Autoencoder-based anomaly detector for gear tooth bending fatigue cracks
Proceedings of the Annual Conference of the Prognostics and Health Management Society(2021)
摘要
The article reports on anomaly detection performance of data-driven models based on a few selected autoencoder topologies and compares them to the performance of a set of popular classical vibration-based condition indicators. The evaluation of these models employed data that consisted of baseline gearbox runs and the associated runs with seeded bending cracks in the root of the gear teeth for eight different gear pairings. The analyses showed that the data-driven models, trained on a subset of baseline data outperformed classical CIs as anomaly detectors.
更多查看译文
AI 理解论文
溯源树
样例
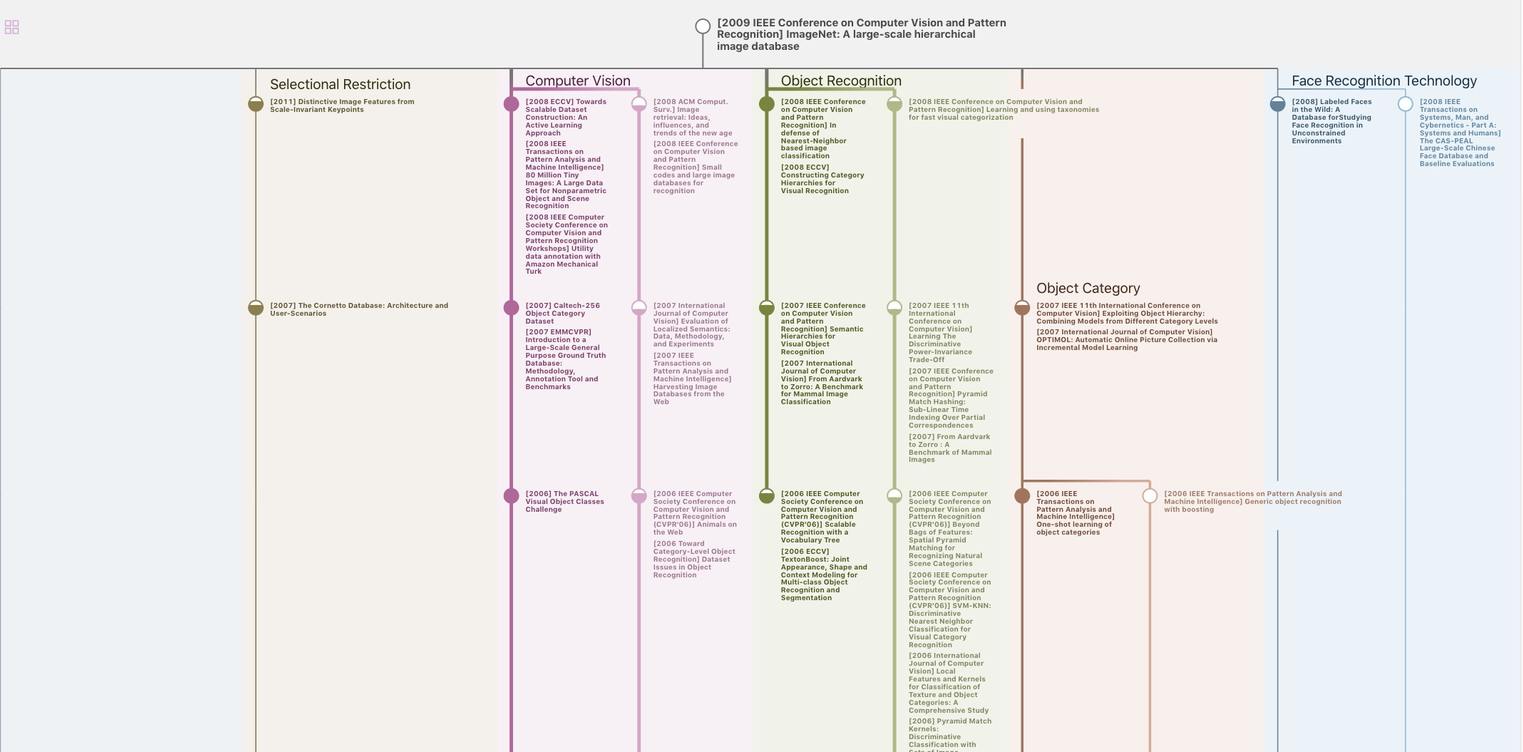
生成溯源树,研究论文发展脉络
Chat Paper
正在生成论文摘要