On Clustering for Cell-Phenotyping in Multiplex Immunohistochemistry (mihc) and Multiplexed Ion Beam Imaging (MIBI) Data
BMC Research Notes(2022)
摘要
Objective Multiplex immunohistochemistry (mIHC) and multiplexed ion beam imaging (MIBI) images are usually phenotyped using a manual thresholding process. The thresholding is prone to biases, especially when examining multiple images with high cellularity. Results Unsupervised cell-phenotyping methods including PhenoGraph, flowMeans, and SamSPECTRAL, primarily used in flow cytometry data, often perform poorly or need elaborate tuning to perform well in the context of mIHC and MIBI data. We show that, instead, semi-supervised cell clustering using Random Forests, linear and quadratic discriminant analysis are superior. We test the performance of the methods on two mIHC datasets from the University of Colorado School of Medicine and a publicly available MIBI dataset. Each dataset contains a bunch of highly complex images.
更多查看译文
关键词
Multiplex tissue imaging,Cell phenotyping,Vectra polaris,MIBI,Semi-supervised Learning
AI 理解论文
溯源树
样例
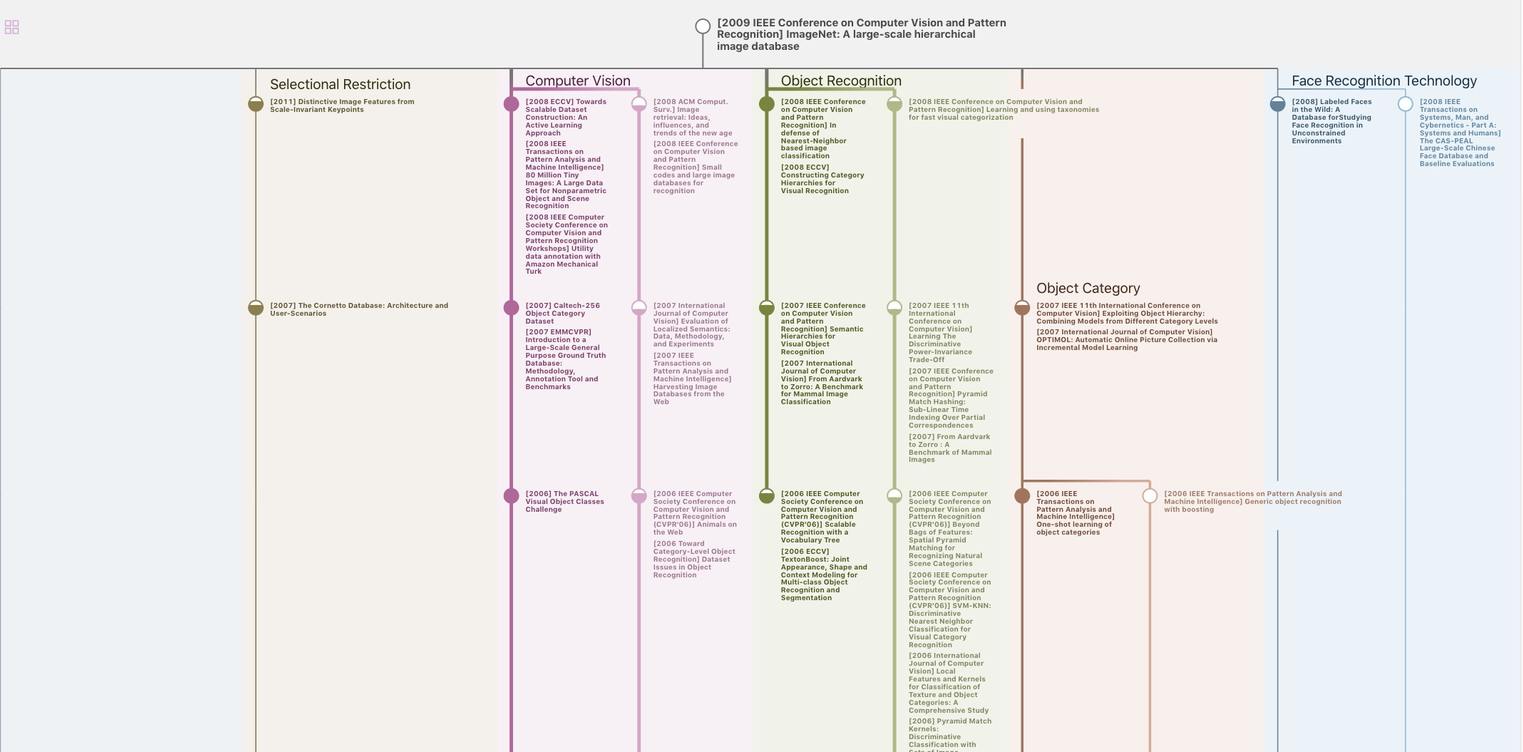
生成溯源树,研究论文发展脉络
Chat Paper
正在生成论文摘要