DHWP: Learning High-Quality Short Hash Codes Via Weight Pruning
ICASSP 2022 - 2022 IEEE International Conference on Acoustics, Speech and Signal Processing (ICASSP)(2022)
摘要
Hashing is widely used in large-scale image retrieval because of its efficiency in storage and computation. Although longer hash codes can lead to higher search accuracy, the retrieval cost increases linearly with the increase of the number of hash bits. Most deep hashing methods suffer from the problem of trivial solutions and usually result in highly correlated redundant hash bits in practice, which limits the performance. To obtain short hash codes with high quality for fast and accurate image retrieval, we propose a novel framework named Deep Hashing via Weight Pruning (DHWP). DHWP first trains the model with relatively long hash codes. Then it obtains shorter codes gradually by weight pruning based on four different criteria. The framework of DHWP can be applied to most deep supervised hashing models, which helps remove those redundant hash bits while retaining the representation ability of long hash codes. Extensive experimental results on two widely used benchmark datasets show that DHWP outperforms the existing state-of-the-art methods, especially for short hash codes.
更多查看译文
关键词
Deep hashing,Image retrieval,Supervised hashing
AI 理解论文
溯源树
样例
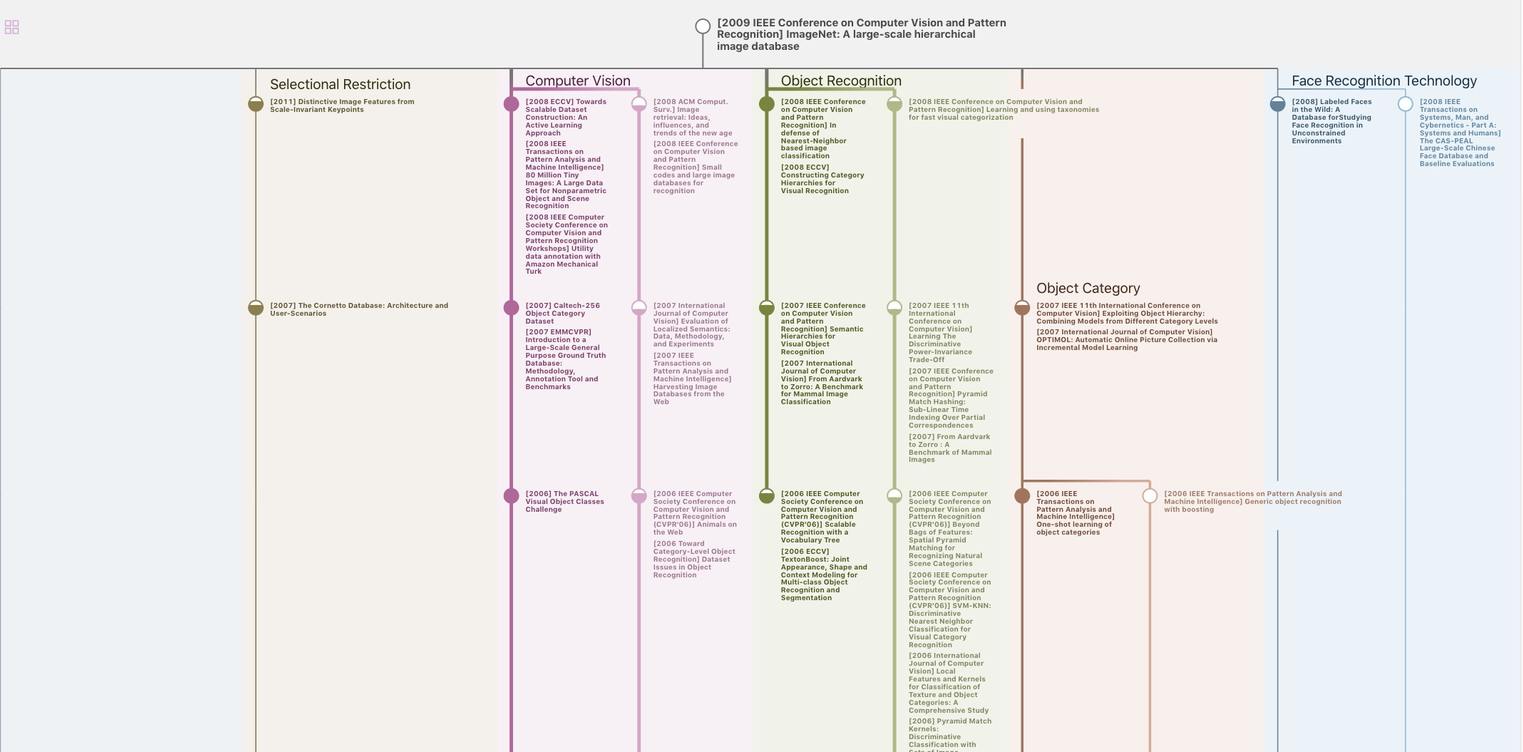
生成溯源树,研究论文发展脉络
Chat Paper
正在生成论文摘要