WrapperFL: A Model Agnostic Plug-in for Industrial Federated Learning
arxiv(2022)
摘要
Federated learning, as a privacy-preserving collaborative machine learning paradigm, has been gaining more and more attention in the industry. With the huge rise in demand, there have been many federated learning platforms that allow federated participants to set up and build a federated model from scratch. However, exiting platforms are highly intrusive, complicated, and hard to integrate with built machine learning models. For many real-world businesses that already have mature serving models, existing federated learning platforms have high entry barriers and development costs. This paper presents a simple yet practical federated learning plug-in inspired by ensemble learning, dubbed WrapperFL, allowing participants to build/join a federated system with existing models at minimal costs. The WrapperFL works in a plug-and-play way by simply attaching to the input and output interfaces of an existing model, without the need of re-development, significantly reducing the overhead of manpower and resources. We verify our proposed method on diverse tasks under heterogeneous data distributions and heterogeneous models. The experimental results demonstrate that WrapperFL can be successfully applied to a wide range of applications under practical settings and improves the local model with federated learning at a low cost.
更多查看译文
关键词
learning,wrapperfl,model
AI 理解论文
溯源树
样例
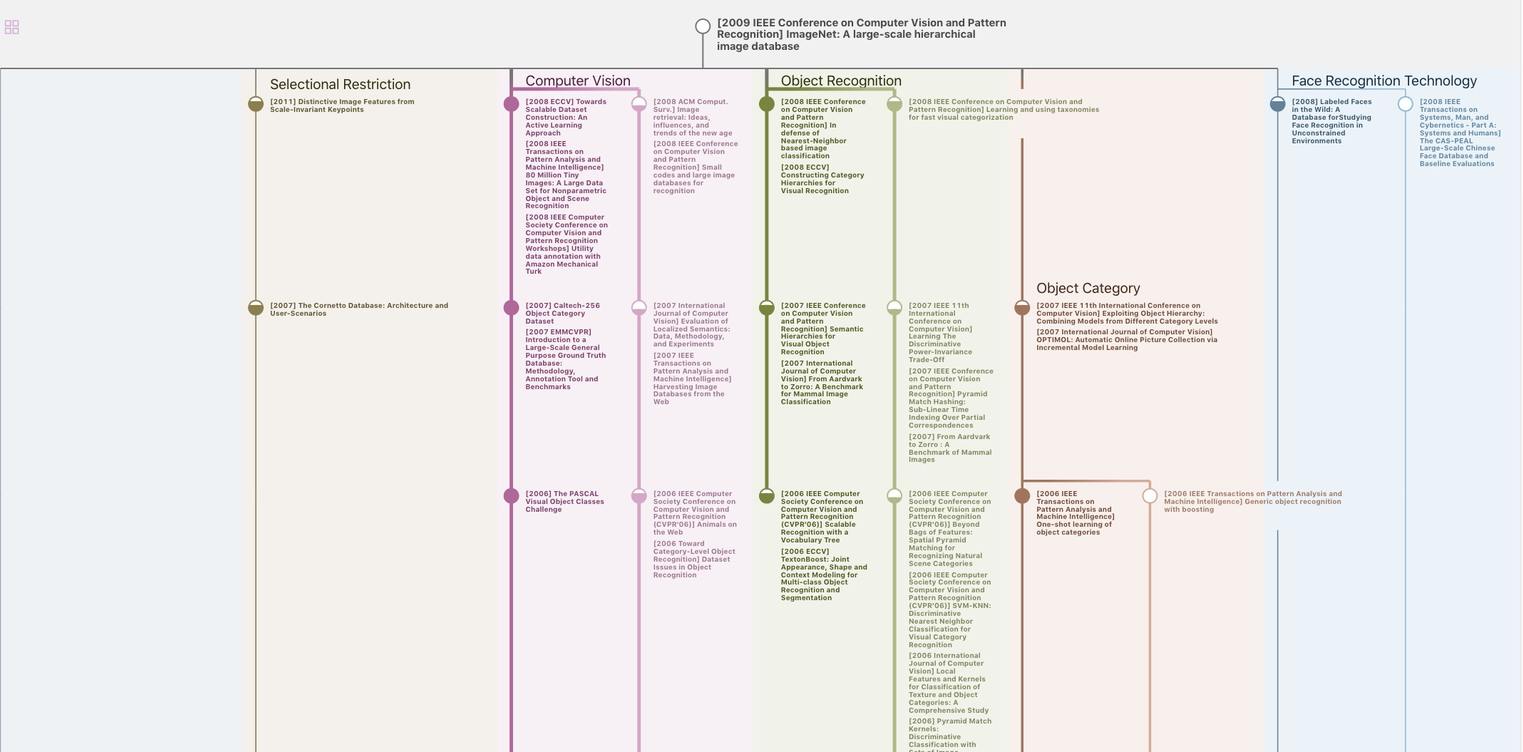
生成溯源树,研究论文发展脉络
Chat Paper
正在生成论文摘要