Novel Instance Mining with Pseudo-Margin Evaluation for Few-Shot Object Detection
ICASSP 2022 - 2022 IEEE International Conference on Acoustics, Speech and Signal Processing (ICASSP)(2022)
摘要
Few-shot object detection (FSOD) enables the detector to recognize novel objects only using limited training samples, which could greatly alleviate model’s dependency on data. Most existing methods include two training stages, namely base training and fine-tuning. However, the unlabeled novel instances in the base set were untouched in previous works, which can be re-used to enhance the FSOD performance. Thus, a new instance mining model is proposed in this paper to excavate the novel samples from the base set. The detector is thus fine-tuned again by these additional free novel instances. Meanwhile, a novel pseudo-margin evaluation algorithm is designed to address the quality problem of pseudo-labels brought by those new novel instances. The experimental results on MS-COCO dataset show the effectiveness of the proposed model, which does not require any additional training samples or parameters. Our code is available at: https://github.com/liuweijie19980216/NimPme.
更多查看译文
关键词
Few-shot object detection,pseudo-labels,pseudo-margin evaluation
AI 理解论文
溯源树
样例
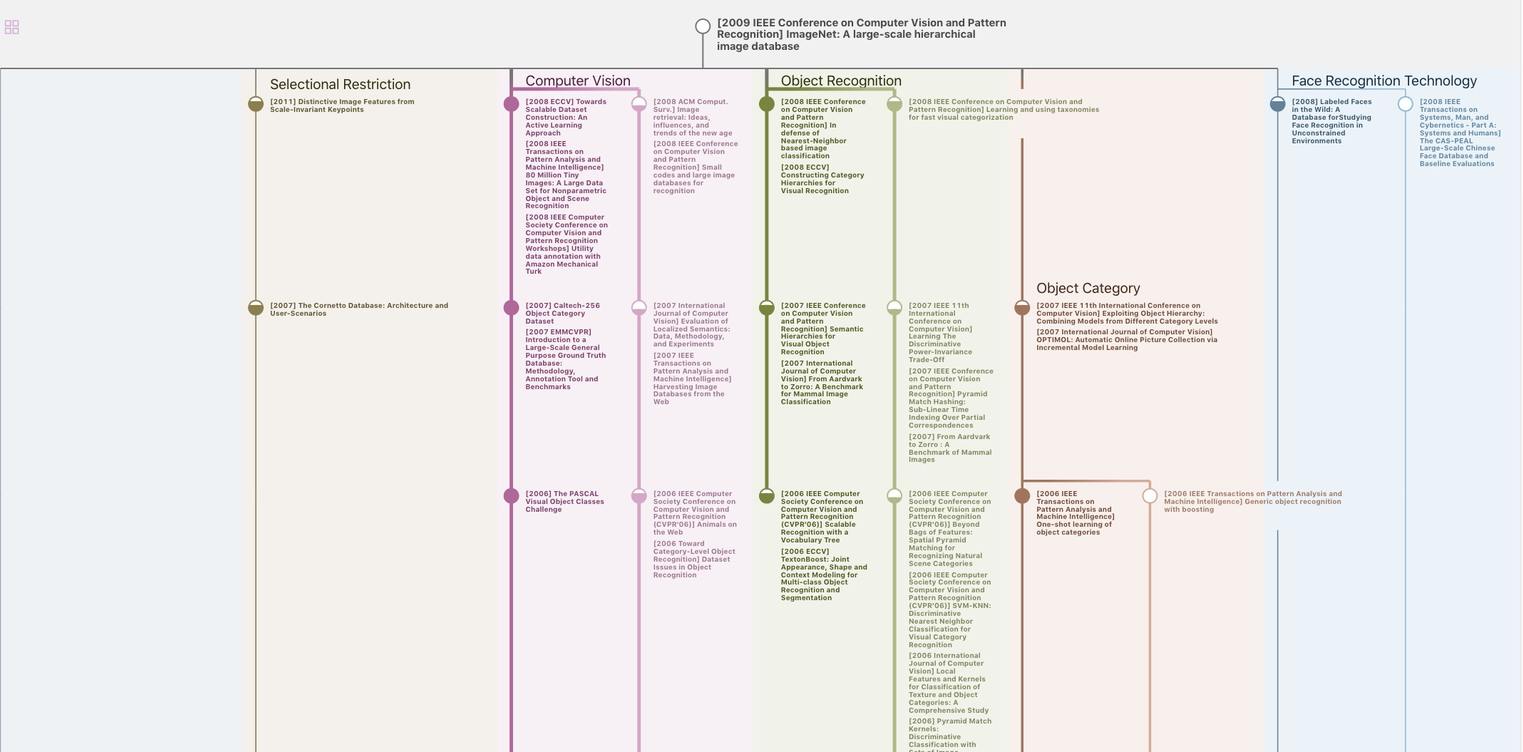
生成溯源树,研究论文发展脉络
Chat Paper
正在生成论文摘要