Determination of uncertainties in parton densities
PHYSICAL REVIEW D(2022)
摘要
We review various methods used to estimate uncertainties in quantum correlation functions, such as parton distribution functions (PDFs). Using a toy model of a PDF, we compare the uncertainty estimates yielded by the traditional Hessian and data resampling methods, as well as from explicitly Bayesian analyses using nested sampling or hybrid Markov chain Monte Carlo techniques. We investigate how uncertainty bands derived from neural network approaches depend on details of the network training, and how they compare to the uncertainties obtained from more traditional methods with a specific underlying parametrization. Our results show that utilizing a neural network on a simplified example of PDF data has the potential to inflate uncertainties, in part due to the cross-validation procedure that is generally used to avoid overfitting data.
更多查看译文
AI 理解论文
溯源树
样例
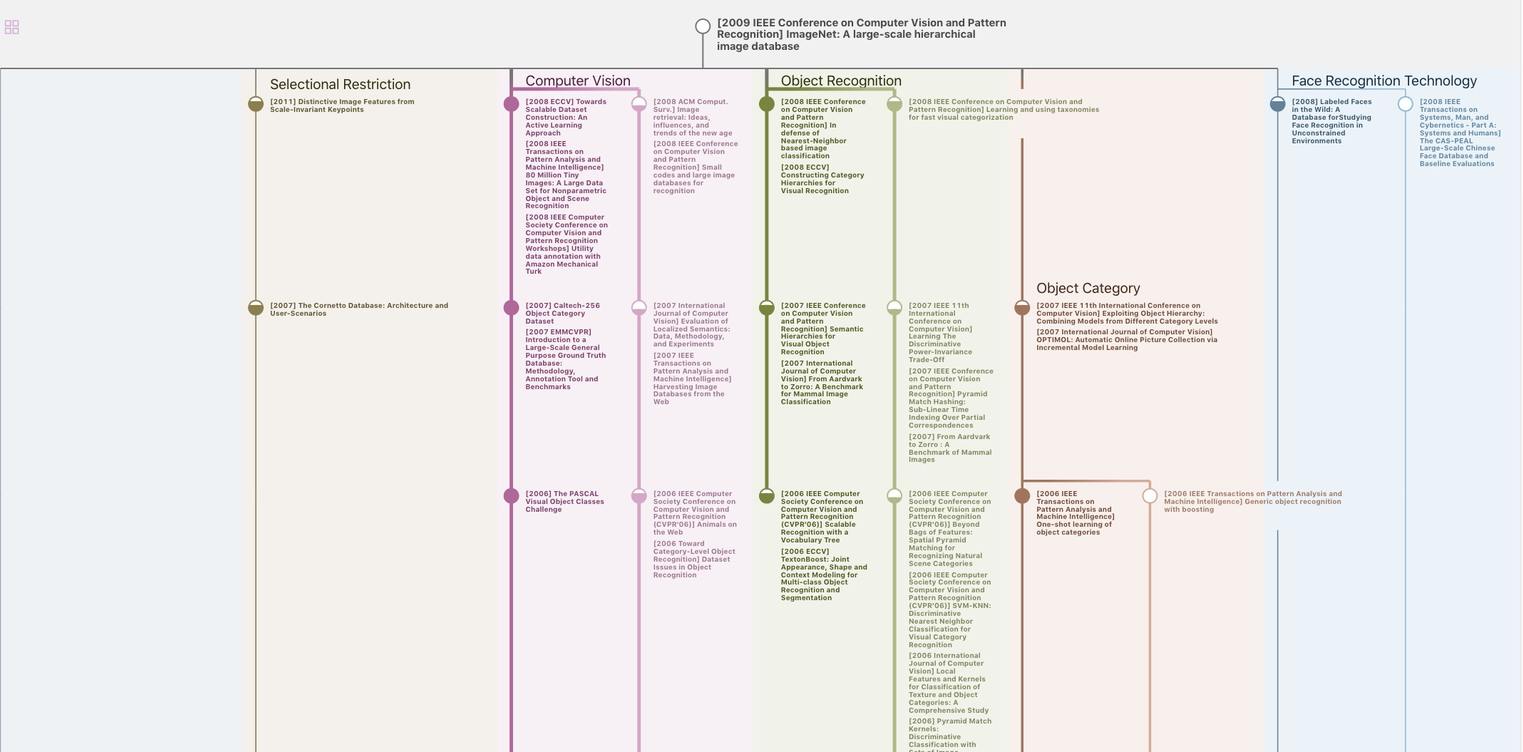
生成溯源树,研究论文发展脉络
Chat Paper
正在生成论文摘要