An adaptive subspace data-driven method for nonlinear dynamic systems
JOURNAL OF THE FRANKLIN INSTITUTE-ENGINEERING AND APPLIED MATHEMATICS(2023)
摘要
Quality-related data-driven fault detection methods have been universally recognized by researchers in industry and academia. However, the detection performance of the common data-driven method will degrade if it cannot keep up with the dynamic shifts of the production process. In this paper, an adaptive kernel principal component regression (AKPCR) method is thus proposed to process the nonlinear feature and the time-varying property in the system. The approximate Hilbert space approach is provided to update the projection matrix based on the innovation information from the new data for the nonlinear high-dimensional feature space. Besides, the recursive post-decomposition technique is created from the updated projection matrix to better monitor the quality state. Compared with the fixed threshold, an adaptive one is designed in this study to detect the fault encountered in the time-varying system, which can decrease false alarms in fault detection. Finally, the Tennessee Eastman case (TEC) and the waste water treatment system (WWTS) are leveraged to illustrate the reliability of the proposed approach.(c) 2022 The Franklin Institute. Published by Elsevier Ltd. All rights reserved.
更多查看译文
AI 理解论文
溯源树
样例
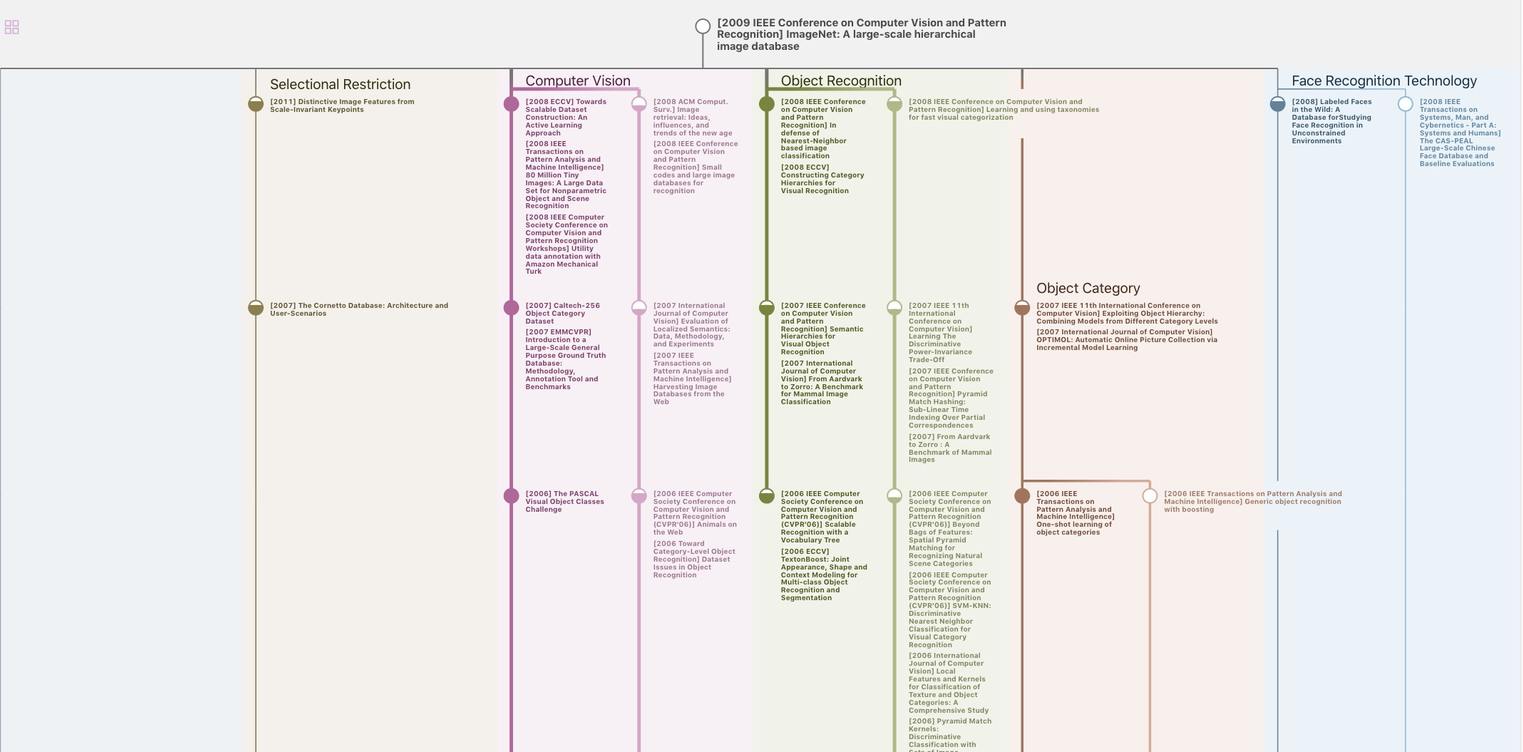
生成溯源树,研究论文发展脉络
Chat Paper
正在生成论文摘要