Cross-Session EEG-Based Emotion Recognition Via Maximizing Domain Discrepancy
2022 3rd International Conference on Electronic Communication and Artificial Intelligence (IWECAI)(2022)
摘要
Cross-session pose a challenge to the application of EEG-based emotion recognition (ER), which is due to the non-stationary nature of EEG that causes the EEG to reveal distribution discrepancies over time, thereby leading to degradation of performance. The traditional way is by collecting labelled data over multiple sessions and then retraining a new model, but this is time-consuming and labor-intensive. In this paper, we propose the Maximizing Domain Discrepancy for EEG-based ER (MDD-ER) to improve cross-session performance. MDD-ER applies distinct domain adaptation strategies to alleviate feature distribution discrepancies between source session and target session at different levels: for shallow features, we use maximum mean discrepancy (MMD) to align the source and target domains based on statistical criterion, and for deep features, we adversarially train two classifiers, which effectively improves the alignment precision of the source and target domains due to the consideration of the class of features. Consequently, the proposed MDD-ER method can improve model generalization across sessions. We conduct comprehensive experiments on SEED dataset, and the experimental results demonstrate the effectiveness of the proposed MDD-ER method in cross-session ER.
更多查看译文
关键词
Emotion recognition,Electroencephalography,Deep learning,Domain Adaption
AI 理解论文
溯源树
样例
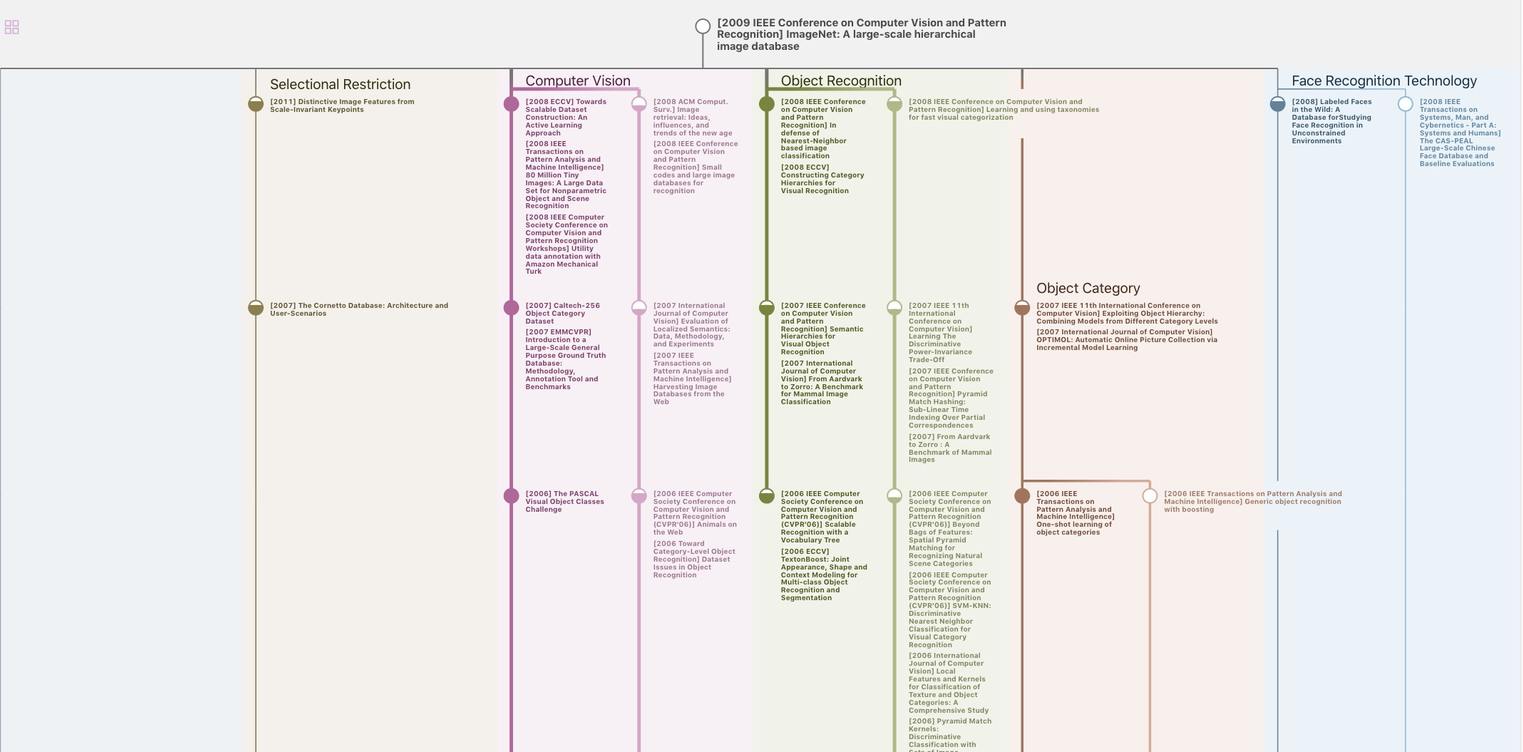
生成溯源树,研究论文发展脉络
Chat Paper
正在生成论文摘要