Cloud Workload Turning Points Prediction via Cloud Feature-Enhanced Deep Learning
IEEE Transactions on Cloud Computing(2023)
摘要
Cloud workload turning point is either a local peak point standing for workload pressure or a local valley point standing for resource waste. Predicting such critical points is important to give warnings to system managers to take precautionary measures aimed at achieving high resource utilization, quality of service ( QoS), and profit of the investment. Existing researches mainly focus more on the workload's future point value prediction only, whereas trend-based turning point prediction is not considered. Moreover, one of the most critical challenges during the prediction is the fact that traditional trend prediction methods which succeed in financial and industrial areas, etc., have a weak ability to represent the cloud features, which means that they cannot describe the highly-variable cloud workloads time series. This article introduces a novel cloud workload turning point prediction approach based on cloud feature-enhanced deep learning. First, we establish a turning point prediction model of cloud server workload considering cloud workload features. Then, a cloud feature-enhanced deep learning model is designed for workload turning point prediction. Experiments on the most famous Google cluster demonstrate the effectiveness of our model compared with state- of-the-art models. To the best of our knowledge, this article is the first systematic research on turning point-based trend prediction of cloud workload time series by cloud feature-enhanced deep learning.
更多查看译文
关键词
Cloud computing,turning point prediction,deep learning,cloud feature-enhanced,time series analysis
AI 理解论文
溯源树
样例
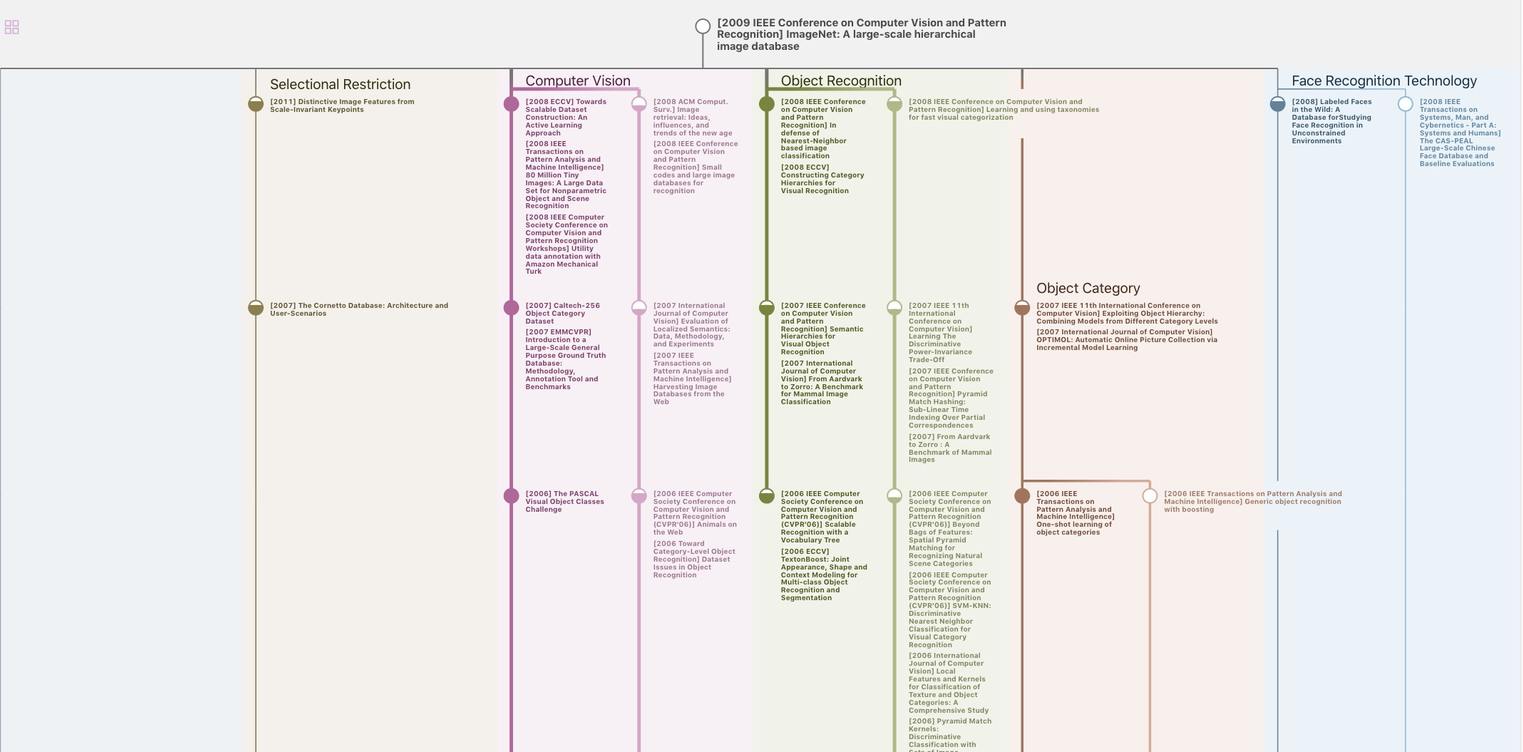
生成溯源树,研究论文发展脉络
Chat Paper
正在生成论文摘要