EBBE-Text: Explaining Neural Networks by Exploring Text Classification Decision Boundaries
IEEE transactions on visualization and computer graphics(2023)
摘要
While neural networks (NN) have been successfully applied to many NLP tasks, the way they function is often difficult to interpret. In this article, we focus on binary text classification via NNs and propose a new tool, which includes a visualization of the decision boundary and the distances of data elements to this boundary. This tool increases the interpretability of NN. Our approach uses two innovative views: (1) an overview of the text representation space and (2) a local view allowing data exploration around the decision boundary for various localities of this representation space. These views are integrated into a visual platform, EBBE-Text, which also contains state-of-the-art visualizations of NN representation spaces and several kinds of information obtained from the classification process. The various views are linked through numerous interactive functionalities that enable easy exploration of texts and classification results via the various complementary views. A user study shows the effectiveness of the visual encoding and a case study illustrates the benefits of using our tool for the analysis of the classifications obtained with several recent NNs and two datasets.
更多查看译文
关键词
Binary text classification,decision boundary,deep learning,interpretability,neural networks,representation space,visual analytics
AI 理解论文
溯源树
样例
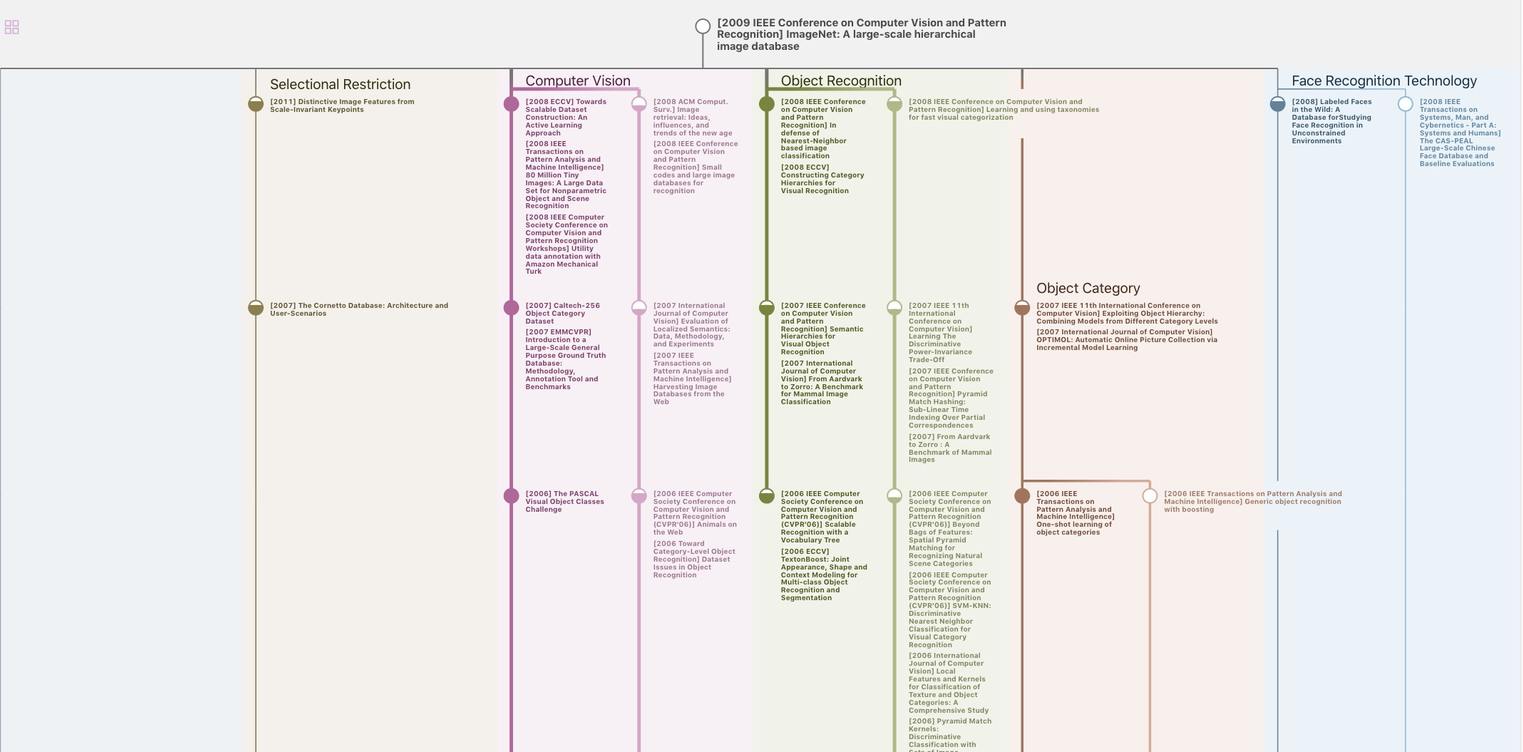
生成溯源树,研究论文发展脉络
Chat Paper
正在生成论文摘要