Diffusion models as plug-and-play priors
NeurIPS 2022(2022)
摘要
We consider the problem of inferring high-dimensional data $\mathbf{x}$ in a model that consists of a prior $p(\mathbf{x})$ and an auxiliary differentiable constraint $c(\mathbf{x},\mathbf{y})$ on $x$ given some additional information $\mathbf{y}$. In this paper, the prior is an independently trained denoising diffusion generative model. The auxiliary constraint is expected to have a differentiable form, but can come from diverse sources. The possibility of such inference turns diffusion models into plug-and-play modules, thereby allowing a range of potential applications in adapting models to new domains and tasks, such as conditional generation or image segmentation. The structure of diffusion models allows us to perform approximate inference by iterating differentiation through the fixed denoising network enriched with different amounts of noise at each step. Considering many noised versions of $\mathbf{x}$ in evaluation of its fitness is a novel search mechanism that may lead to new algorithms for solving combinatorial optimization problems.
更多查看译文
关键词
diffusion models,conditional generation,image segmentation
AI 理解论文
溯源树
样例
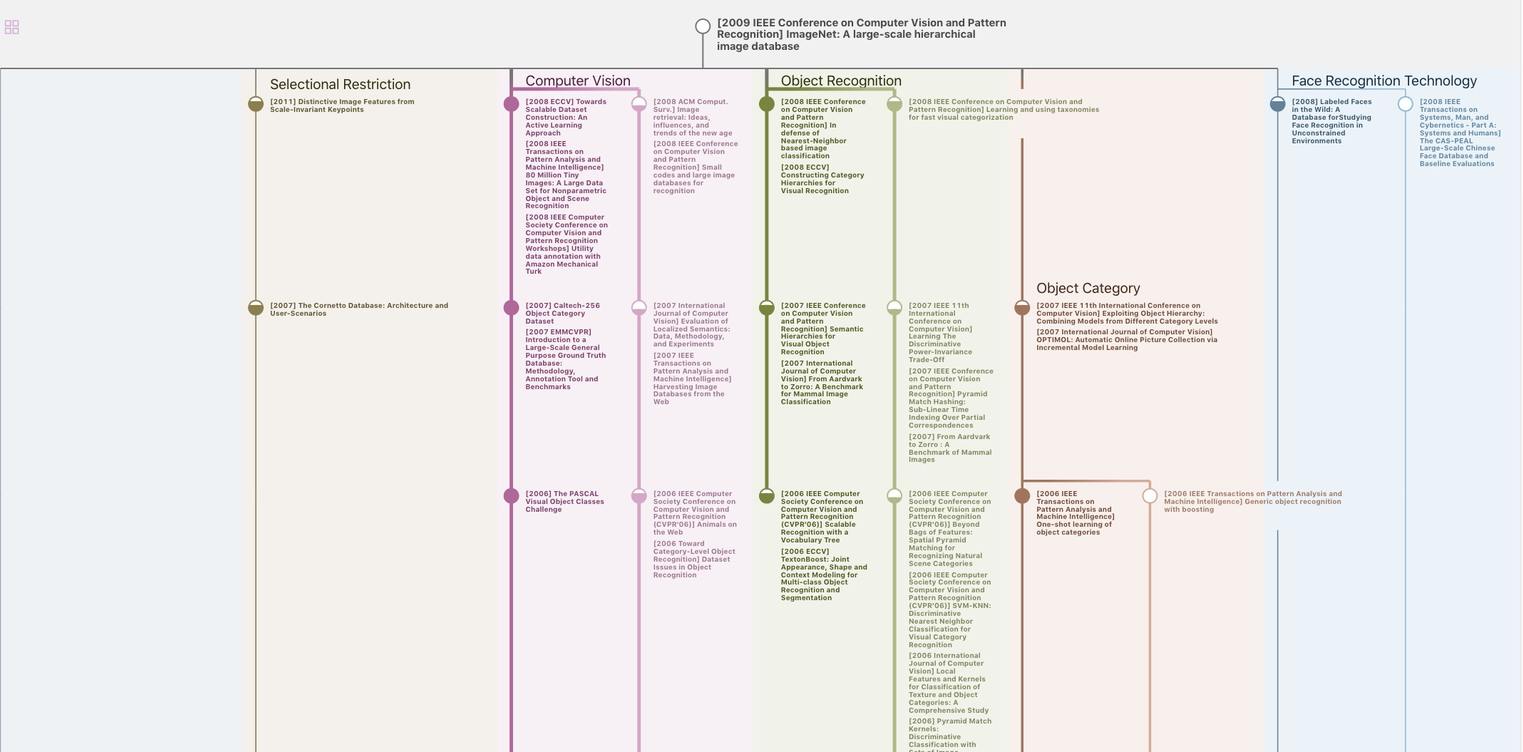
生成溯源树,研究论文发展脉络
Chat Paper
正在生成论文摘要