Machine Learning Based Non-Enhanced CT Radiomics for the Identification of Orbital Cavernous Venous Malformations: an Innovative Tool.
The Journal of craniofacial surgery/The journal of craniofacial surgery(2022)
摘要
PURPOSE:To evaluate the capability of non-enhanced computed tomography (CT) images for distinguishing between orbital cavernous venous malformations (OCVM) and non-OCVM, and to identify the optimal model from radiomics-based machine learning (ML) algorithms. METHODS:A total of 215 cases of OCVM and 120 cases of non- OCVM were retrospectively analyzed in this study. A stratified random sample of 268 patients (80%) was used as the training set (172 OCVM and 96 non-OCVM); the remaining data were used as the testing set. Six feature selection techniques and thirteen ML models were evaluated to construct an optimal classification model. RESULTS:There were statistically significant differences between the OCVM and non-OCVM groups in the density and tumor location (P < 0.05), whereas other indicators were comparable (age, gender, sharp, P > 0.05). Linear regression (area under the curve [AUC] = 0.9351; accuracy = 0.8657) and Stochastic Gradient Descent (AUC = 0.9448; accuracy = 0.8806) classifiers, both of which coupled with the f test and L1-based feature selection method, achieved optimal performance. The support vector machine (AUC = 0.9186; accuracy = 0.8806), Random Forest (AUC = 0.9288; accuracy = 0.8507) and eXtreme Gradient Boosting (AUC = 0.9147; accuracy = 0.8507) classifier combined with f test method showed excellent average performance among our study, respectively. CONCLUSIONS:The effect of non-enhanced CT images in OCVM not only can help ophthalmologist to find and locate lesion, but also bring great help for the qualitative diagnosis value using radiomic- based ML algorithms.
更多查看译文
关键词
Machine learning,non-enhanced computed tomography,orbital cavernous venous malformation,radiomics
AI 理解论文
溯源树
样例
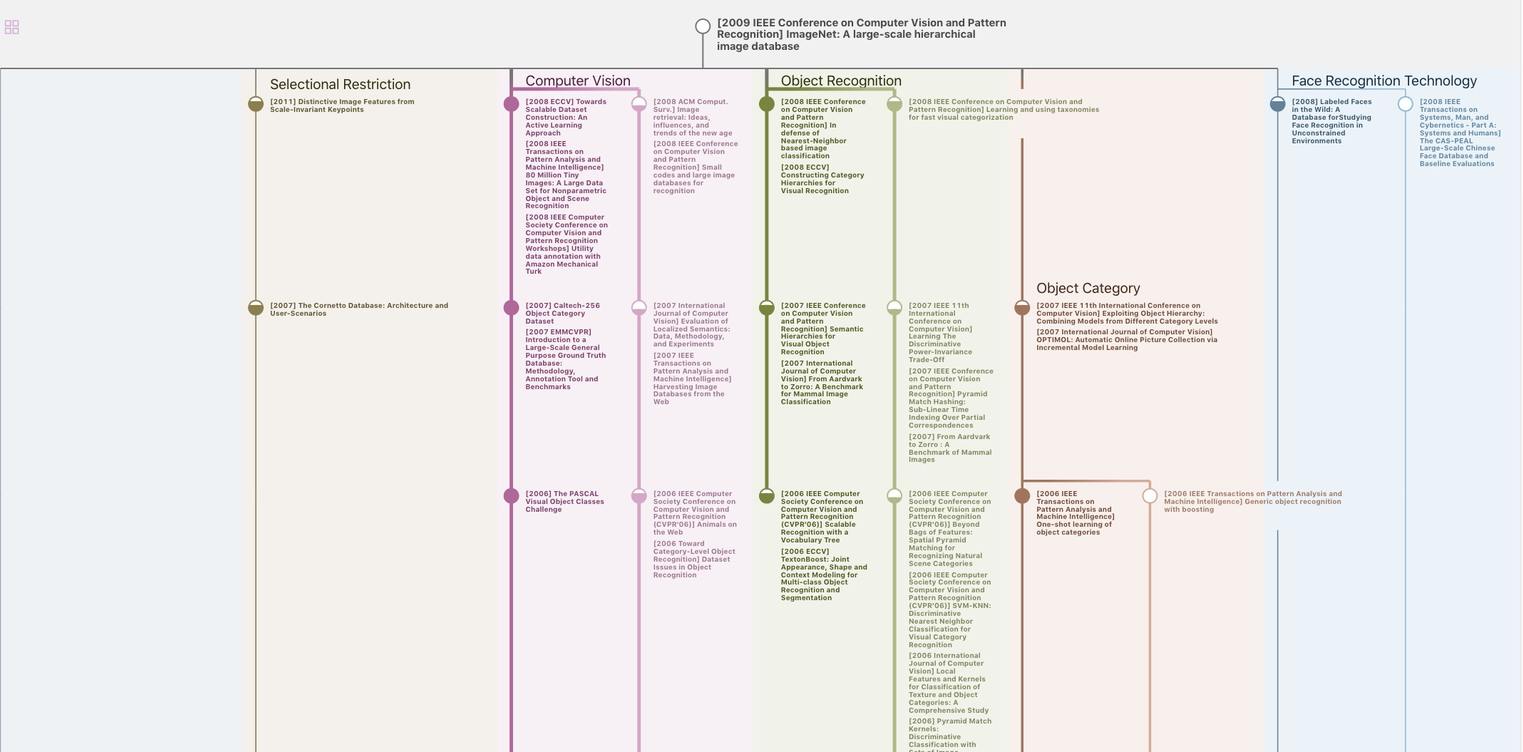
生成溯源树,研究论文发展脉络
Chat Paper
正在生成论文摘要