A Sparse Non-parametric BRDF Model.
ACM Trans. Graph.(2022)
摘要
This paper presents a novel sparse non-parametric BRDF model derived using a machine learning approach to represent the space of possible BRDFs using a set of multidimensional sub-spaces, or dictionaries. By training the dictionaries under a sparsity constraint, the model guarantees high quality representations with minimal storage requirements and an inherent clustering of the BDRF-space. The model can be trained once and then reused to represent a wide variety of measured BRDFs. Moreover, the proposed method is flexible to incorporate new unobserved data sets, parameterizations, and transformations. In addition, we show that any two, or more, BRDFs can be smoothly interpolated in the coefficient space of the model rather than the significantly higher-dimensional BRDF space. The proposed sparse BRDF model is evaluated using the MERL, DTU and RGL-EPFL BRDF databases. Experimental results show that the proposed approach results in about 9.75dB higher SNR on average for rendered images as compared to current state-of-the-art models.
更多查看译文
关键词
Rendering,reflectance and shading models,machine learning,dictionary learning,non-parametric BRDF model,BRDF interpolation
AI 理解论文
溯源树
样例
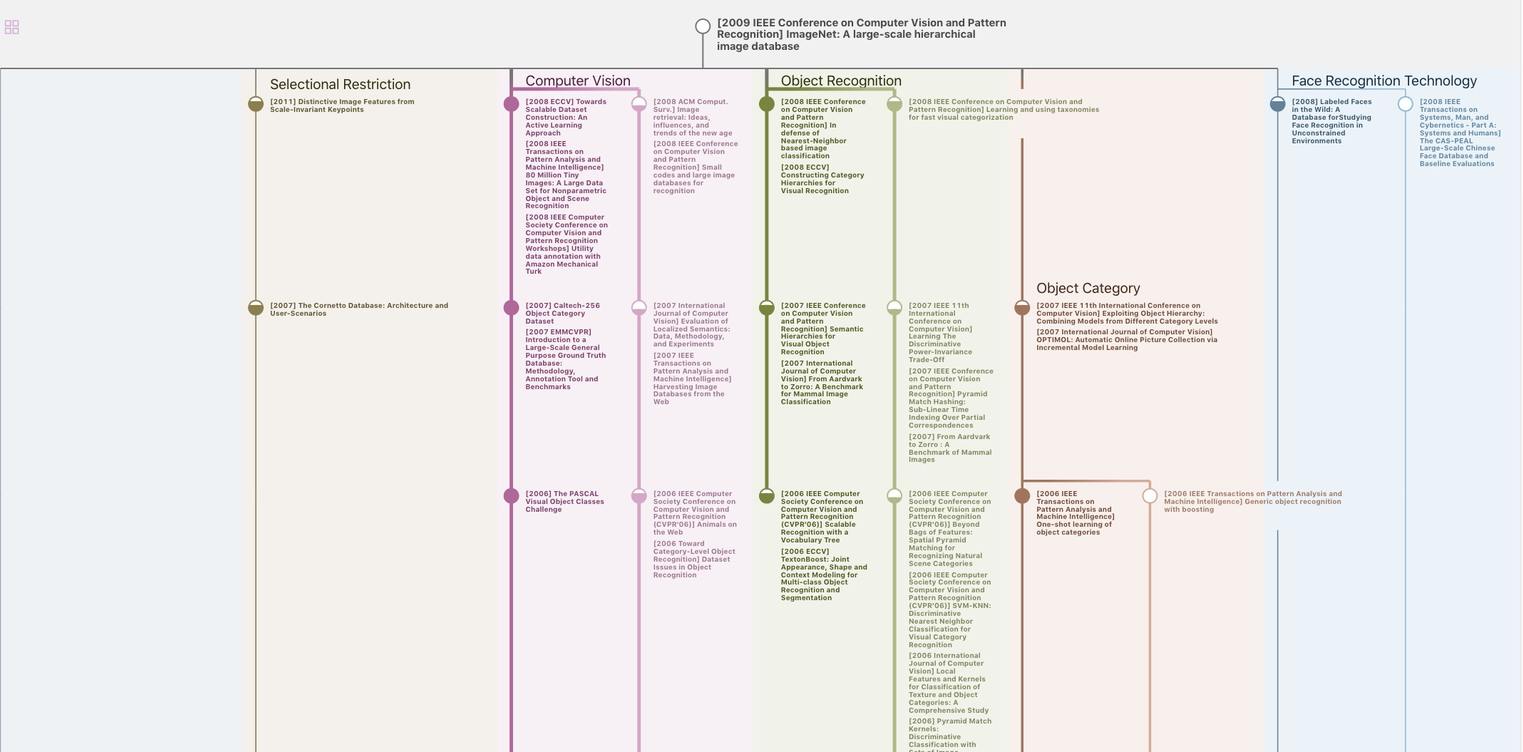
生成溯源树,研究论文发展脉络
Chat Paper
正在生成论文摘要