RB-Net: Training Highly Accurate and Efficient Binary Neural Networks With Reshaped Point-Wise Convolution and Balanced Activation
IEEE Transactions on Circuits and Systems for Video Technology(2022)
摘要
In this paper, we find that the conventional convolution operation becomes the bottleneck for extremely efficient binary neural networks (BNNs). To address this issue, we open up a new direction by introducing a reshaped point-wise convolution (RPC) to replace the conventional one to build BNNs. Specifically, we conduct a point-wise convolution after rearranging the spatial information into depth, with which at least
$2.25\times $
computation reduction can be achieved. Such an efficient RPC allows us to explore more powerful representational capacity of BNNs under a given computation complexity budget. Moreover, we propose to use a balanced activation (BA) to adjust the distribution of the scaled activations after binarization, which enables significant performance improvement of BNNs. After integrating RPC and BA, the proposed network, dubbed as RB-Net, strikes a good trade-off between accuracy and efficiency, achieving superior performance with lower computational cost against the state-of-the-art BNN methods. Specifically, our RB-Net achieves 66.8% Top-1 accuracy with ResNet-18 backbone on ImageNet, exceeding the state-of-the-art Real-to-Binary Net (65.4%) by 1.4% while achieving more than
$3\times $
reduction (52M vs. 165M) in computational complexity.
更多查看译文
关键词
Binary neural network,reshaped point-wise convolution,balanced activation,object classification
AI 理解论文
溯源树
样例
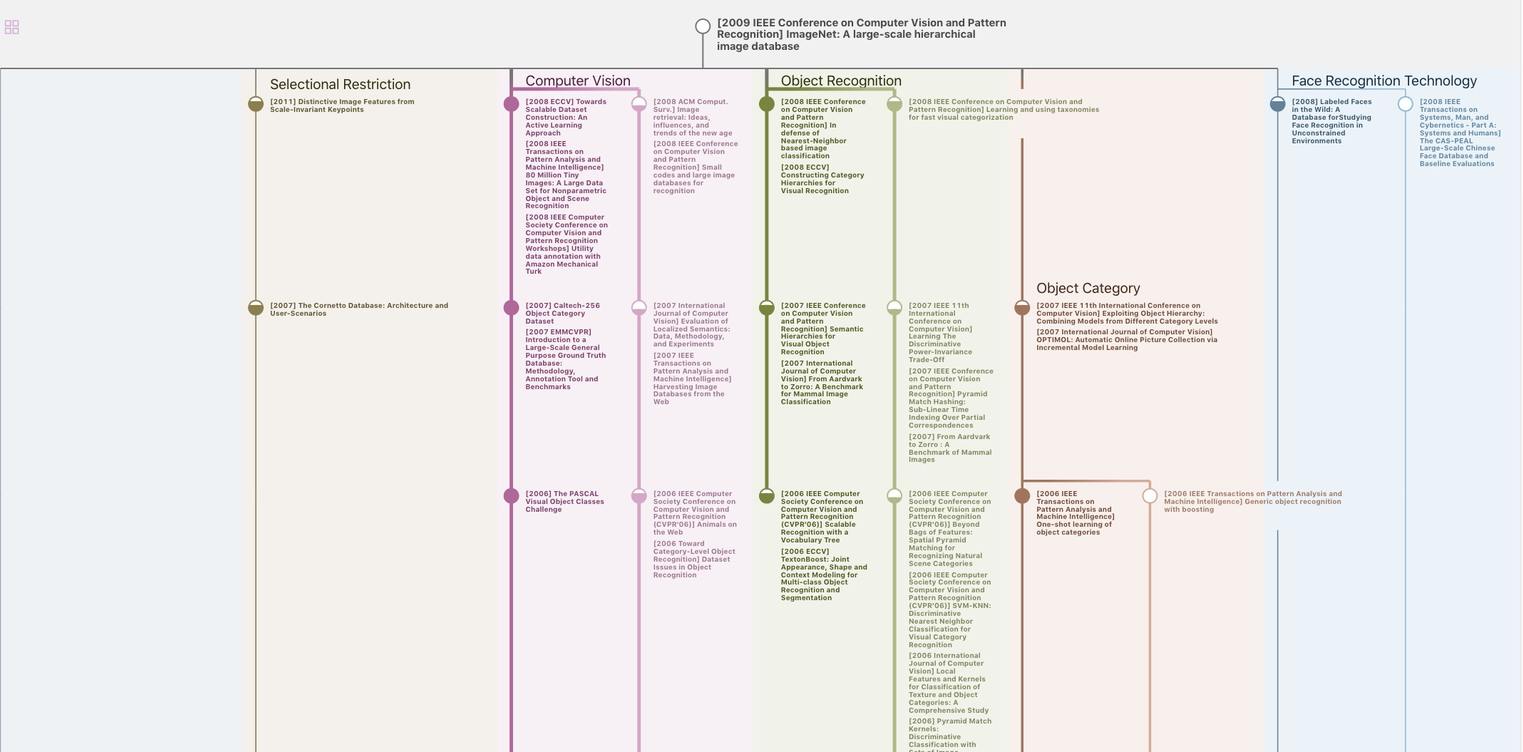
生成溯源树,研究论文发展脉络
Chat Paper
正在生成论文摘要