Evaluation of graph convolutional networks performance for visual question answering on reasoning datasets
MULTIMEDIA TOOLS AND APPLICATIONS(2022)
摘要
In the recent era, graph neural networks are widely used on vision-to-language tasks and achieved promising results. In particular, graph convolution network (GCN) is capable of capturing spatial and semantic relationships needed for visual question answering (VQA). But, applying GCN on VQA datasets with different subtasks can lead to varying results. Also, the training and testing size, evaluation metrics and hyperparameter used are other factors that affect VQA results. These, factors can be subjected into similar evaluation schemes in order to obtain fair evaluations of GCN based result for VQA. This study proposed a GCN framework for VQA based on fine tune word representation to solve handle reasoning type questions. The framework performance is evaluated using various performance measures. The results obtained from GQA and VQA 2.0 datasets slightly outperform most existing methods.
更多查看译文
关键词
VQA,GCN,Performance measure,Fine-tuned representation,Reasoning datasets
AI 理解论文
溯源树
样例
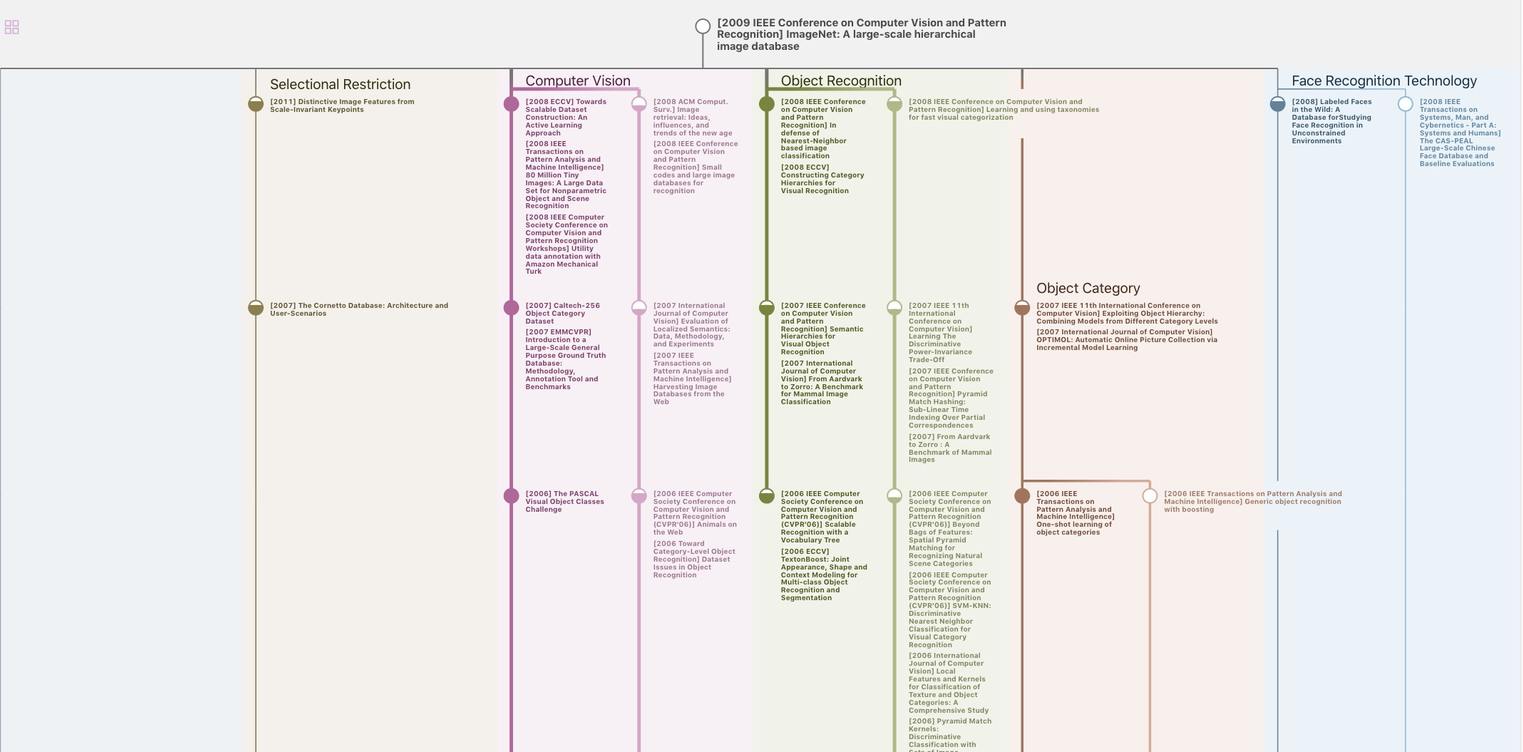
生成溯源树,研究论文发展脉络
Chat Paper
正在生成论文摘要