Data-Driven Signal Detection for Underwater Acoustic Filter Bank Multicarrier Communications
WIRELESS COMMUNICATIONS & MOBILE COMPUTING(2022)
摘要
By contraposing the signal detection for filter bank multicarrier (FBMC) communications with the underwater acoustic (UWA) channel, this paper analyzes the traditional imaginary interference problem and proposes a deep learning-based method. The neural network with feature extraction and automatic learning ability is employed to replace the demodulation modules to recover transmitted signals without explicit channel estimation and equalization. Sufficient data sets are generated according to the measured channel conditions in Qingjiang river, the optimization of network parameters is finished by constraining cost function in offline training, and the signal detection is carried out directly with the well-trained network in online testing. The system performance of various supervised learning models such as multilayer perceptron (MLP), convolutional neural network (CNN), and bidirectional long short-term memory (BLSTM) network is compared under different data sizes, network parameters, and prototype filters. The simulation results show that the bit error rate (BER) performance of the proposed signal detection is better than that of the classic one, which indicates that deep learning is a promising tool in UWA communication systems.
更多查看译文
AI 理解论文
溯源树
样例
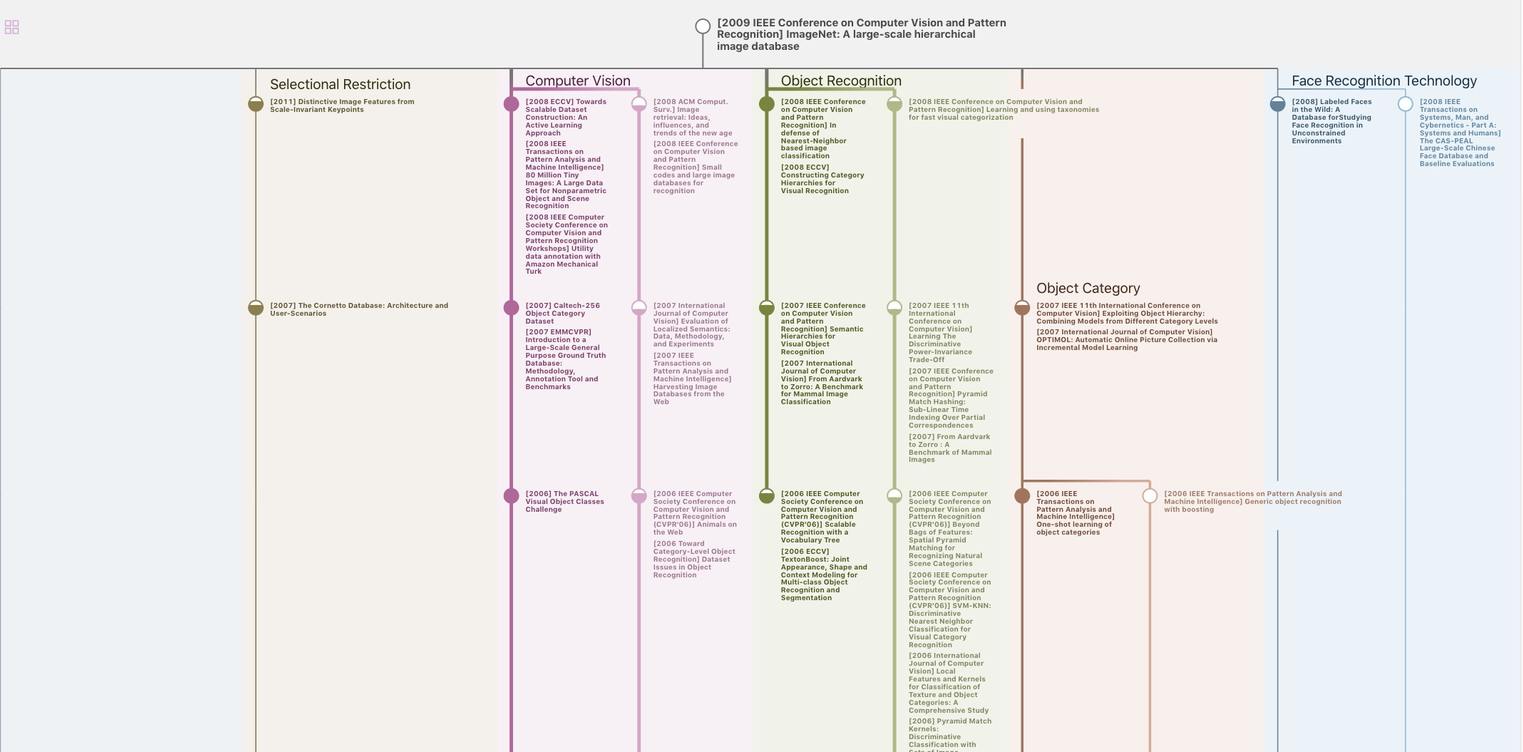
生成溯源树,研究论文发展脉络
Chat Paper
正在生成论文摘要