BestOf: an online implementation selector for the training and inference of deep neural networks
JOURNAL OF SUPERCOMPUTING(2022)
摘要
Tuning and optimising the operations executed in deep learning frameworks is a fundamental task in accelerating the processing of deep neural networks (DNNs). However, this optimisation usually requires extensive manual efforts in order to obtain the best performance for each combination of tensor input size, layer type, and hardware platform. In this work, we present BestOf, a novel online auto-tuner that optimises the training and inference phases of DNNs. BestOf automatically selects at run time, and among the provided alternatives, the best performing implementation in each layer according to gathered profiling data. The evaluation of BestOf is performed on multi-core architectures for different DNNs using PyDTNN, a lightweight library for distributed training and inference. The experimental results reveal that the BestOf auto-tuner delivers the same or higher performance than that achieved using a static selection approach.
更多查看译文
关键词
Deep neural networks,Auto-tuning,Implementation selector,Python
AI 理解论文
溯源树
样例
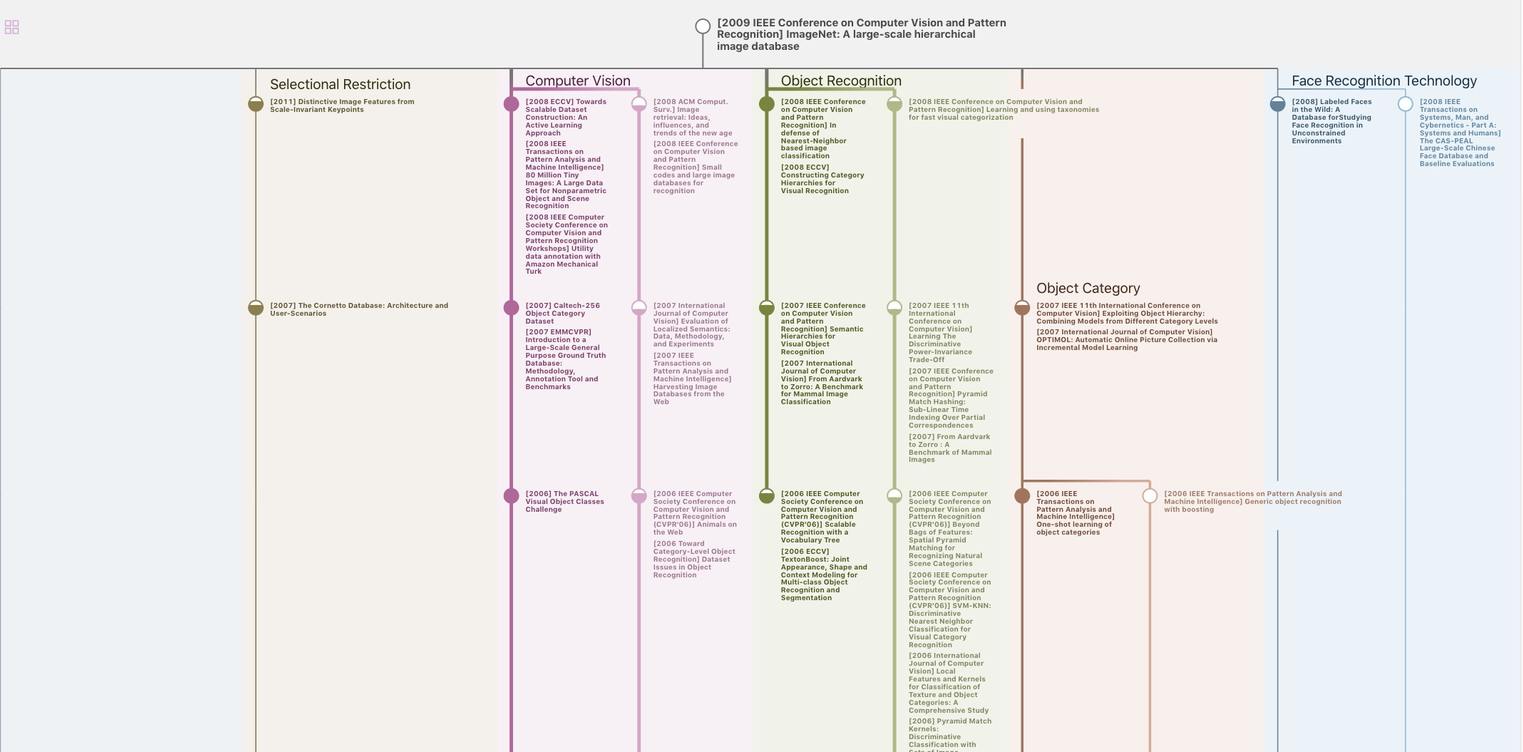
生成溯源树,研究论文发展脉络
Chat Paper
正在生成论文摘要