Redundancy Reduction Twins Network: A Training framework for Multi-output Emotion Regression
arxiv(2022)
摘要
In this paper, we propose the Redundancy Reduction Twins Network (RRTN), a redundancy reduction training framework that minimizes redundancy by measuring the cross-correlation matrix between the outputs of the same network fed with distorted versions of a sample and bringing it as close to the identity matrix as possible. RRTN also applies a new loss function, the Barlow Twins loss function, to help maximize the similarity of representations obtained from different distorted versions of a sample. However, as the distribution of losses can cause performance fluctuations in the network, we also propose the use of a Restrained Uncertainty Weight Loss (RUWL) or joint training to identify the best weights for the loss function. Our best approach on CNN14 with the proposed methodology obtains a CCC over emotion regression of 0.678 on the ExVo Multi-task dev set, a 4.8% increase over a vanilla CNN 14 CCC of 0.647, which achieves a significant difference at the 95% confidence interval (2-tailed).
更多查看译文
关键词
emotion,network,training framework,multi-output
AI 理解论文
溯源树
样例
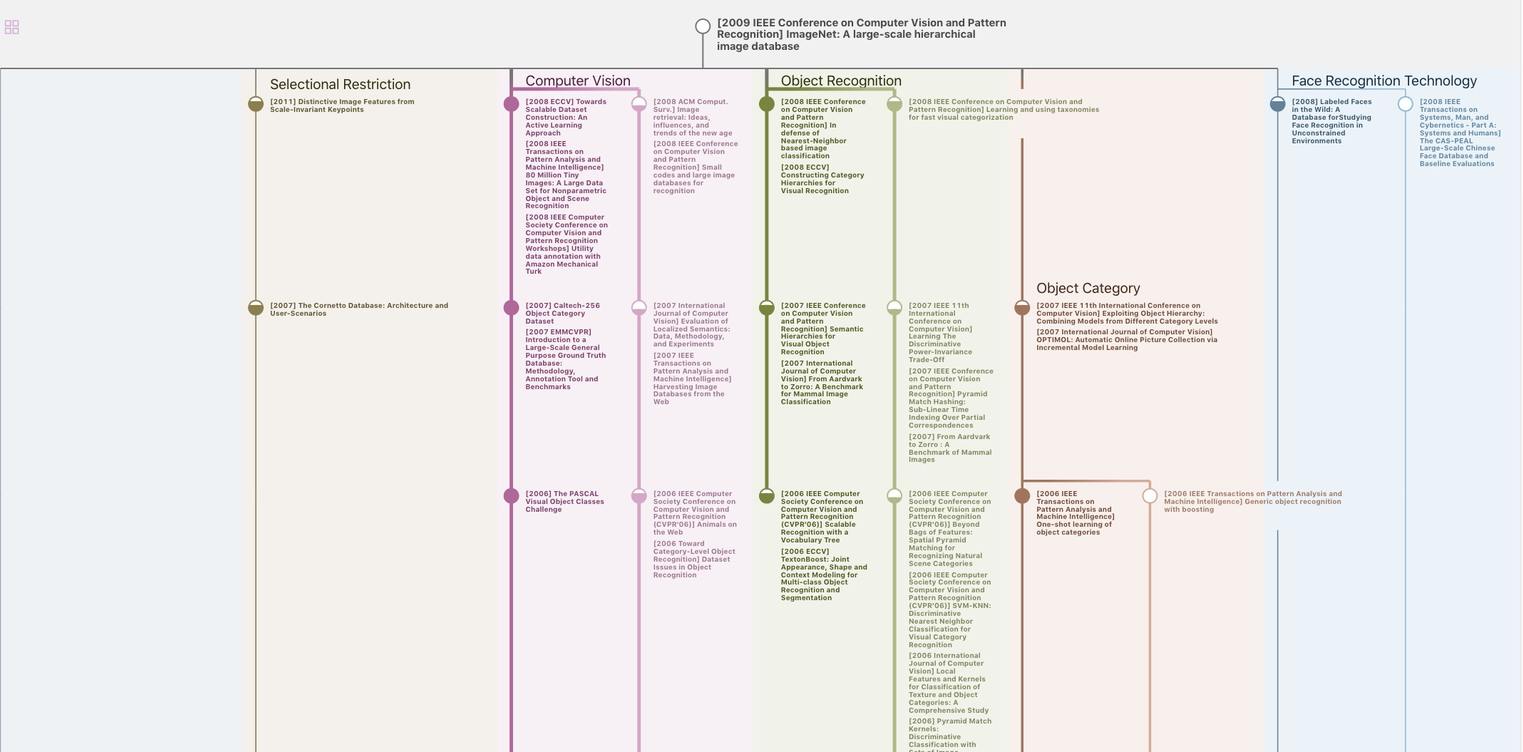
生成溯源树,研究论文发展脉络
Chat Paper
正在生成论文摘要