A pre-training method for 3d building point cloud semantic segmentation
ISPRS Annals of the Photogrammetry, Remote Sensing and Spatial Information Sciences(2022)
摘要
Abstract. As a result of the success of Deep Learning (DL) techniques, DL-based approaches for extracting information from 3D building point clouds have evolved in recent years. Despite noteworthy progress in existing methods for interpreting point clouds, the excessive cost of annotating 3D data has resulted in DL-based 3D point cloud understanding tasks still lagging those for 2D images. The notion that pre-training a network on a large source dataset may help enhance performance after it is fine-tuned on the target task and dataset has proved vital in numerous tasks in the Natural Language Processing (NLP) domain. This paper proposes a straightforward but effective pre-training method for 3D building point clouds that learns from a large source dataset. Specifically, it first learns the ability of semantic segmentation by pre-training on a cross-domain source Stanford 3D Indoor Scene Dataset. It then initialises the downstream networks with the pre-trained weights. Finally, the models are fine-tuned with the target building scenes obtained from the ArCH benchmarking dataset. Our paper evaluates the proposed method by employing four fully supervised networks as backbones. The results of two pipelines are compared between training from scratch and pre-training. The results illustrate that pre-training on the source dataset can consistently improve the performance of the target dataset with an average gain of 3.9%.
更多查看译文
关键词
3D Building Point Cloud, Deep Learning, Fine-tune, Pre-training, Transfer Learning
AI 理解论文
溯源树
样例
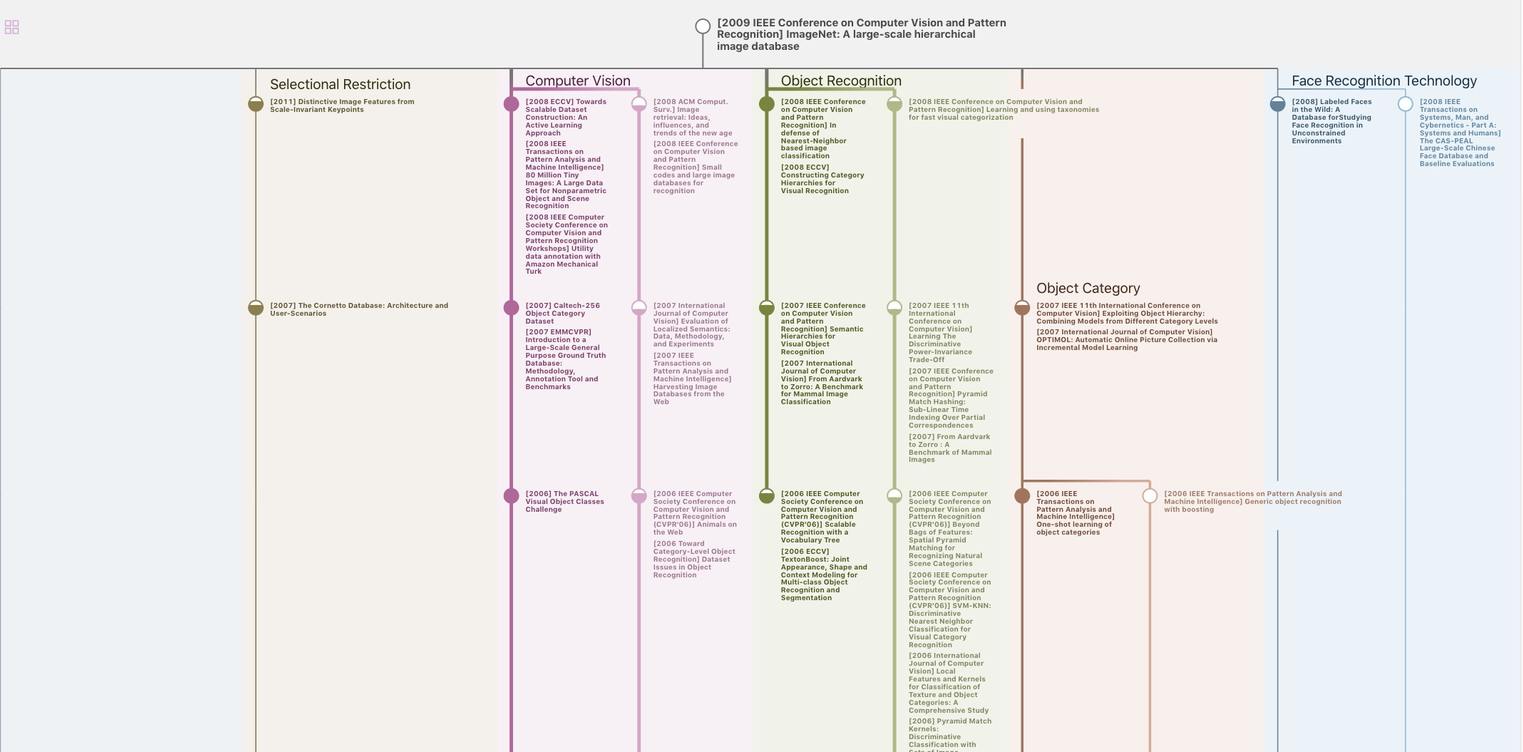
生成溯源树,研究论文发展脉络
Chat Paper
正在生成论文摘要