miRNA‐Based Cancer Classifier from TCGA Expression Profiles
The FASEB Journal(2022)
摘要
Introduction The Cancer Genome Atlas (TCGA) is a comprehensive multi-omics database of 33 different cancers, providing a wealth of clinical information and molecular datasets for biomarker discovery (Peng & Croce, 2016) and genomic pipeline development (Hutter & Zenklusen, 2018). MicroRNAs (miRNAs) are small non-coding regulatory RNAs that are excellent classificatory tissue markers due to their abundance and cell-type and disease-stage specificity (Gustafson et al., 2016); miRNA expression data are available from all 33 cancer types in the TCGA. Objectives and Hypothesis The primary objective of this study is to design and validate a miRNA-based classifier. We hypothesize that our machine learning algorithm will identify a set of miRNA biomarkers that can discriminate between the 33 types of cancer listed in the TCGA. Methods We compiled and preprocessed miRNA expression profiles for the 33 different cancers in TCGA. After preprocessing to remove duplicates, batch effects and technical outliers, unsupervised hierarchical clustering of miRNA expression profiles showed distinct separation of ovarian serous cystadenocarcinoma, glioblastoma multiforme and low-grade glioma, and testicular germ cell tumors from other cancer types (Figure 1). We observed certain cancer types with reproductive system origin had grouped together and apart from other cancer types during unsupervised clustering. Based on these observations and prior knowledge of tumor anatomy and pathology, we created a hierarchical classifier wherein each cancer type is systematically discriminated from the remaining cancer types, until each cancer is identifiable through process of elimination. At each step, a feature selection algorithm developed in our lab identified miRNA biomarkers that can classify these cancers based on organ system and subsequently, the specific cancer. Results After data preprocessing and filtering at the 90th percentile of expression, the data set included 8287 samples and 617 miRNAs. Feature selection analysis for classifier design effectively separates reproductive cancers from others. Conclusion We have developed a miRNA-based classifier for of ovarian serous cystadenocarcinoma and testicular germ cell tumors, both under reproductive system cancers. We will continue to expand the classifier for all 33 cancers in TCGA and finalize through validation with unlabeled samples. The resultant classifier for multiple cancers has clinical potential for sample diagnosis, but also provides insight into cancer diversity and pathogenesis across organ systems and subtypes. Gustafson, D., Tyryshkin, K., & Renwick, N. (2016). microRNA-guided diagnostics in clinical samples. Best Pract Res Clin Endocrinol Metab, 30(5), 563-575. https://doi.org/10.1016/j.beem.2016.07.002 Hutter, C., & Zenklusen, J. C. (2018). The Cancer Genome Atlas: Creating Lasting Value beyond Its Data. Cell, 173(2), 283-285. https://doi.org/10.1016/j.cell.2018.03.042 Peng, Y., & Croce, C. M. (2016). The role of MicroRNAs in human cancer. Signal Transduction and Targeted Therapy, 1(1), 15004. https://doi.org/10.1038/sigtrans.2015.4
更多查看译文
关键词
cancer classifier
AI 理解论文
溯源树
样例
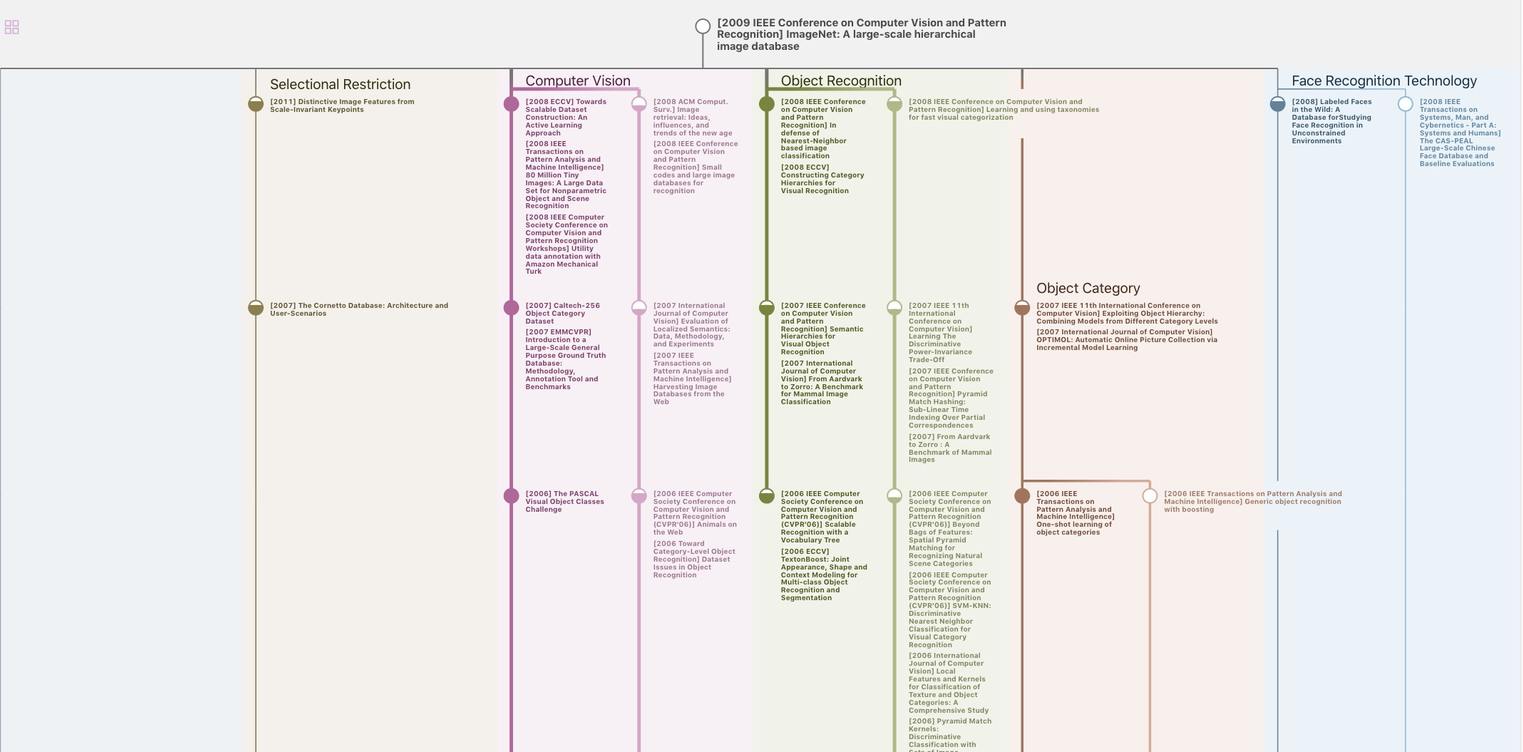
生成溯源树,研究论文发展脉络
Chat Paper
正在生成论文摘要