SCIM: Simultaneous Clustering, Inference, and Mapping for Open-World Semantic Scene Understanding
arxiv(2022)
摘要
In order to operate in human environments, a robot's semantic perception has to overcome open-world challenges such as novel objects and domain gaps. Autonomous deployment to such environments therefore requires robots to update their knowledge and learn without supervision. We investigate how a robot can autonomously discover novel semantic classes and improve accuracy on known classes when exploring an unknown environment. To this end, we develop a general framework for mapping and clustering that we then use to generate a self-supervised learning signal to update a semantic segmentation model. In particular, we show how clustering parameters can be optimized during deployment and that fusion of multiple observation modalities improves novel object discovery compared to prior work. Models, data, and implementations can be found at https://github.com/hermannsblum/scim
更多查看译文
关键词
Self-supervised learning, Semantic segmentation, Self-improving perception, Semantic scene understanding
AI 理解论文
溯源树
样例
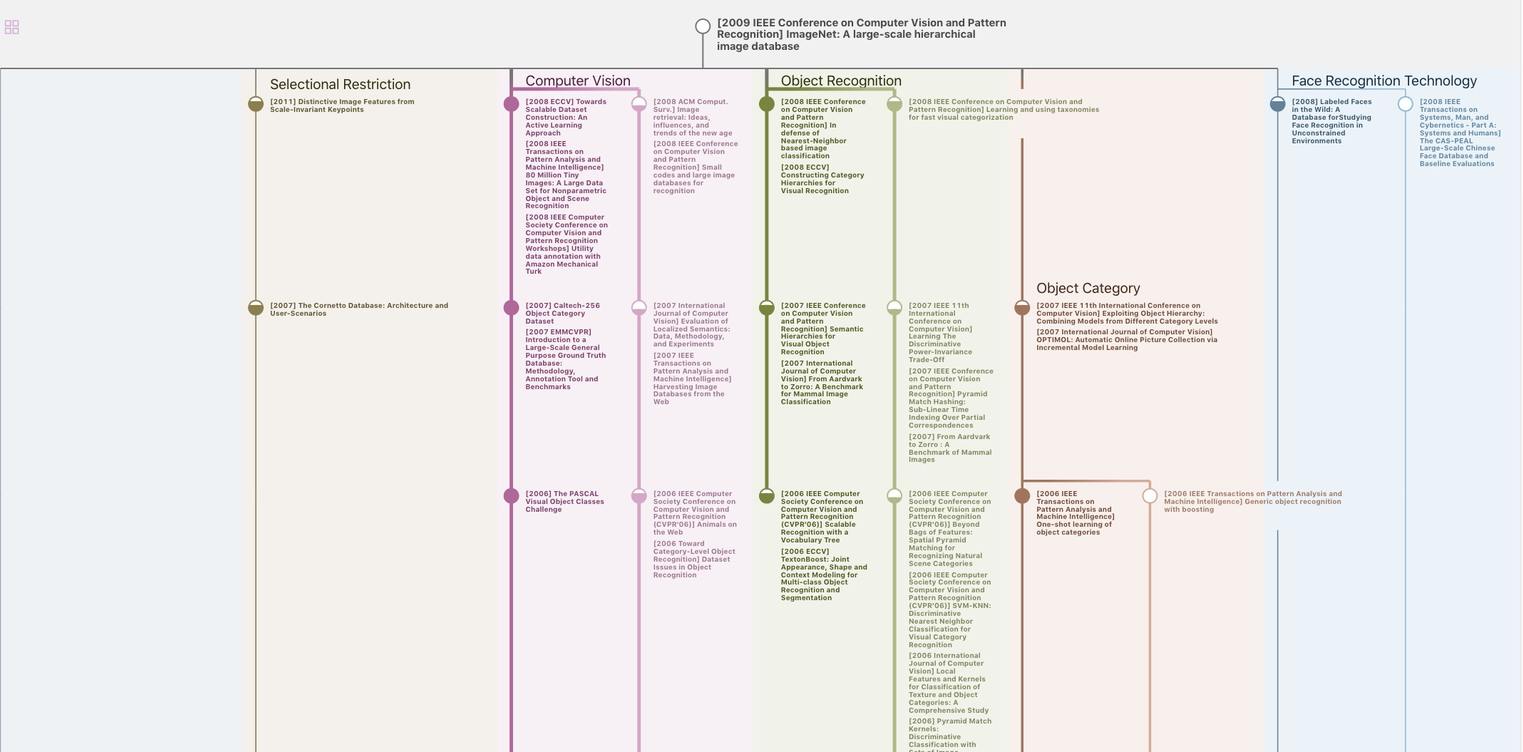
生成溯源树,研究论文发展脉络
Chat Paper
正在生成论文摘要