Using Machine Learning Algorithms to Enhance the Diagnostic Performance of Electrical Impedance Myography
Muscle & nerve(2022)
摘要
Introduction/Aims We assessed the classification performance of machine learning (ML) using multifrequency electrical impedance myography (EIM) values to improve upon diagnostic outcomes as compared to those based on a single EIM value. Methods EIM data was obtained from unilateral excised gastrocnemius in eighty diseased mice (26 D2-mdx, Duchenne muscular dystrophy model, 39 SOD1G93A ALS model, and 15 db/db, a model of obesity-induced muscle atrophy) and 33 wild-type (WT) animals. We assessed the classification performance of a ML random forest algorithm incorporating all the data (multifrequency resistance, reactance and phase values) comparing it to the 50 kHz phase value alone. Results ML outperformed the 50 kHz analysis as based on receiver-operating characteristic curves and measurement of the area under the curve (AUC). For example, comparing all diseases together versus WT from the test set outputs, the AUC was 0.52 for 50 kHz phase, but was 0.94 for the ML model. Similarly, when comparing ALS versus WT, the AUCs were 0.79 for 50 kHz phase and 0.99 for ML. Discussion Multifrequency EIM using ML improves upon classification compared to that achieved with a single-frequency value. ML approaches should be considered in all future basic and clinical diagnostic applications of EIM.
更多查看译文
关键词
amyotrophic lateral sclerosis,classification,electrical impedance,machine learning,muscle,muscular dystrophy
AI 理解论文
溯源树
样例
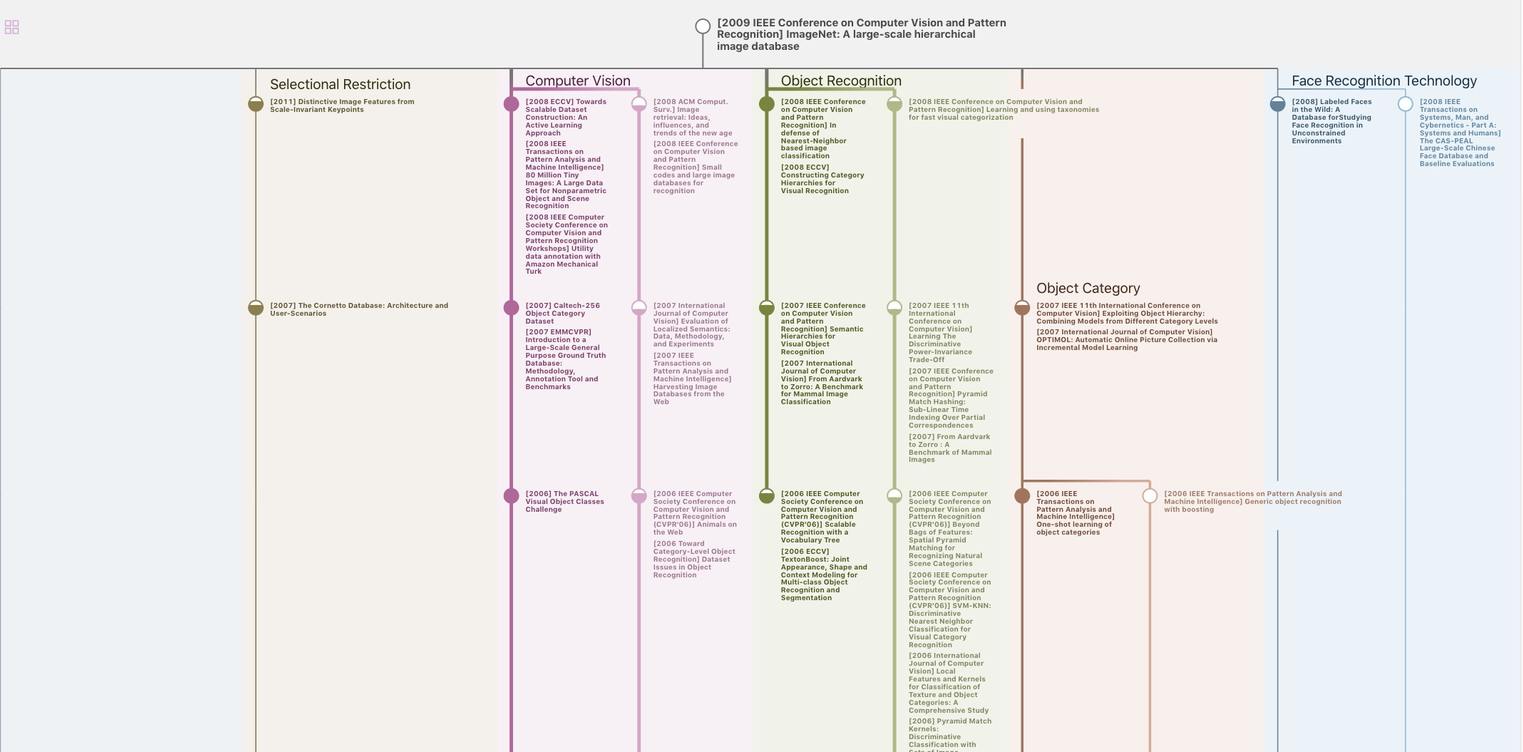
生成溯源树,研究论文发展脉络
Chat Paper
正在生成论文摘要