AAAN: Anomaly Alignment in Attributed Networks
Knowledge-Based Systems(2022)
摘要
Anomaly subgraph detection is an important problem that has been well researched in various applications, ranging from cyberattacks in computer networks to malicious activities in social networks. Most existing approaches detect anomaly subgraphs in attributed networks with sufficient features. However, multitudinous industry data with insufficient anomalous attributes are the main challenge for traditional anomaly subgraph detection algorithms. In particular, none of the literature focuses on connected anomaly subgraph detection with insufficient anomalous features. To address this problem, we propose Anomaly Alignment in Attributed Networks (AAAN), which first detects connected anomaly subgraphs by aligning anomalies in two attributed networks (one with insufficient anomalous features and the other with sufficient anomalous features). Extensive experiments on three real-world datasets (the Weibo, Baidu migration network, and COVID-19 pandemic datasets) show the effectiveness and efficiency of our algorithm. We can identify the crime hotspots in terms of city blocks from urban traffic networks, which are aligned with the criminal events reported on social networks. The results also demonstrate how AAAN outperforms competitive approaches in the COVID-19 outbreak anomaly subgraph detection and urban crime hotspot detection tasks.
更多查看译文
关键词
Anomaly subgraph detection,Network alignment
AI 理解论文
溯源树
样例
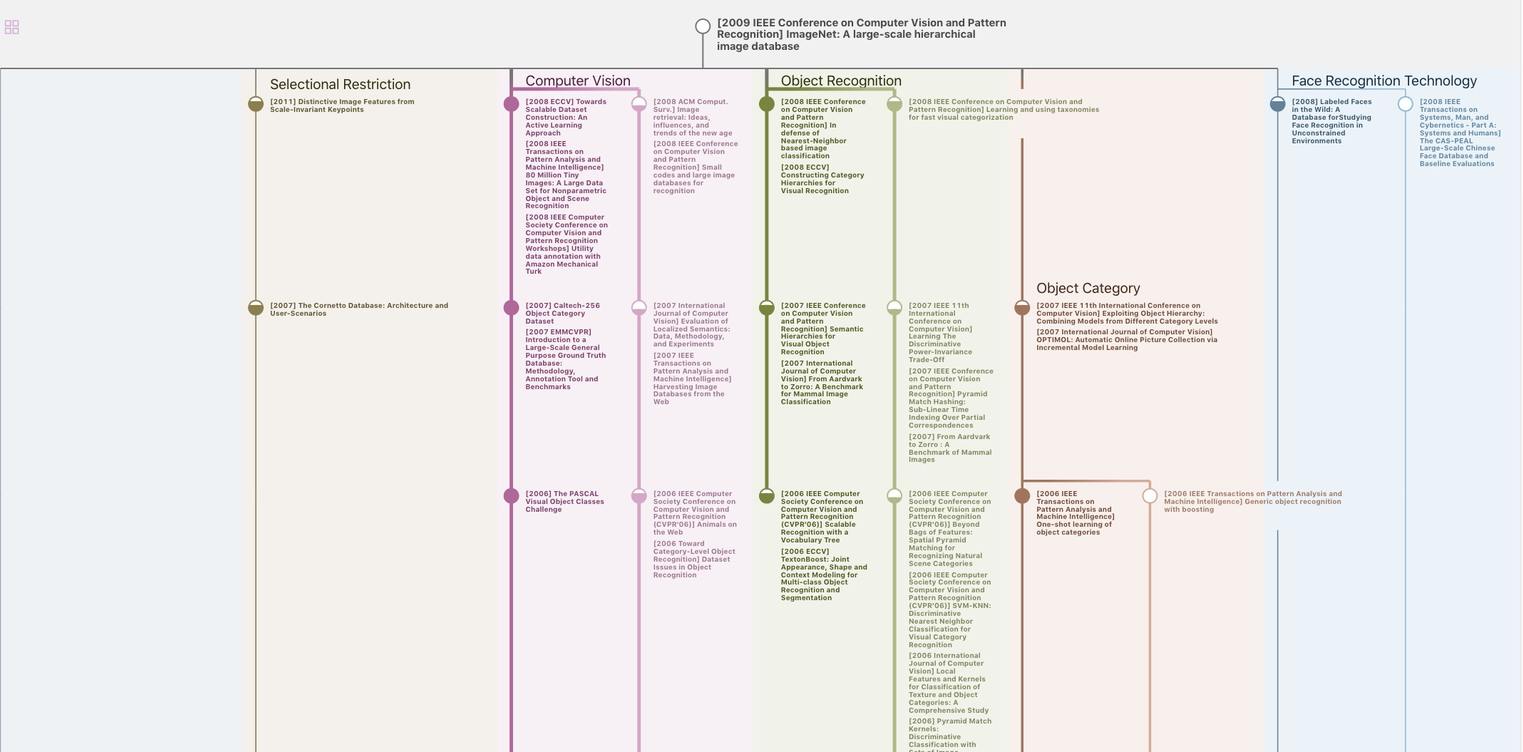
生成溯源树,研究论文发展脉络
Chat Paper
正在生成论文摘要