Establishment and Analysis of a Combined Diagnostic Model of Liver Cancer with Random Forest and Artificial Neural Network
Mathematical Problems in Engineering(2022)
摘要
The incidence of liver cancer (hepatocellular carcinoma; HCC) is rising and with poor clinical outcome expected, a more accurate judgment of tumor tissues and adjacent nontumor tissues is necessary. The aim of this study was to construct a diagnostic model based on random forest (RF) and artificial neural network (ANN). It can be used to aid in the identification of diseased tissue such as cancerous tissue, for HCC clinical diagnosis and surgical guidance. GSE36376 and GSE121248 from Gene Expression Omnibus (GEO) were used as training sets in this investigation. R package “limma” and WGCNA were used to filter the training set for statistically significant differential genes. To better understand the biological function and characteristics, R software was used to perform GO and KEGG enrichment analyses. To pick out and further understand the key genes, we performed PPI analysis and random forest tree analysis. Next, we built the ANN to predict training sets and validation set (GSE84402), and ROC curve was plotted to calculate area under curve (AUC). Then immune cell infiltration indicated difference of immune cell subsets between control and case groups. Finally, the survival analysis of key genes was also carried out based on data in TCGA database. Based on the expression of these 9 genes, we built the artificial neural network (ANN) and the accuracy of the final models was assessed with an ROC curve. The areas under the ROC curve were 0.984 (95% CI 0.972–0.993) in training sets. Its predictive capability was further assessed using the validation set. And the areas under the ROC curve were 0.929 (95% CI 0.786–1.000). In summary, this method effectively classifies hepatocellular carcinoma tissues and the corresponding noncancerous tissues and provides reasonable new ideas for the early diagnosis of liver cancer in the future.
更多查看译文
AI 理解论文
溯源树
样例
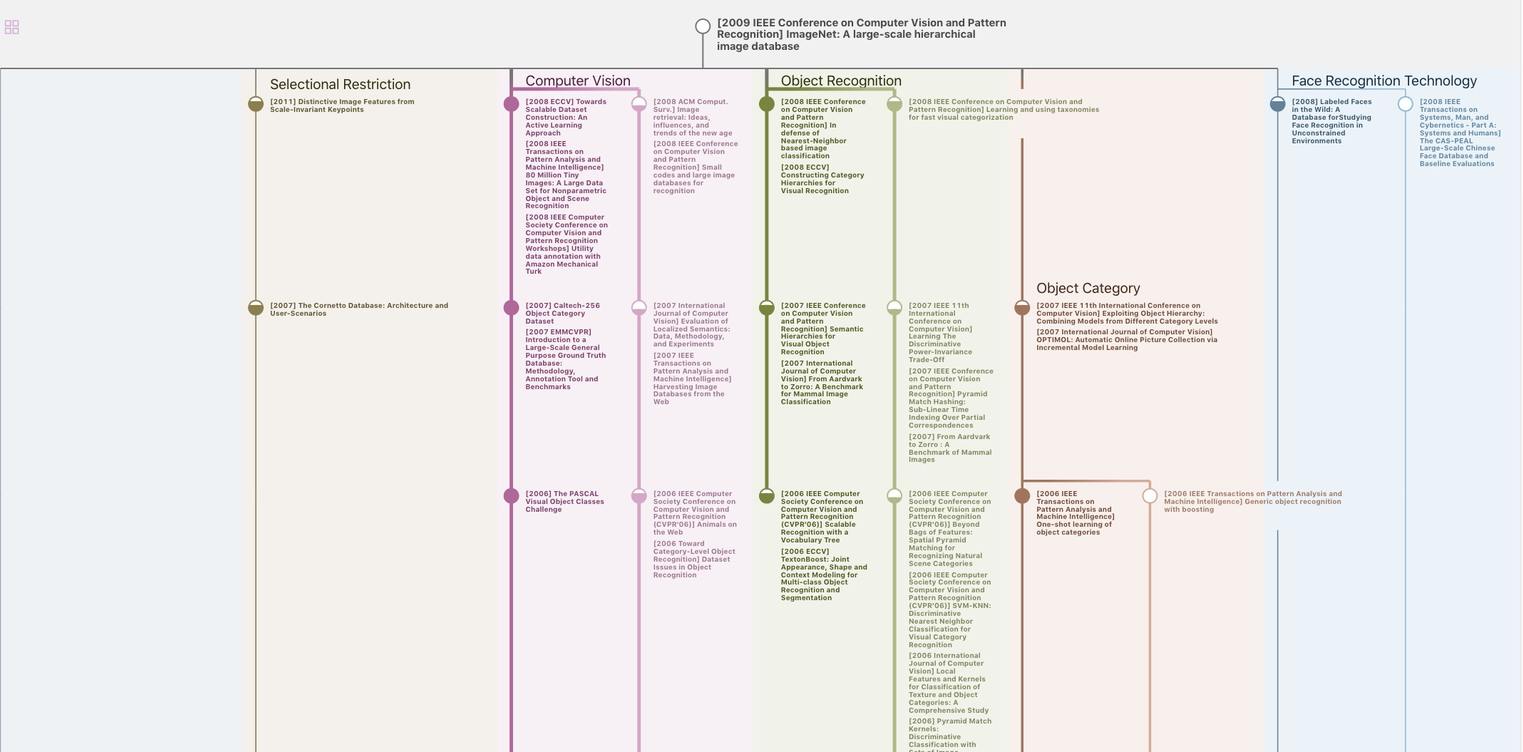
生成溯源树,研究论文发展脉络
Chat Paper
正在生成论文摘要