Open Vocabulary Object Detection with Proposal Mining and Prediction Equalization
arxiv(2022)
摘要
Open-vocabulary object detection (OVD) aims to scale up vocabulary size to detect objects of novel categories beyond the training vocabulary. Recent work resorts to the rich knowledge in pre-trained vision-language models. However, existing methods are ineffective in proposal-level vision-language alignment. Meanwhile, the models usually suffer from confidence bias toward base categories and perform worse on novel ones. To overcome the challenges, we present MEDet, a novel and effective OVD framework with proposal mining and prediction equalization. First, we design an online proposal mining to refine the inherited vision-semantic knowledge from coarse to fine, allowing for proposal-level detection-oriented feature alignment. Second, based on causal inference theory, we introduce a class-wise backdoor adjustment to reinforce the predictions on novel categories to improve the overall OVD performance. Extensive experiments on COCO and LVIS benchmarks verify the superiority of MEDet over the competing approaches in detecting objects of novel categories, e.g., 32.6% AP50 on COCO and 22.4% mask mAP on LVIS.
更多查看译文
关键词
proposal mining,detection,object
AI 理解论文
溯源树
样例
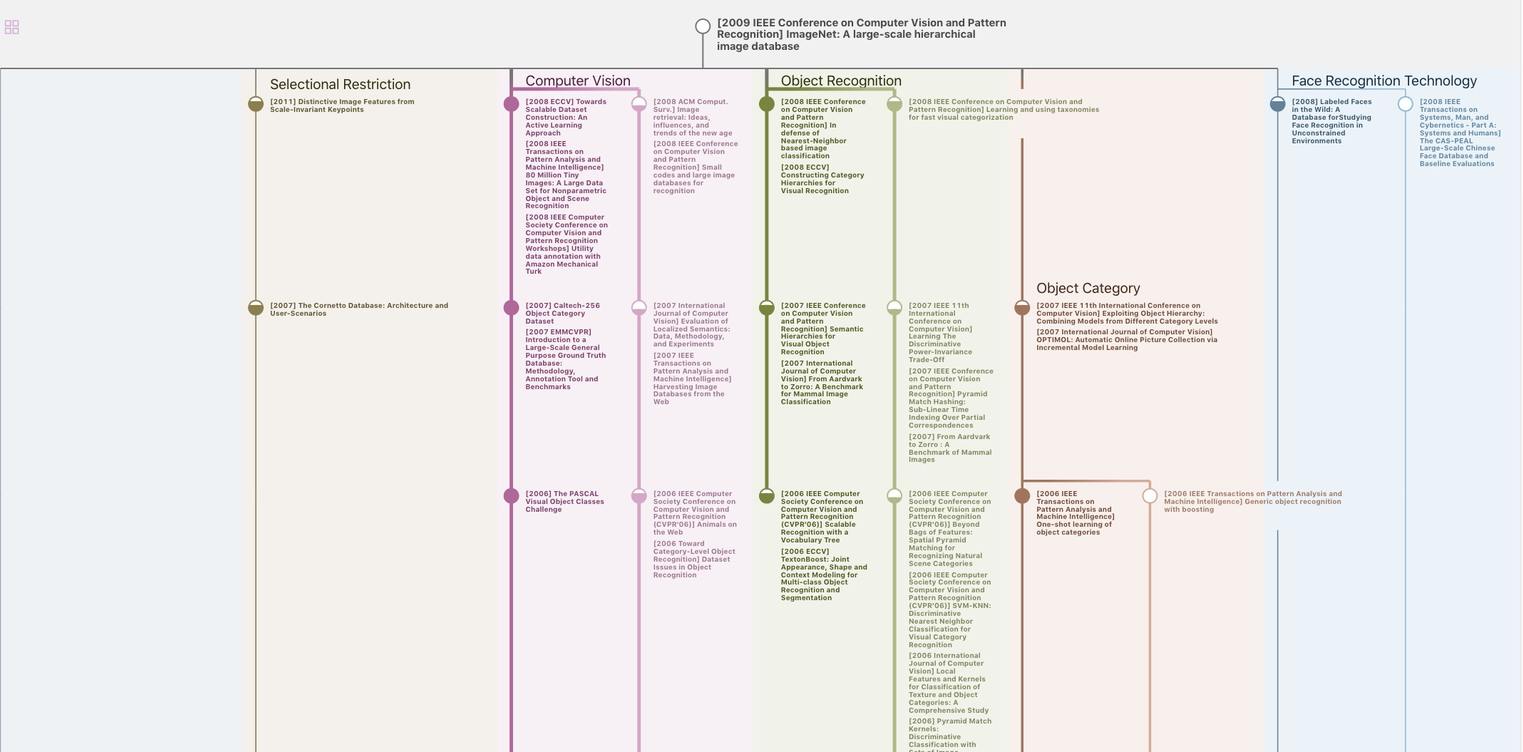
生成溯源树,研究论文发展脉络
Chat Paper
正在生成论文摘要