Correct the distribution by augmenting feature for few-shot learning
2021 3rd International Academic Exchange Conference on Science and Technology Innovation (IAECST)(2021)
摘要
Few-shot classification is a challenge in deep learning, the purpose is to learn a good classifier through the limited samples. Since the number of samples for learning is small, the learned classifier is easily overfitted. In this paper, to address the problem we propose an effective few-shot-learning method. Our contributions are three-fold: 1) the pre-training model on the base class (the sufficient examples) is adaptively generated by the manifold-mixup and self-supervision training way of rotation. 2) we adopt the features of the novel class to fine-tune the last layer of the model. 3) Using the mean and covariance of the base class features, we design a novel feature augmentation method to estimate the feature distribution of the novel class to further improve the performance of our few-shot-learning model. Experiments on benchmark datasets show that our method is effective, and it can achieve the better results.
更多查看译文
关键词
Few-shot classification,self-supervision,deep learning,feature augmentation
AI 理解论文
溯源树
样例
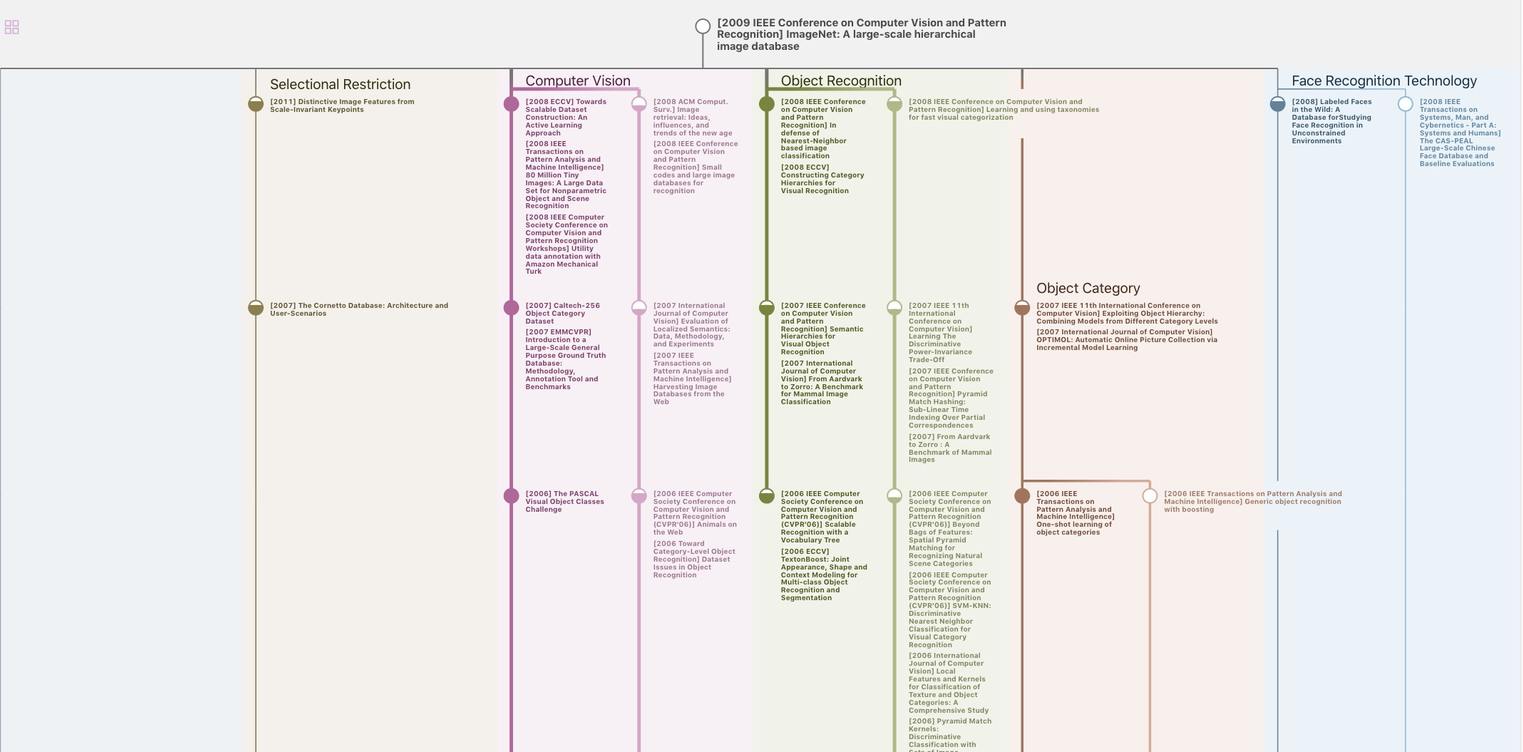
生成溯源树,研究论文发展脉络
Chat Paper
正在生成论文摘要