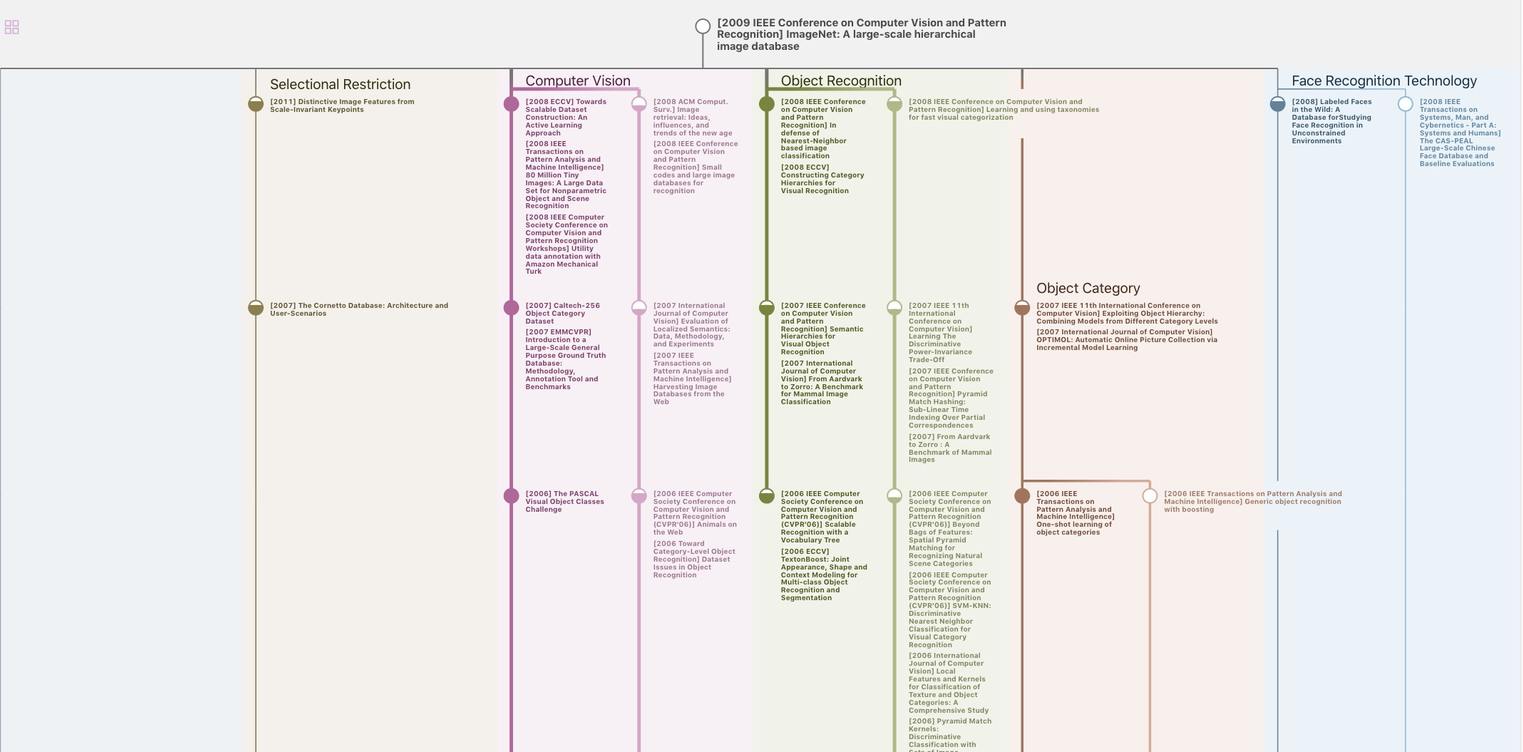
生成溯源树,研究论文发展脉络
Chat Paper
正在生成论文摘要