TiCo: Transformation Invariance and Covariance Contrast for Self-Supervised Visual Representation Learning
arxiv(2022)
摘要
We present Transformation Invariance and Covariance Contrast (TiCo) for self-supervised visual representation learning. Similar to other recent self-supervised learning methods, our method is based on maximizing the agreement among embeddings of different distorted versions of the same image, which pushes the encoder to produce transformation invariant representations. To avoid the trivial solution where the encoder generates constant vectors, we regularize the covariance matrix of the embeddings from different images by penalizing low rank solutions. By jointly minimizing the transformation invariance loss and covariance contrast loss, we get an encoder that is able to produce useful representations for downstream tasks. We analyze our method and show that it can be viewed as a variant of MoCo with an implicit memory bank of unlimited size at no extra memory cost. This makes our method perform better than alternative methods when using small batch sizes. TiCo can also be seen as a modification of Barlow Twins. By connecting the contrastive and redundancy-reduction methods together, TiCo gives us new insights into how joint embedding methods work.
更多查看译文
关键词
visual representation learning,representation learning,covariance contrast,transformation invariance,self-supervised
AI 理解论文
溯源树
样例
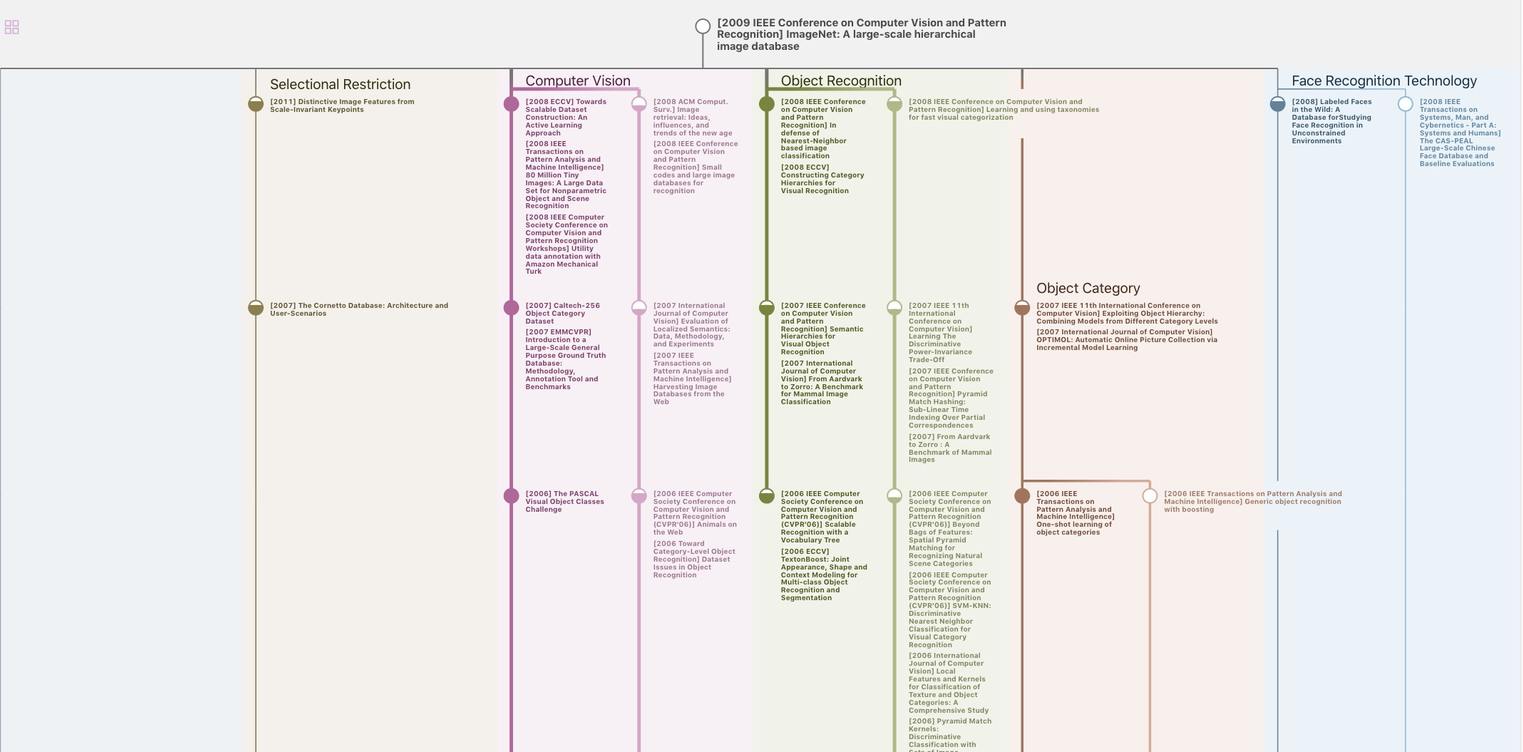
生成溯源树,研究论文发展脉络
Chat Paper
正在生成论文摘要