An assessment of multi-view spectral information from UAV-based color-infrared images for improved estimation of nitrogen nutrition status in winter wheat
Precision Agriculture(2022)
摘要
Rapid, accurate and non-destructive assessment of nutrition status is beneficial not only to the optimization of fertilization strategy in crop production, but also to the improvement of crop productivity. Unmanned aerial vehicle (UAV) remote sensing, as a new monitoring technology, has been widely used in nitrogen nutrition assessment because of its low cost, easy operation and flexibility in data acquisition with high temporal and spatial resolutions. However, most studies have only used mosaicked orthophotos obtained by UAV conventional survey to estimate crop parameters. Few studies have considered whether the multi-view information in UAV-based high-overlapping images is conducive to improving the estimation of crop nitrogen nutrition. To this end, this study evaluated the use of multi-view information from individual high-overlapping images and compared it with those of single-view information from a nadir-view image and a mosaicked orthophoto. A UAV-based color infrared camera (CIR) was employed to collect UAV images at critical growth stages of winter wheat. Three types of machine learning algorithms (support vector regression, SVR; extreme learning machine, ELM; random forest, RF) were used to compare and analyze the performance of these three methods for the estimation of leaf nitrogen concentration (LNC), plant nitrogen concentration (PNC), leaf nitrogen accumulation (LNA) and plant nitrogen accumulation (PNA). The results showed that the performance of the nadir-view image was the worst for the estimation of nitrogen nutrition parameters, while the mosaicked orthophoto yielded moderate estimation accuracy. High-overlapping multi-view images yielded the highest estimation accuracy of LNC and PNC (LNC: R 2 = 0.61, RMSE = 0.37%; PNC: R 2 = 0.52, RMSE = 0.24%). Similarly, the best predictive accuracy was obtained using UAV-based multi-view information with SVR for LNA ( R 2 = 0.72, RMSE = 1.45 g/m 2 ) and PNA ( R 2 = 0.62, RMSE = 3.02 g/m 2 ). This improvement was obtained with SVR but not with ELM and RF, suggesting that SVR performed the best for a small sample size. This study demonstrated that the multi-view information extracted from UAV-based high-overlapping images has great potential in improving the ability of crop growth monitoring.
更多查看译文
关键词
Unmanned aerial vehicle (UAV),High-overlapping images,Single-view images,Multi-view images Nitrogen status,Wheat
AI 理解论文
溯源树
样例
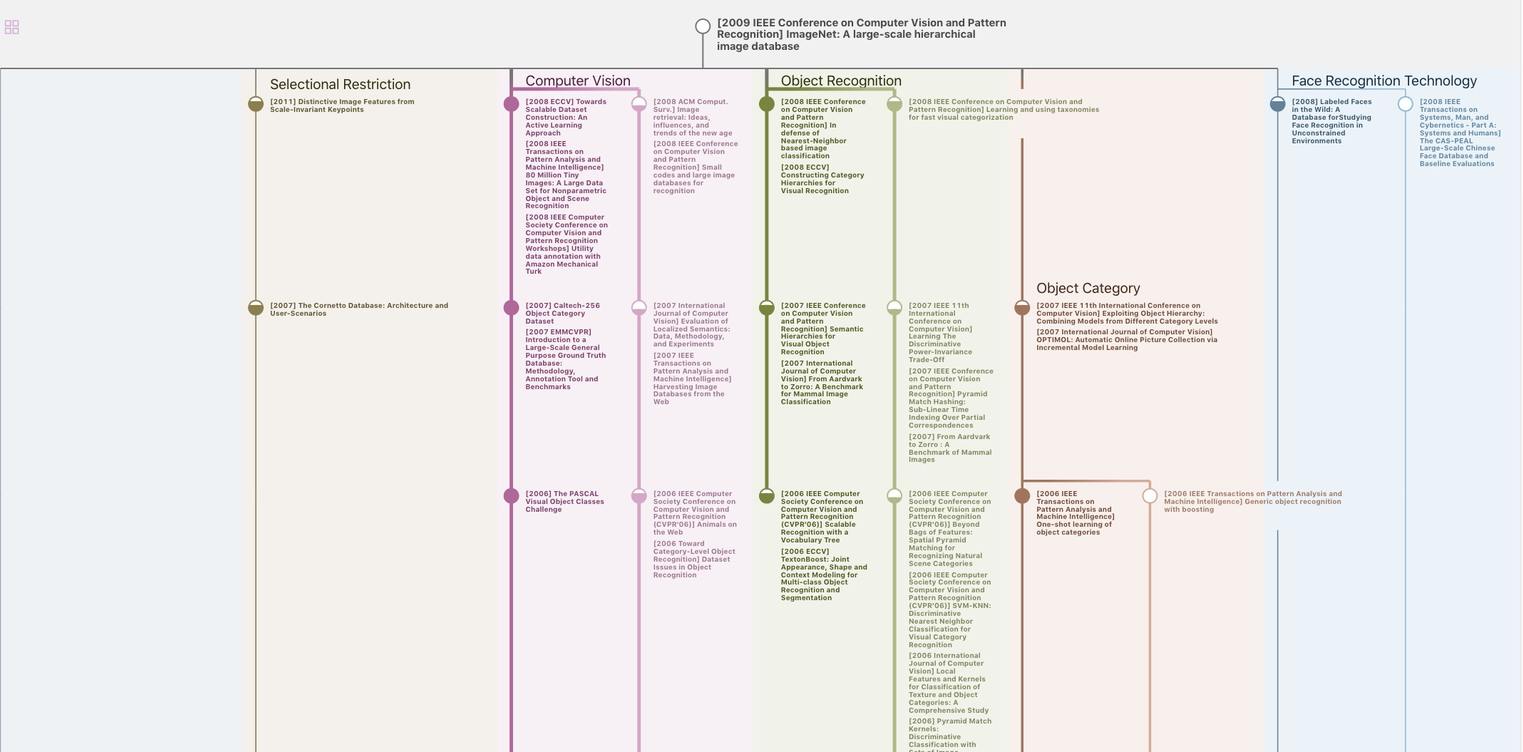
生成溯源树,研究论文发展脉络
Chat Paper
正在生成论文摘要