Face anti-spoofing via conditional adversarial domain generalization
Journal of Ambient Intelligence and Humanized Computing(2023)
摘要
Many currently existing face anti-spoofing methods do not generalize well to new scenarios due to the changes of background, light, and other factors. To tackle this problem, a face anti-spoofing model based on conditional adversarial domain generalization is proposed in this paper. The model tries to alleviate the discrepancy between source and target domains through the adversarial training of a generator and a domain discriminator. The domain discriminator uses the joint variables generated by multilinear mapping of the features and the classifier predictions as input data. The multiplicative interaction of the input data can promote the domain adversarial model to align multiple domains at the feature and class level, and form a feature space shared by the multiple domains. Besides, the domain discriminator uses the entropy criterion to adjust the priority of samples to reduce the adverse effects of difficult-to-transfer samples with the inaccurate prediction on domain generalization. The generator of the adversarial network consists of attention-Unet and ResNet-18 architectures, where the Unet embedded with the attention mechanism can extract more richer multi-scale domain shared features. The following supervised auxiliary classifier further amplifies the distinguishing features between classes. During the training phase, the model introduces an asymmetric triplet loss in order to get a clearer classification boundary, and introduces a face depth loss to enhance scenario-invariant. Comparative experiments on four public datasets and a custom dataset verify the feasibility of our model. The code is available at https://github.com/17863205785/CADG-master .
更多查看译文
关键词
Face anti-spoofing,Conditional adversarial domain generalization,Multi-linear map,Entropy criterion,Face depth
AI 理解论文
溯源树
样例
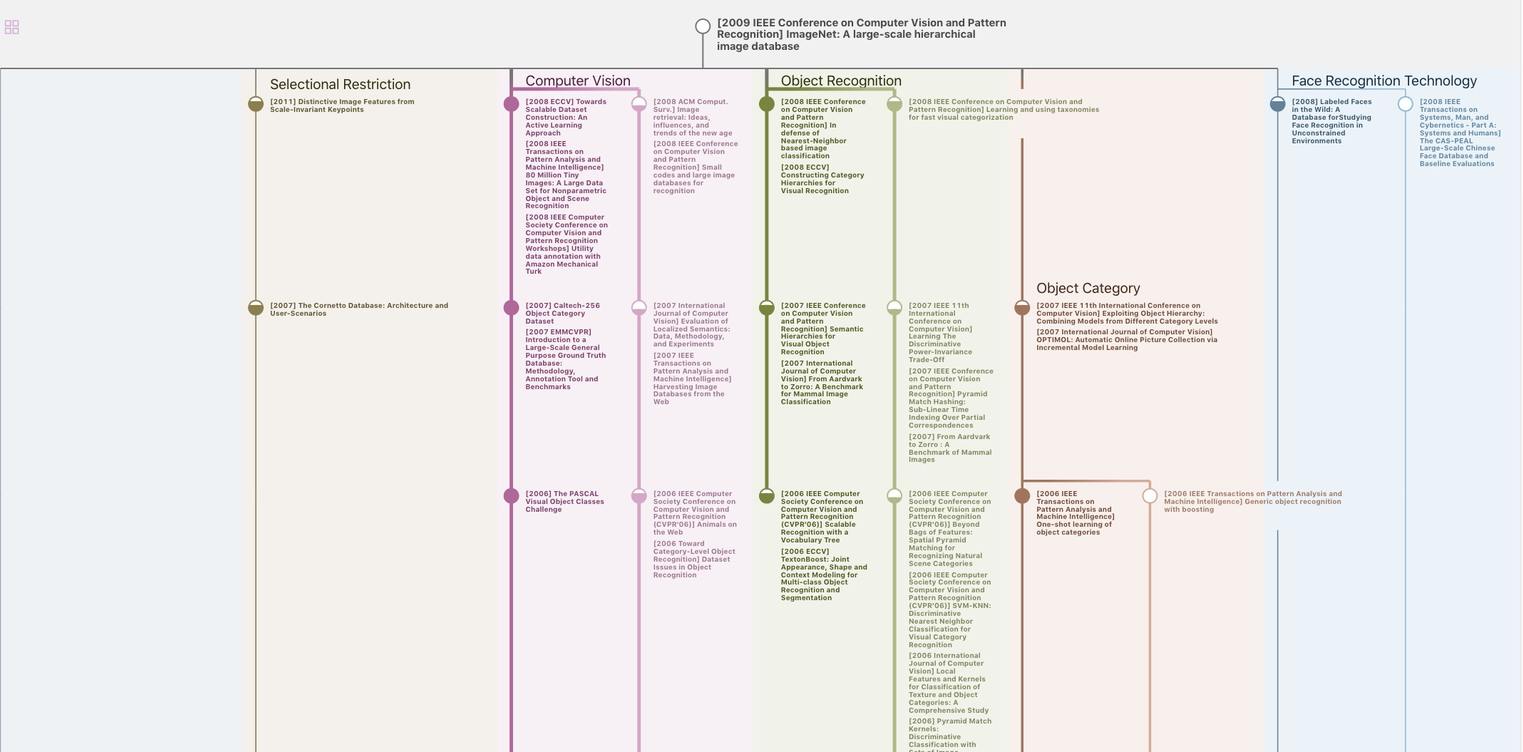
生成溯源树,研究论文发展脉络
Chat Paper
正在生成论文摘要