Mapping land-use intensity of grasslands in Germany with machine learning and Sentinel-2 time series
REMOTE SENSING OF ENVIRONMENT(2022)
摘要
Information on grassland land-use intensity (LUI) is crucial for understanding trends and dynamics in biodi-versity, ecosystem functioning, earth system science and environmental monitoring. LUI is a major driver for numerous environmental processes and indicators, such as primary production, nitrogen deposition and resil-ience to climate extremes. However, large extent, high resolution data on grassland LUI is rare. New satellite generations, such as Copernicus Sentinel-2, enable a spatially comprehensive detection of the mainly subtle changes induced by land-use intensification by their fine spatial and temporal resolution. We developed a methodology quantifying key parameters of grassland LUI such as grazing intensity, mowing frequency and fertiliser application across Germany using Convolutional Neural Networks (CNN) on Sentinel-2 satellite data with 20 m x 20 m spatial resolution. Subsequently, these land-use components were used to calculate a continuous LUI index. Predictions of LUI and its components were validated using comprehensive in situ grassland management data. A feature contribution analysis using Shapley values substantiates the applicability of the methodology by revealing a high relevance of springtime satellite observations and spectral bands related to vegetation health and structure. We achieved an overall classification accuracy of up to 66% for grazing in-tensity, 68% for mowing, 85% for fertilisation and an r2 of 0.82 for subsequently depicting LUI. We evaluated the methodology's robustness with a spatial 3-fold cross-validation by training and predicting on geographically distinctly separated regions. Spatial transferability was assessed by delineating the models' area of applicability. The presented methodology enables a high resolution, large extent mapping of land-use intensity of grasslands.
更多查看译文
关键词
Mowing,Grazing,Fertilisation,Convolutional Neural Networks,Random Forest,Classification,Deep learning,Optical satellite data
AI 理解论文
溯源树
样例
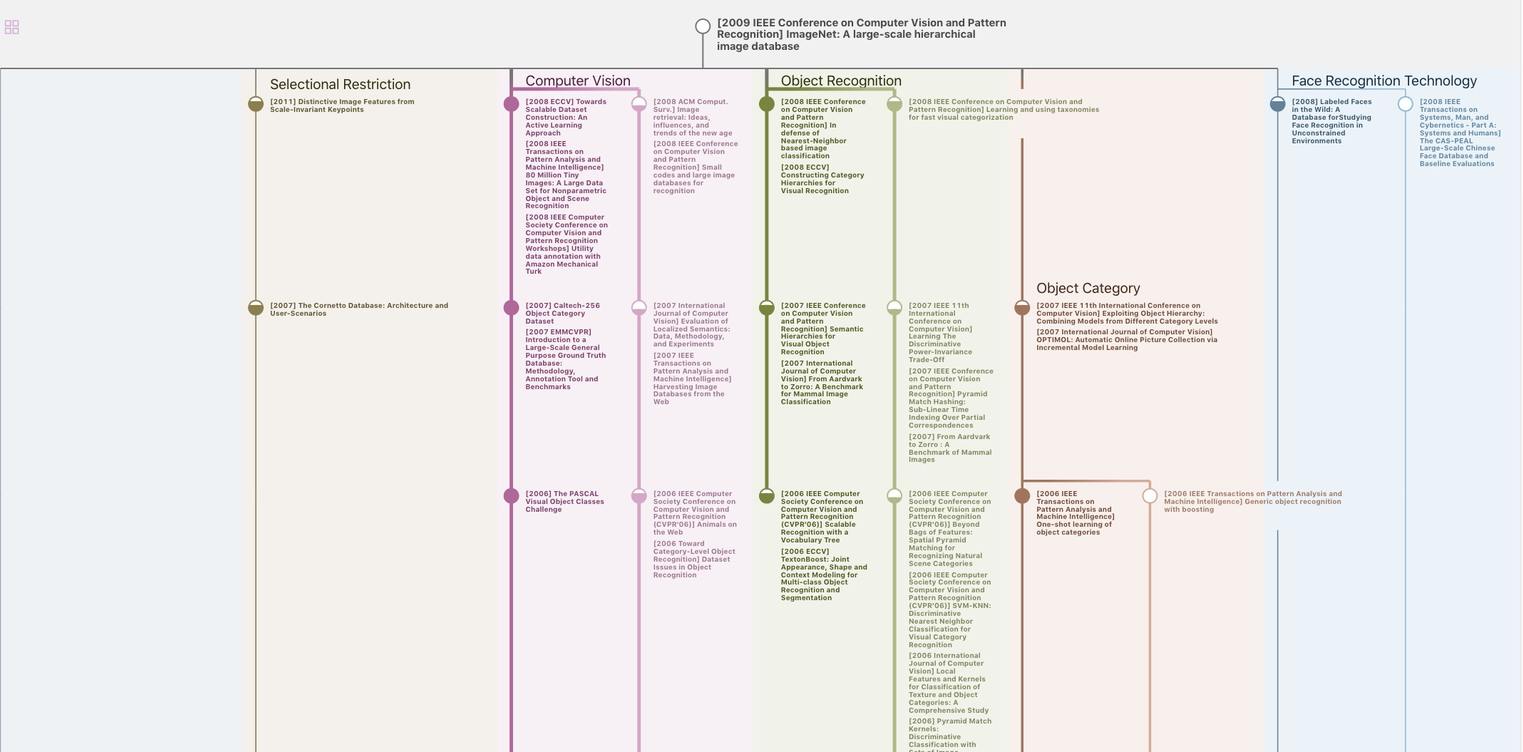
生成溯源树,研究论文发展脉络
Chat Paper
正在生成论文摘要