Explainable global error weighted on feature importance: The xGEWFI metric to evaluate the error of data imputation and data augmentation
arxiv(2023)
摘要
Evaluating data imputation and augmentation performance is a critical issue in data science. In statistics, methods like Kolmogorov-Smirnov K-S test, Cramér-von Mises W^2 , Anderson-Darling A^2 , Pearson’s χ ^2 and Watson’s U^2 exists for decades to compare the distribution of two datasets. In the context of data generation, typical evaluation metrics have the same flaw: They calculate the feature’s error and the global error on the generated data without weighting the error with the feature’s importance. In most cases, the importance of the features is imbalanced, and it can induce a bias on the features and global errors. This paper proposes a novel metric named “Explainable Global Error Weighted on Feature Importance” ( xGEWFI ). This new metric is tested in a whole preprocessing method that 1. Process the outliers, 2. impute the missing data, and 3. augments the data. At the end of the process, the xGEWFI error is calculated. The distribution error between the original and generated data is calculated using a Kolmogorov-Smirnov test (K-S test) for each feature. Those results are multiplied by the importance of the respective features and calculated using a Random Forest (RF) algorithm. The metric result is expressed in an explainable format, aiming for an ethical AI. This novel method provides a more precise evaluation of a data generation process than if only a K-S test were used.
更多查看译文
关键词
xGEWFI,Data imputation,Data augmentation,Random forest,SMOTE,KNNImputer
AI 理解论文
溯源树
样例
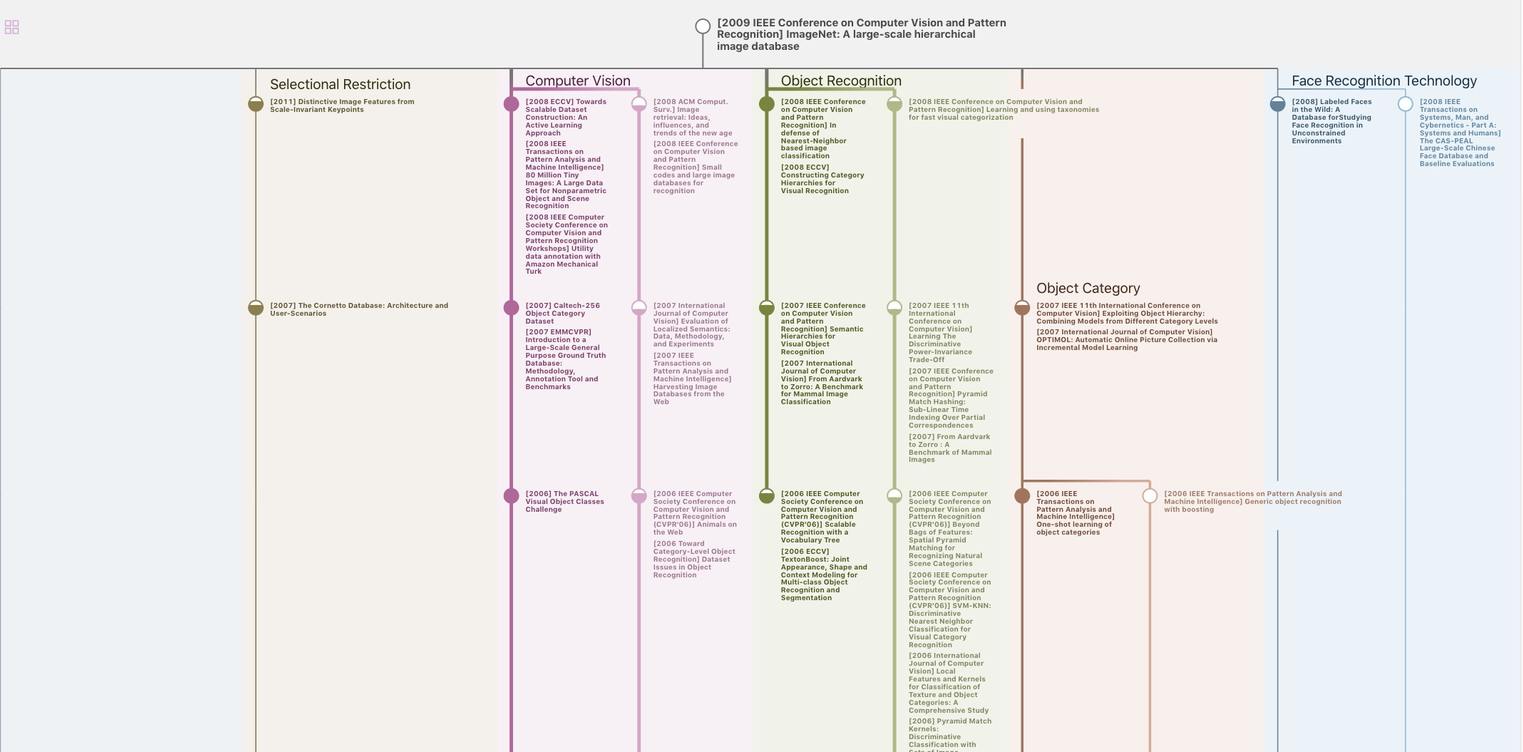
生成溯源树,研究论文发展脉络
Chat Paper
正在生成论文摘要